Optimal Routing Protocol in LPWAN Using SWC: A Novel Reinforcement Learning Framework
IEEE Sensors Journal(2024)
摘要
Low Power Wide Area Network (LPWAN) has emerged as a dominating communication technology that offers low-power and wide coverage for the Internet of Things (IoT) applications. However, the direct data transmission approach has a limited network lifetime. Even multi-hop data transmission experiences many difficulties including high data latency, poor bandwidth utilization, and reduced data throughput. To overcome these challenges, in this paper, a recent breakthrough in social networks known as Small-World Characteristics (SWC) is incorporated into LPWANs. In particular, in this work, Small-World LPWANs (SW-LPWANs) are developed by using the Reinforcement Learning (RL) technique and using different node centrality measures like degree, betweenness, and closeness centrality. Further, the performance of the developed SW-LPWANs is evaluated in terms of energy-efficiency (alive/dead devices, and network residual energy) and Quality-of-Service (average data latency, data throughput, and bandwidth utilization), and are compared with that of conventional multi-hop LPWAN. Finally, to validate the simulation results, similar analyses are performed on the real-field LPWAN testbed. The obtained simulation results confirm that SW-LPWAN developed by the RL method performs better than other techniques, with 11% more alive devices, 5.5% higher residual energy, 2.4% improved data throughput, and 14% efficient bandwidth utilization compared to the next best method. A similar trend is observed with real-field LPWAN testbed data also.
更多查看译文
关键词
Internet of Things (IoT),Low Power Wide Area Network (LPWAN),Small-World Characteristics (SWC),Reinforcement Learning,Energy-efficiency,Quality-of-Service (QoS)
AI 理解论文
溯源树
样例
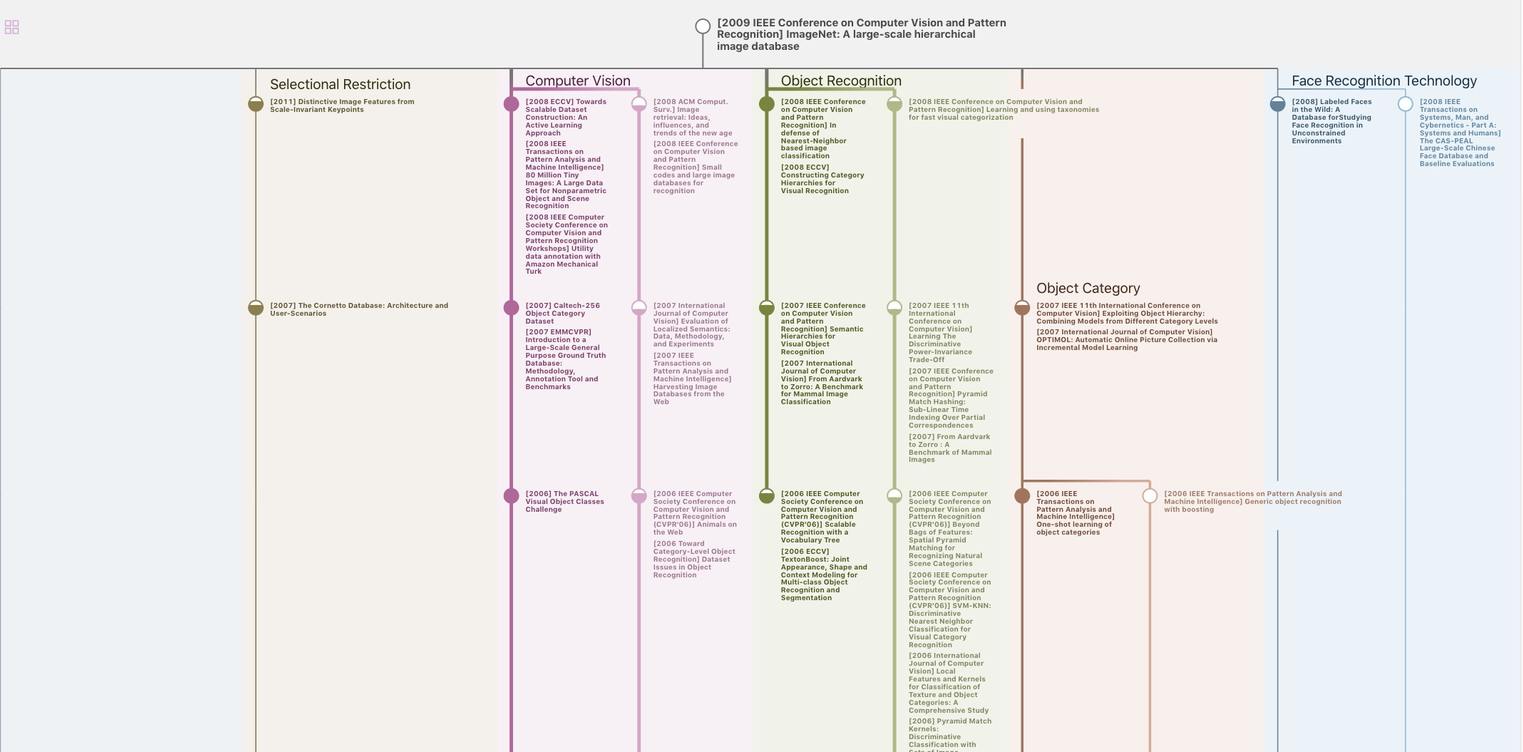
生成溯源树,研究论文发展脉络
Chat Paper
正在生成论文摘要