(Quasi-)Monte Carlo Improtance Sampling with QMCPY
Illinois Tech Undergraduate Research Journal(2023)
摘要
(Quasi-)Monte Carlo, (Q)MC, methods are a class of powerful numerical integration algorithms that have been proven to scale well to high dimensions. Various techniques exist to decrease the computational cost of (Q)MC methods. This article focuses on importance sampling, a technique that performs variable transformations to make the integral easier for (Q)MC approximation. The build up to composed importance sampling is paralleled by code from our QMCPy package that implements these concepts.
更多查看译文
AI 理解论文
溯源树
样例
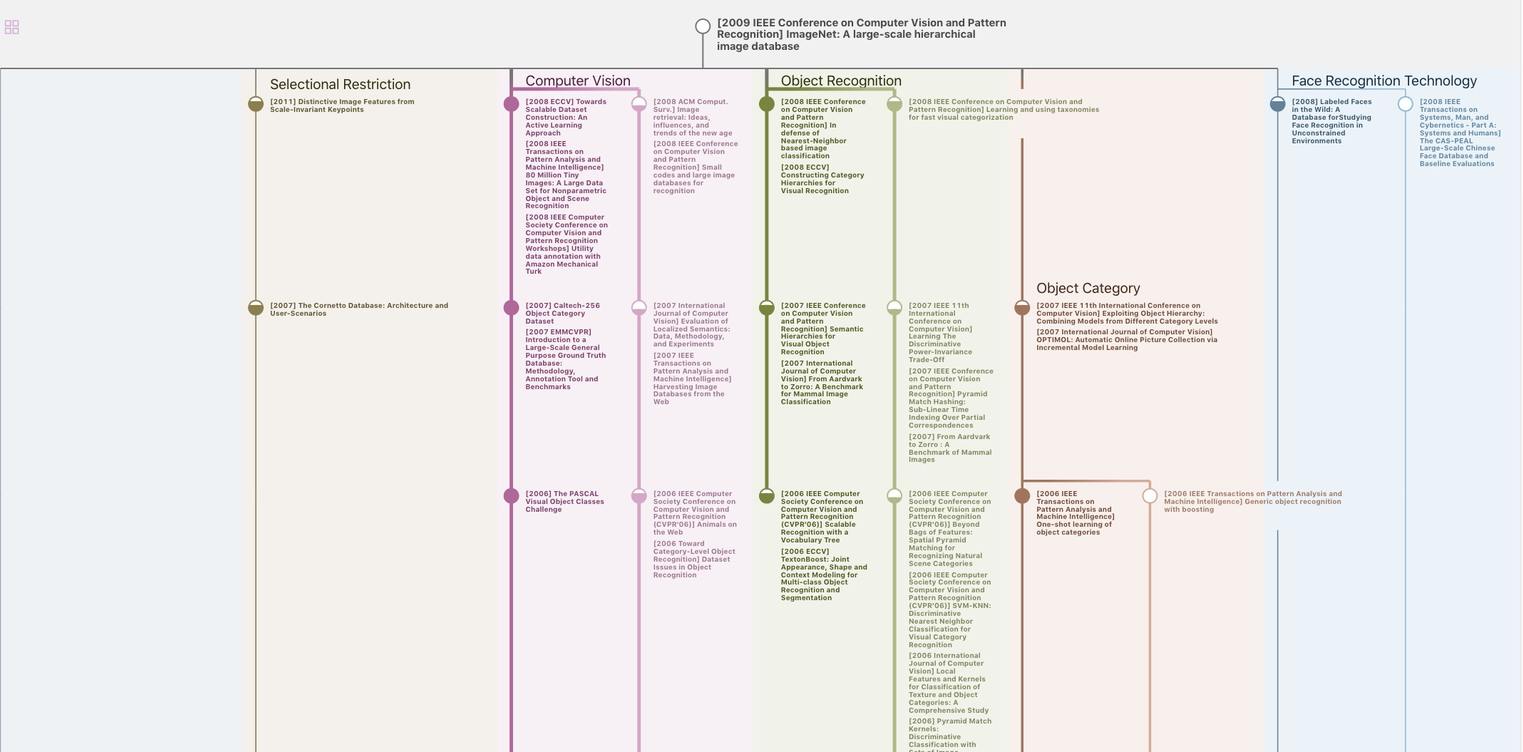
生成溯源树,研究论文发展脉络
Chat Paper
正在生成论文摘要