Malicious detection model with artificial neural network in IoT-based smart farming security
Cluster Computing(2024)
摘要
The Internet of Things (IoT) tunes modern technologies, including wireless sensors and cloud computing, to create a homogeneous and highly effective environment. Therefore, IoT has emerged in various fields of life, such as healthcare, industry, and agriculture. Agriculture is among the primary components of developing nations’ financial states and is vital in maintaining human life. However, the human capacity to reproduce far exceeds the capability of our planet to secure the food required for our lives. Hence, the emergence of IoT in this industry has seen essential advancements to help boost agriculture production and quality. In addition, this emergence exposes the smart agriculture environment to considerable cyber threats. This paper presents a network intrusion detection system (NIDS) to mitigate smart agriculture security vulnerabilities. We developed our framework using radial basis functions neural networks (RBFNN) to detect and classify intrusions in the IoT network. To get our model to perform in its best form, we applied crowd wisdom tree-based machine learning (ML) techniques to select relevant features from the datasets, such as random Forrest (RF), AdaBoost (ADA), extra trees (ET), LightGBM (LGBM), and XGBoost (XGB). We implemented a single-class support vector machine (1-CSVM) to detect and remove outliers. We evaluated our model using NF-Bot-IoT and NF-ToN-IoT datasets. It scored 99.25
更多查看译文
关键词
Smart farming security,Intrusion detection,Artificial neural network,Feature selection
AI 理解论文
溯源树
样例
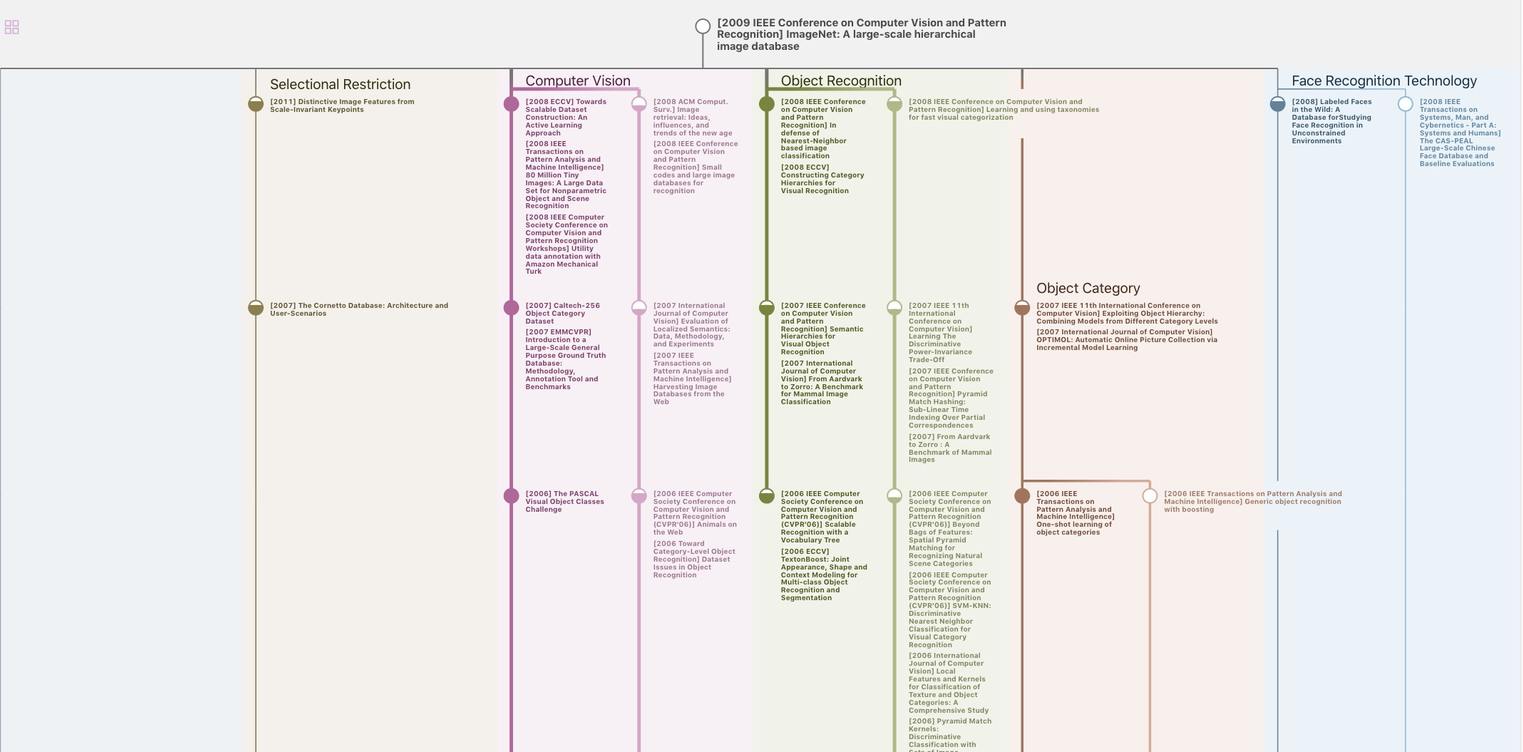
生成溯源树,研究论文发展脉络
Chat Paper
正在生成论文摘要