Robust social recommendation based on contrastive learning and dual-stage graph neural network
Neurocomputing(2024)
摘要
GNN-based social recommendation aims to use social network information to improve recommendation performance of traditional user-item interaction network (U-I network). However, in graph neural network information aggregation, both social networks and U-I networks inevitably have noise, which affects accuracy of recommendation results. To reduce the noise impact of network data, we propose Robust Social Recommendation based on Contrastive Learning and Dual-Stage Graph Neural Network (CLDS). First, considering instability of social networks, we propose the social preference network. It is robust and retains only social friend relationships with common preferences. Based on it and U-I network, we construct a social recommendation pre-training model. Next, we propose self-contrastive learning method. The method initializes multiple social network node representations through Gaussian distribution, pre-training and random disturbance, respectively. Then, it uses contrastive learning on the generated multiple node representations to enhance the robustness of node representation. Finally, CLDS avoids directly capturing potentially user-user and item-item information in U-I networks which is incomplete and untrusted. And instead, it only extracts user-item information to reduce the noise generated by GNN-based U-I network information aggregation. We conduct experiments under the open-source real network dataset. The experimental results show that CLDS outperforms state-of-art methods in social recommendation. The code is available at: https://github.com/Andrewsama/CLDS-master.
更多查看译文
关键词
Robustness,Social recommendation,Self-contrastive learning,Graph neural network,Network data denoising
AI 理解论文
溯源树
样例
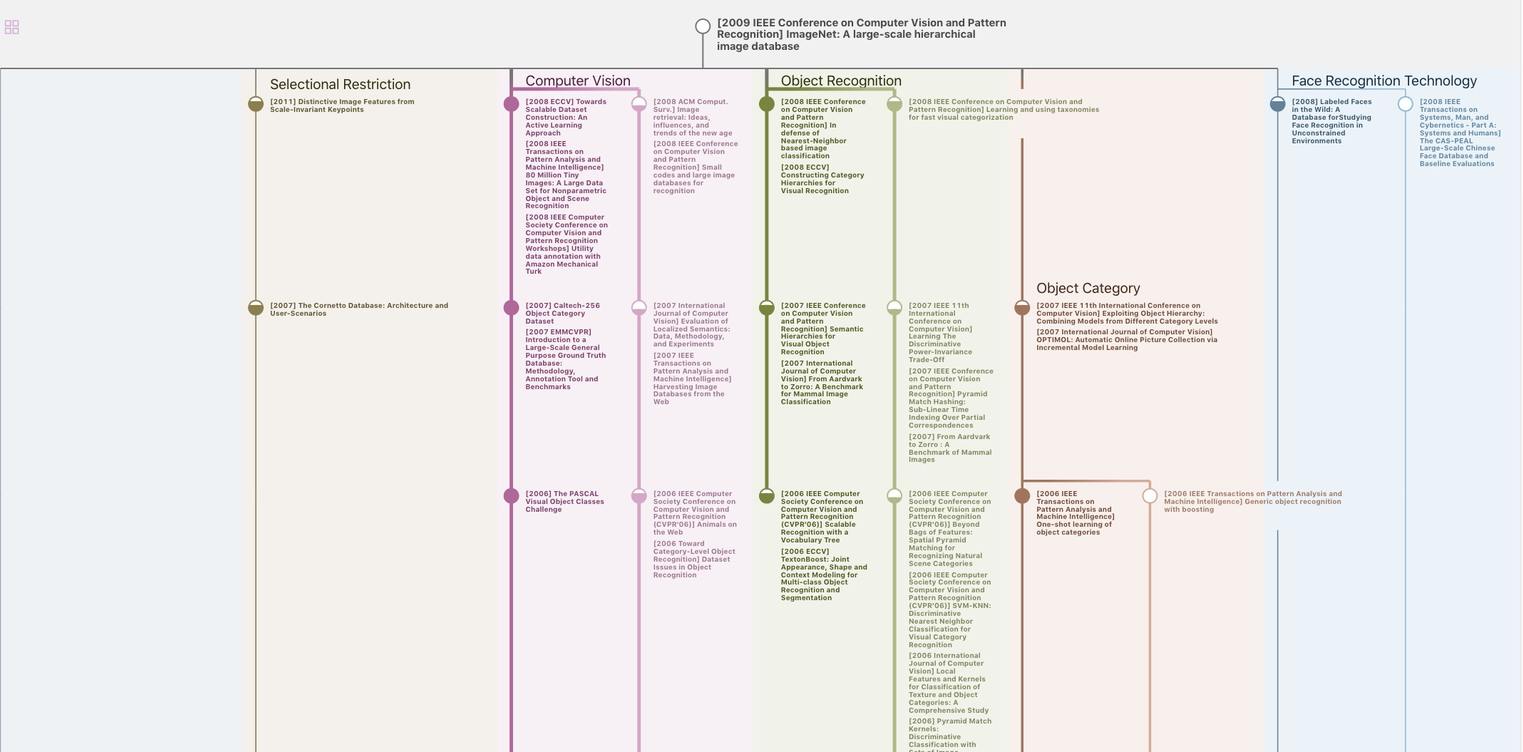
生成溯源树,研究论文发展脉络
Chat Paper
正在生成论文摘要