Abstract 6181: Digital pathology workflow: Building a bridge for translational biomarker development in humanized mice
Cancer Research(2024)
摘要
Abstract The introduction of immune checkpoint inhibitors (ICIs) directed against programmed death protein 1 (PD-1), as well as cytotoxic T lymphocyte antigen 4 (CTLA-4), has revolutionized the treatment of non-small cell lung cancer (NSCLC) in the last decade. These therapies have shown significant success in a subset of NSCLC patients, leading to durable response and improved overall survival. However, clear predictive biomarkers for response are still yet to be defined. Tumor-infiltrating lymphocytes (TILs) as one important part of the tumor microenvironment (TME) have proven to be predictive biomarkers in other tumor types such as melanoma, whereas not fully understood in NSCLC. The assessment of TILs typically involves techniques such as flow cytometry (FC) or immunohistochemistry (IHC). While the former has the advantage of being quantitative and relatively easy to standardize it lacks translational value as most clinical samples will be examined using IHC. The current study aims to compare both methods at terminal end point and for treatment outcome, using a panel of 14 NSCLC PDX models, implanted in humanized NSG mice and treated with anti-CTLA4, anti-PD1 and the combination thereof. Based on FC analyses of the untreated tumor tissue the PDX models were stratified into seven hot (>5% TILs) and seven cold (<5% TILs) tumors. The IHC samples were analyzed by digital pathology for TIL infiltration by making use of automated segmentation of the three main tissue classes: tumor, stroma and necrosis. Small number of IHC slides were manually annotated to form a training set, which were then used to train a deep learning model using Visiopharm, a commercial AI platform for image analysis. By quantification of TILs in a spatial context of the tumor tissue, across all models, tumor was the most prominent tissue class. The tissue class distribution as well as the TIL rate (FC or IHC measure) is a model immanent feature. Under treatment, the ratio was stable in case of the cold tumors. In the hot tumors the stroma compartment increased while tumor tissue decreased. Treatment sensitive models displayed an increase of TILs confirmed by FC and IHC. The latter revealed that the TILs specifically increased in the tumor tissue whereas decreased or was stable in the stroma. This observation was significantly more pronounced in cold tumors compared to hot tumors (Oneway ANOVA, p < 0.001). Furthermore, a positive correlation between FC and IHC results on CD45 positive cells in the tumors (p< 0.002) could be confirmed. In this study, we proofed the feasibility of software-based image analysis as translational relevant read-out in preclinical animal experiments. The possibility to reproducibly quantify tissue classes, immune cell markers and including spatial information paves the way towards discovery of novel features predicting response in translational immuno-oncology research. Citation Format: Matti Hakkarainen, Timmo Bragge, Jussi Rytkönen, Eva Oswald, Julia B. Schueler. Digital pathology workflow: Building a bridge for translational biomarker development in humanized mice [abstract]. In: Proceedings of the American Association for Cancer Research Annual Meeting 2024; Part 1 (Regular Abstracts); 2024 Apr 5-10; San Diego, CA. Philadelphia (PA): AACR; Cancer Res 2024;84(6_Suppl):Abstract nr 6181.
更多查看译文
AI 理解论文
溯源树
样例
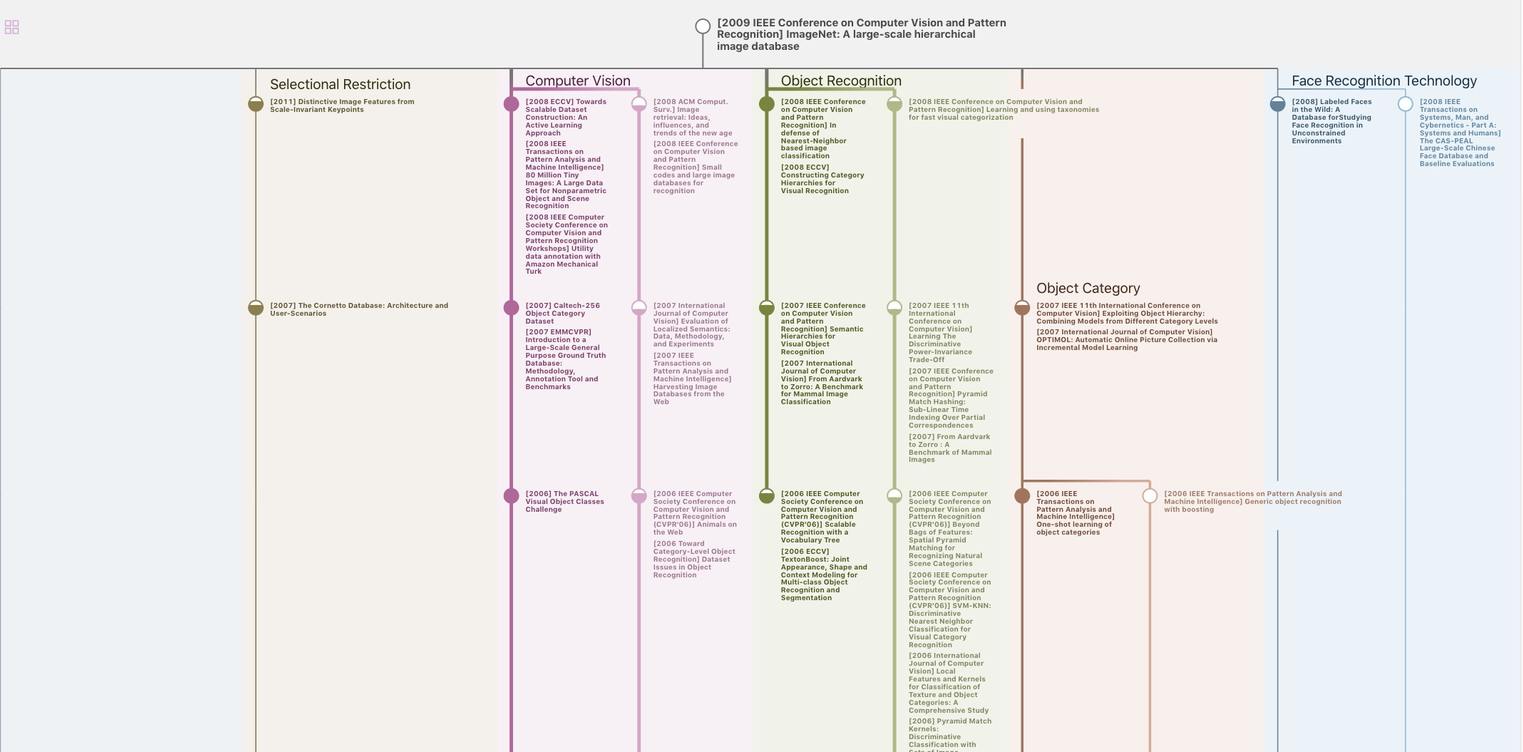
生成溯源树,研究论文发展脉络
Chat Paper
正在生成论文摘要