Mask guided two-stream network for end-to-end few-shot action recognition
Neurocomputing(2024)
摘要
For few-shot video action recognition, it is essential to extract and align features from different videos. However, these operations can be complicated and unreliable due to the complexity of the video scene and the limitations of existing alignment algorithms. To enhance the saliency of the action-related features, we introduce segmentation mask frame sequences as prior information and devise a two-stream feature fusion module to fuse the multimodal features. Furthermore, we propose a self-attention-based temporal alignment module to predict the optimal alignment matrix between the features of samples in the query and support sets. This module avoids solving additional optimization problems in computing the alignment matrix, thus reducing the difficulty of the model for end-to-end learning. Our approach achieves competitive performance on four public datasets. We also experimentally validate the effectiveness of the proposed modules.
更多查看译文
关键词
Few-shot action recognition,Multi-modality fusion,Temporal alignment
AI 理解论文
溯源树
样例
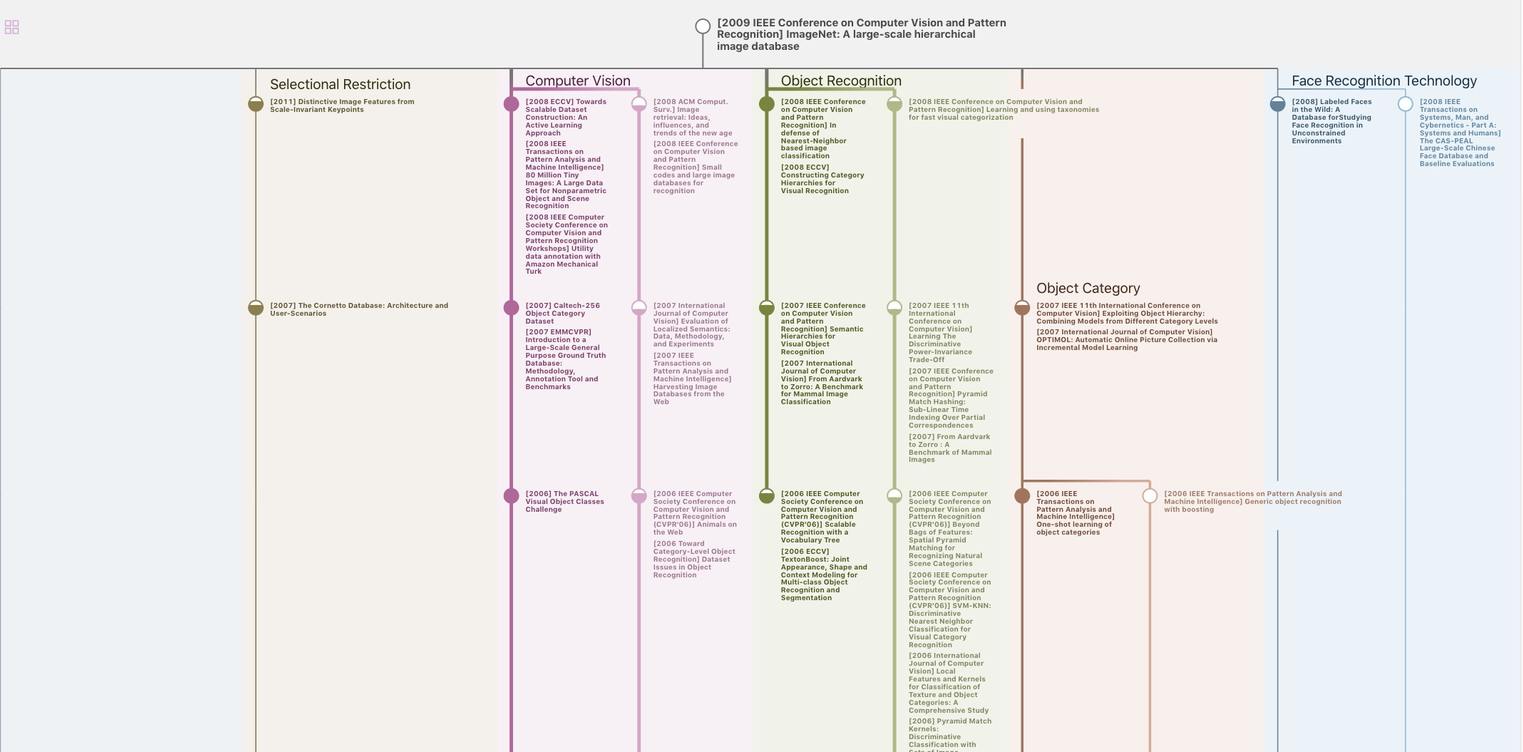
生成溯源树,研究论文发展脉络
Chat Paper
正在生成论文摘要