Weakly-supervised auto-encoder via energy regularization and soft multi-label learning on k labeled samples
Neurocomputing(2024)
摘要
Image classification is a hot topic in computer vision tasks. As a simple unsupervised network model, auto-encoder can learn and apply features to classification. However, due to the lack of prior knowledge of auto-encoder, there are significant limitations in their performance in practical applications. On the contrary, although precise labels can guide supervised learning to learn accurately, it is difficult to obtain strong supervised information on many tasks because of the high cost of data annotation. In this paper, we propose a weakly-supervised auto-encoder via energy regularization and soft multi-label learning on k labeled samples (WSAE), where weakly-supervised learning not only reduces the workload of manual labeling but also introduces supervised information, which improves the performance of auto-encoder to a large extent. Specifically, we first explore a lower bound value of training data that needs to be labeled in weakly supervised learning. Then, we discriminate the visual consistency of k labeled samples compared to unlabeled samples with soft multi-label to efficiently increase the difference of features between classes and reduce the variation of features within classes for more discriminative feature representations and higher classification accuracy. In addition, an energy regularization is introduced to the model to fit the probability distribution of the data so that the smaller the energy, the more concentrated the probability distribution and the more ordered the system. The model with energy regularization can represent the data better, thereby improving the feature extraction ability and increasing the classification accuracy of the network. Extensive experiments are conducted on some publicly available datasets and demonstrate that our method outperforms the state-of-the-art methods.
更多查看译文
关键词
Auto-encoder,unsupervised learning,supervised learning,weakly-supervised learning,soft multi-label,energy regularization
AI 理解论文
溯源树
样例
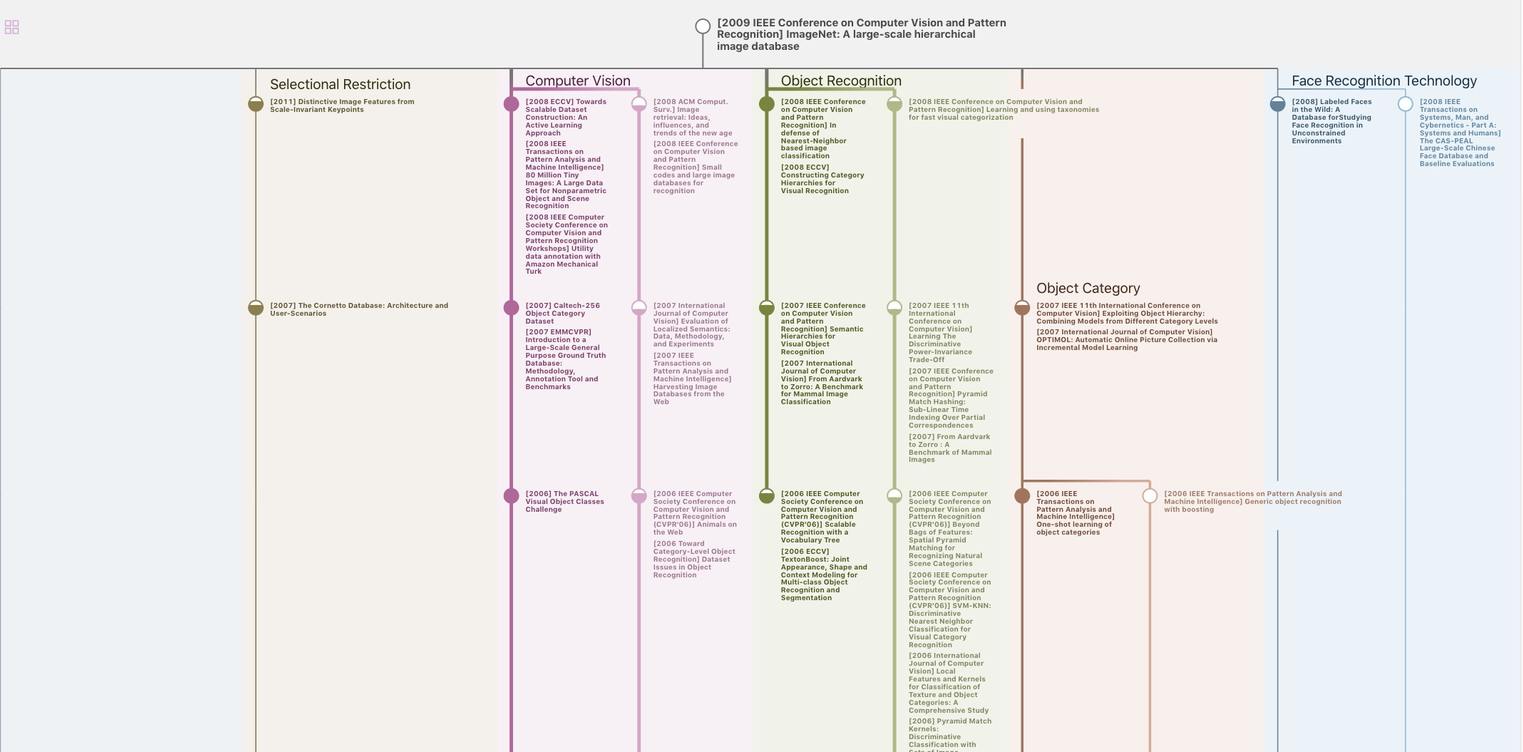
生成溯源树,研究论文发展脉络
Chat Paper
正在生成论文摘要