Abstract 909: Enhancing genomic analysis in cancer diagnostics: A machine learning approach for removing artifacts in FFPE specimens
Cancer Research(2024)
摘要
Abstract Formalin-Fixed Paraffin-Embedded (FFPE) specimens, widely utilized in clinical cancer diagnostics, present significant challenges by introducing artifacts into genomic data. This study aimed to profile these FFPE-induced genomic alterations, with a particular focus on single-nucleotide variants (SNVs), small insertions and deletions (indels), and copy-number variations (CNVs), and to develop computational methods for filtering out such artifacts. Our primary focus was twofold: first, to comprehensively characterize the unique error profile of FFPE specimens observed through whole-genome sequencing (WGS), and second, to construct artifact classifiers and noise filters for SNV/indels and CNVs. We utilized machine learning (ML) and signal-processing techniques on a dataset of FFPE and matched fresh-frozen (FF) samples. The dataset of 52 FFPE-FF pairs were obtained from four different medical institutes and from various cancers including liver, breast, colon, stomach, and lung cancer, with varied FFPE archiving times. We also analyzed additional FFPE-only samples to refine our methods. Our methodology incorporated advanced computational approaches, including stacking ensemble, transfer learning, and wavelet transform, to enhance robustness and accuracy. The method's design was centered around the notion of not only achieving high performance in distinguishing true signals from FFPE-induced artifacts but also addressing the real-world challenges posed by the varying quality and conditions of clinical FFPE samples. In the analysis, we found peculiar patterns of FFPE-specific error profile, including well-known cytosine deamination and novel mutational signatures. The classifier, building on our findings, effectively differentiated true genomic variants from FFPE-induced artifacts for both SNVs and indels, demonstrating a sensitivity of 0.97, specificity of 0.87, and an F1 score of 0.94 for SNVs. For indels, it achieved a sensitivity of 0.91, specificity of 0.91, and an F1 score of 0.91. The CNV filter notably enhanced the signal-to-noise ratio (SNR) of CNV depth profiles, increasing it from 13dB to 17.5dB on average. Furthermore, we conducted evaluations on two critical measures in cancer and clinical genomics: homologous recombination deficiency (HRD) and tumor mutational burden (TMB), achieving post-filtering concordance rates of 0.99 for HRD—correctly identifying all 8 HRD-positive patients in our dataset—and 0.96 for SNV-based TMB and 0.87 for indel-based TMB. Additionally, a post hoc procedure for sensitive detection of cancer driver mutations resulted in concordance rates of 0.94 for SNVs, 0.91 for indels, and 0.95 for oncogene amplification. Taken together, our study advances FFPE WGS analysis in cancer diagnostics by effectively filtering artifacts and addressing challenges with older, degraded samples, enhancing clinical applicability. Citation Format: Joonoh Lim, Seongyeol Park, Won-Chul Lee, Ryul Kim, Sangmoon Lee, Jeong Seok Lee, Brian Baek-Lok Oh, Young Seok Ju. Enhancing genomic analysis in cancer diagnostics: A machine learning approach for removing artifacts in FFPE specimens [abstract]. In: Proceedings of the American Association for Cancer Research Annual Meeting 2024; Part 1 (Regular Abstracts); 2024 Apr 5-10; San Diego, CA. Philadelphia (PA): AACR; Cancer Res 2024;84(6_Suppl):Abstract nr 909.
更多查看译文
AI 理解论文
溯源树
样例
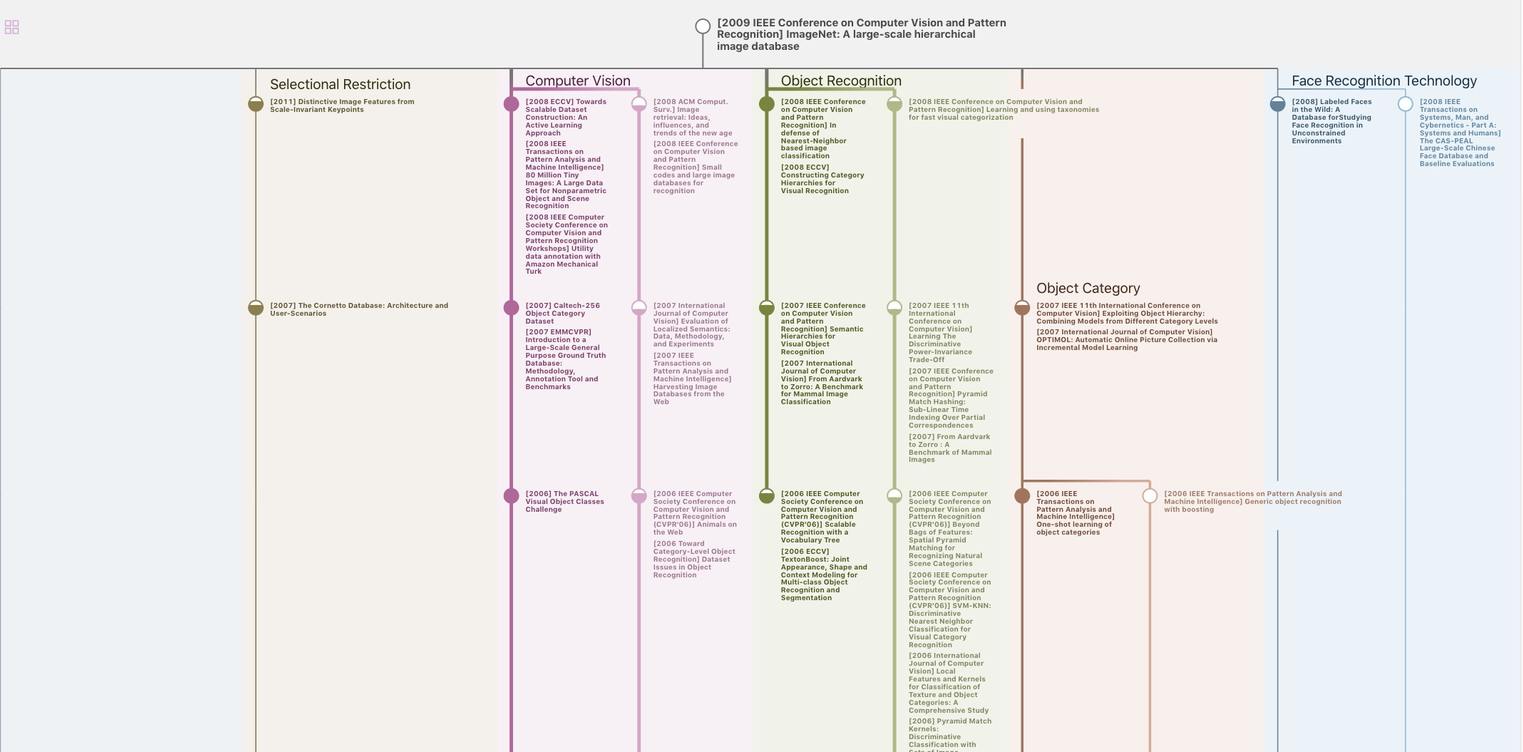
生成溯源树,研究论文发展脉络
Chat Paper
正在生成论文摘要