The success of computational material design for sustainable energy catalysis
CANADIAN JOURNAL OF CHEMISTRY(2024)
摘要
Computational material design (CMD) employs quantum mechanical simulations, density functional theory, and machine learning techniques to correlate electronic structural attributes with physical and chemical properties of materials. Over the last decade, CMD has proven to be critical to the advancement of materials science and a variety of engineering fields. This contribution provides an overview of CMD's success in driving materials discovery for catalysis in the context of sustainable energy applications. Specifically, we discuss how CMD has enabled the development of catalysts for three electrochemical processes that are critical to sustainable energy applications, oxygen reduction reactions, water oxidation reactions, and CO2 reduction reactions. We illustrate how CMD provides a powerful and efficient method for understanding underlying reaction mechanisms as well as predicting and optimizing catalyst properties. Furthermore, we demonstrate how this strategic approach has enabled researchers to effectively navigate the vast chemical space of potential catalysts and rapidly identify novel materials possessing desirable electronic structures and catalytic activity.
更多查看译文
关键词
computational material design,density functional theory calculations,machine learning,catalyst material design
AI 理解论文
溯源树
样例
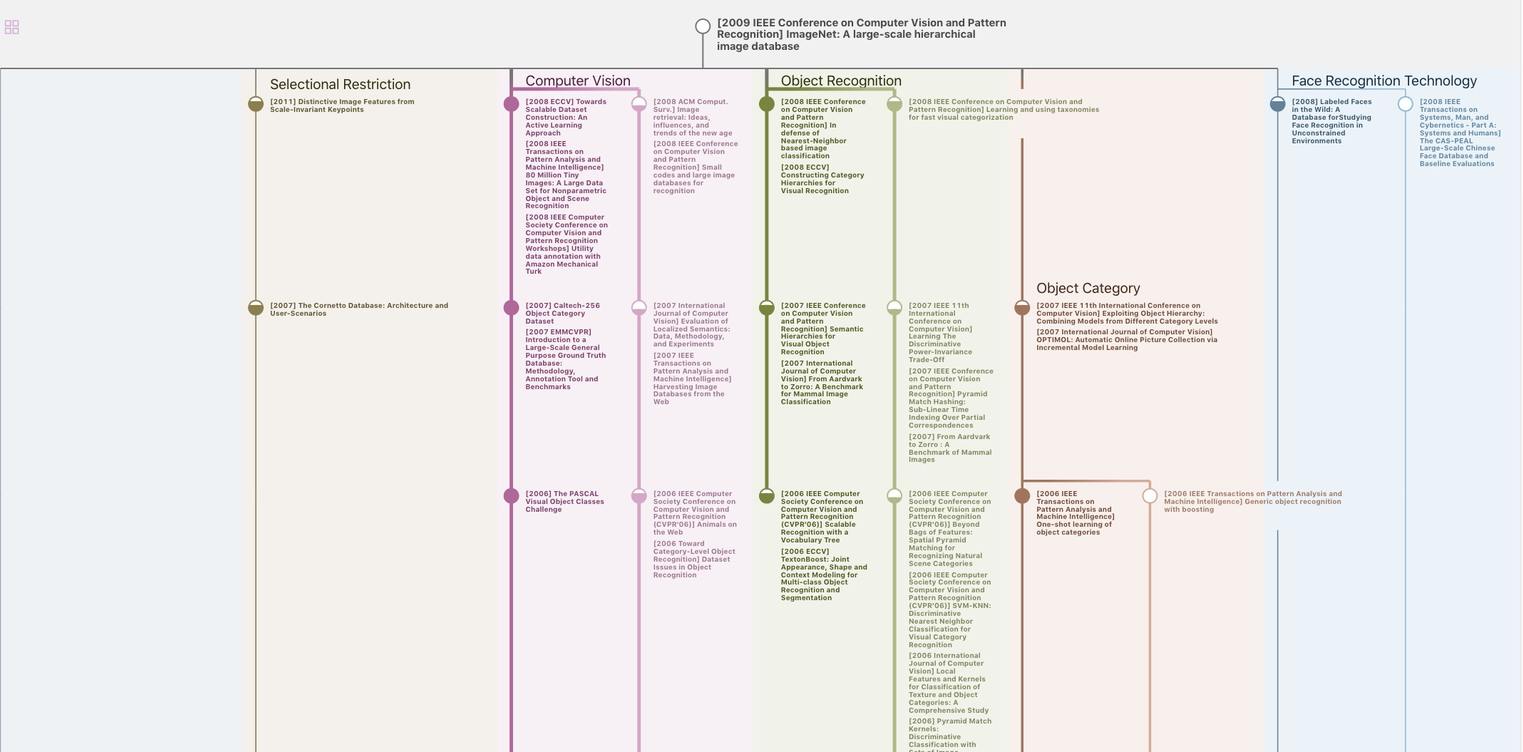
生成溯源树,研究论文发展脉络
Chat Paper
正在生成论文摘要