Shining Light on Electrical Energy Burden: Affordability and Equity in Rate Design
2024 IEEE TEXAS POWER AND ENERGY CONFERENCE, TPEC(2024)
Key words
electricity tariffs,energy transition,energy in-security,energy equity,energy burden
AI Read Science
Must-Reading Tree
Example
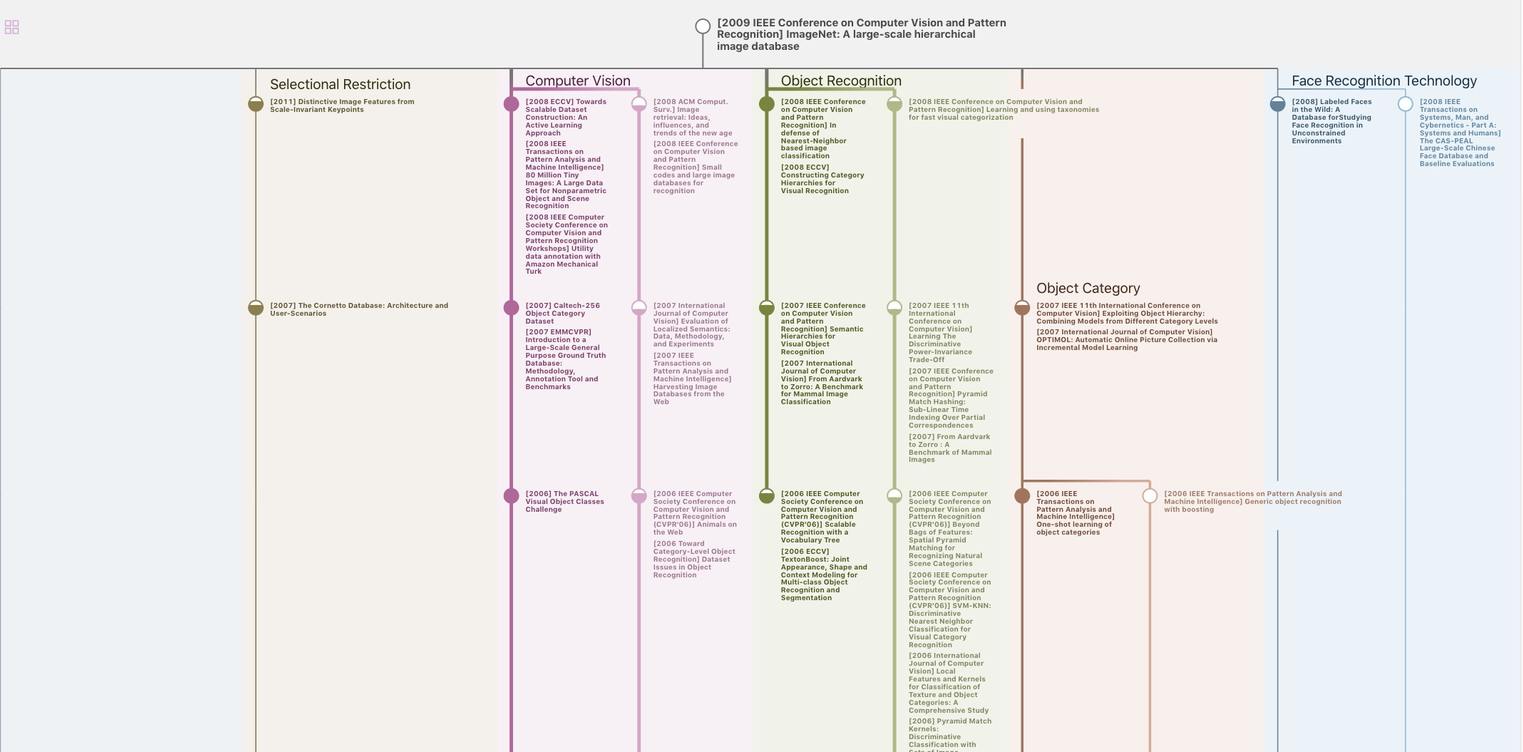
Generate MRT to find the research sequence of this paper
Chat Paper
Summary is being generated by the instructions you defined