Abstract 3529: Leveraging deep learning for fully automated analysis of pre-clinical mouse positron emission tomography
Cancer Research(2024)
摘要
Abstract Turning early-cancer diagnosis into actionable treatment often relies on highly accurate and sensitive imaging techniques to guide surgical intervention or to monitor therapeutic efficacy. Earli is developing a highly sensitive, orthogonal approach that uses genetic constructs to usurp dysregulated cancer pathways to force the tumor to produce a PET reporter gene, enabling the use of radiotracers amenable to positron emission tomography/computed tomography (PET/CT) to guide precision imaging. However, analysis of PET/CT images using standard manual and semi-automated methods is challenging resulting in reduced experimental throughput and user variability, highlighting the need for a fully automated PET/CT analysis pipeline using Deep Learning (DL). To increase pre-clinical throughput and reduce inter-user variability, we developed an automated DL processing pipeline in Python to perform three major tasks: separation of multi-mouse bed data for PET uptake quantification by mouse, segmentation of tumors and background organs, and quantification of PET signal for PK/PD analysis. Preclinical PET/CT data was acquired using a 4 animal, multi-mouse bed and separated into individual mice by co-registering the reconstructed CT image against a multi-mouse bed reference mask using a rigid 3D Euler transformation algorithm and cropping at fixed indices. Following mouse separation, a 3D nnUNet architecture is used to perform semantic segmentation of regions of interest (ROI) on CT for bi-hemispheric subcutaneous tumors, lungs, liver, kidneys, spleen, and the bladder. nnUNet was trained on PET/CT of 311 mice consisting of a mixture of naïve and tumor-bearing animals subcutaneously implanted with H1299 cells using a five-fold cross-validation strategy with 1000 epochs per fold. Model performance was evaluated by comparing the network prediction to the reference manually annotated masks using the Dice coefficient. PET uptake is reported as percent injected dose per milliliter (%ID/mL) or standardized uptake value (SUV). Segmentation performance results for the hold-out set identified mean Dice scores greater than 0.80 for all ROIs, reflecting the similarity between model predictions and human annotations. The automated pipeline reduced the analysis time from 5.5-6 hours per mouse when using traditional manual/semi-automatic approaches to approximately 15 minutes. In summary, we developed a fully automated DL-based PET/CT quantification pipeline for pre-clinical mouse studies that provides accurate ROI segmentation and PET uptake quantification, significantly increasing experimental throughput and reducing inter-user variability. Further improvements to model inference include loss functions weighted for poor performing ROIs and addition of lung nodule segmentation for eventual translation to humans as an accompaniment to Earli’s theranostic programs. Citation Format: Hung-Yu Henry Lee, Mohammed Goryawala, Tim Sproul, Maggie Louie, David Suhy. Leveraging deep learning for fully automated analysis of pre-clinical mouse positron emission tomography [abstract]. In: Proceedings of the American Association for Cancer Research Annual Meeting 2024; Part 1 (Regular Abstracts); 2024 Apr 5-10; San Diego, CA. Philadelphia (PA): AACR; Cancer Res 2024;84(6_Suppl):Abstract nr 3529.
更多查看译文
AI 理解论文
溯源树
样例
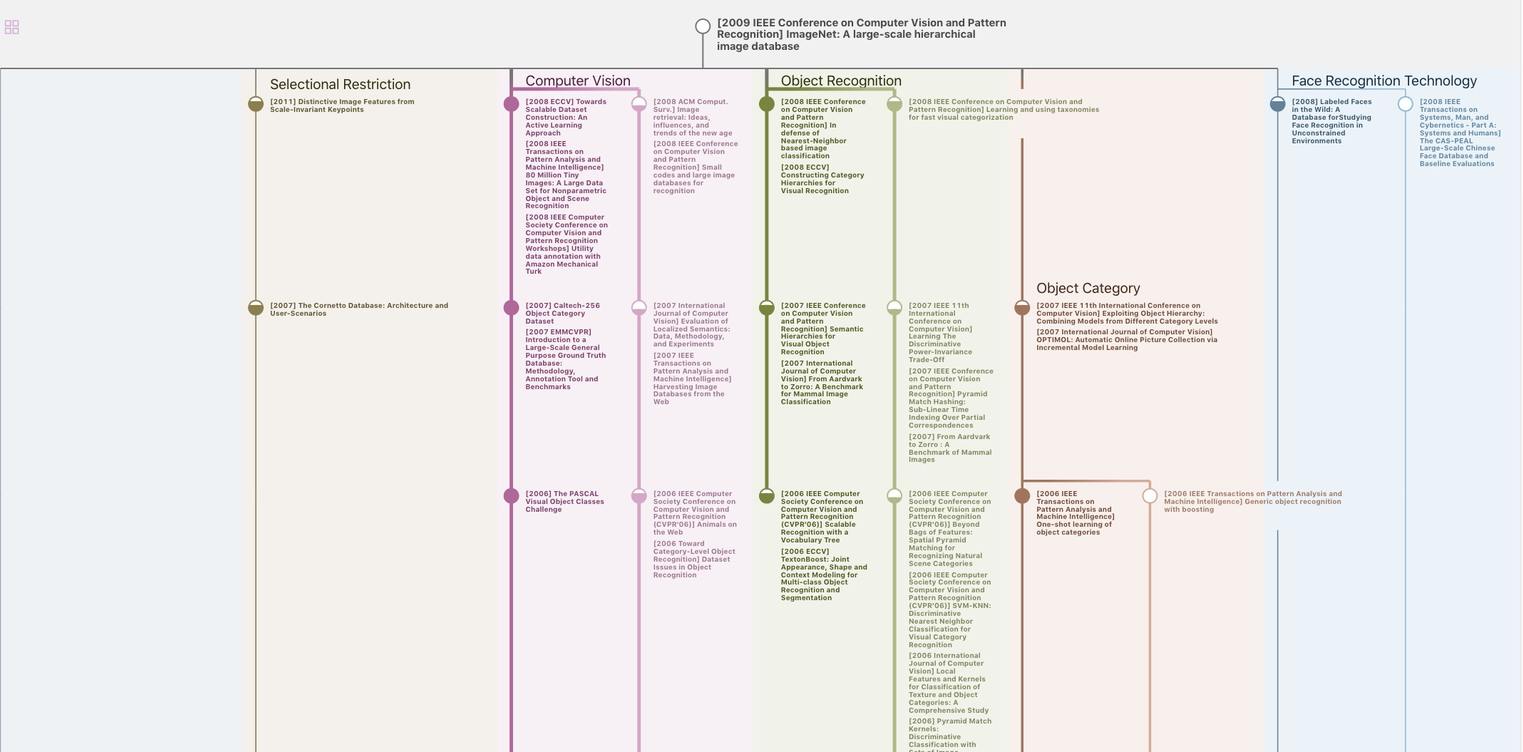
生成溯源树,研究论文发展脉络
Chat Paper
正在生成论文摘要