15 years of Adjoint Algorithmic Differentiation (AAD) in finance
QUANTITATIVE FINANCE(2024)
摘要
Following the seminal 'Smoking Adjoint' paper by Giles and Glasserman [Smoking adjoints: Fast monte carlo greeks. Risk, 2006, 19, 88-92], the development of Adjoint Algorithmic Differentiation (AAD) has revolutionized the way risk is computed in the financial industry. In this paper, we provide a tutorial of this technique, illustrate how it is immediately applicable for Monte Carlo and Partial Differential Equations applications, the two main numerical techniques used for option pricing, and review the most significant literature in quantitative finance of the past fifteen years.
更多查看译文
关键词
Algorithmic differentiation,Monte Carlo simulations,Partial differential equations,Derivatives pricing,Calibration of stochastic models
AI 理解论文
溯源树
样例
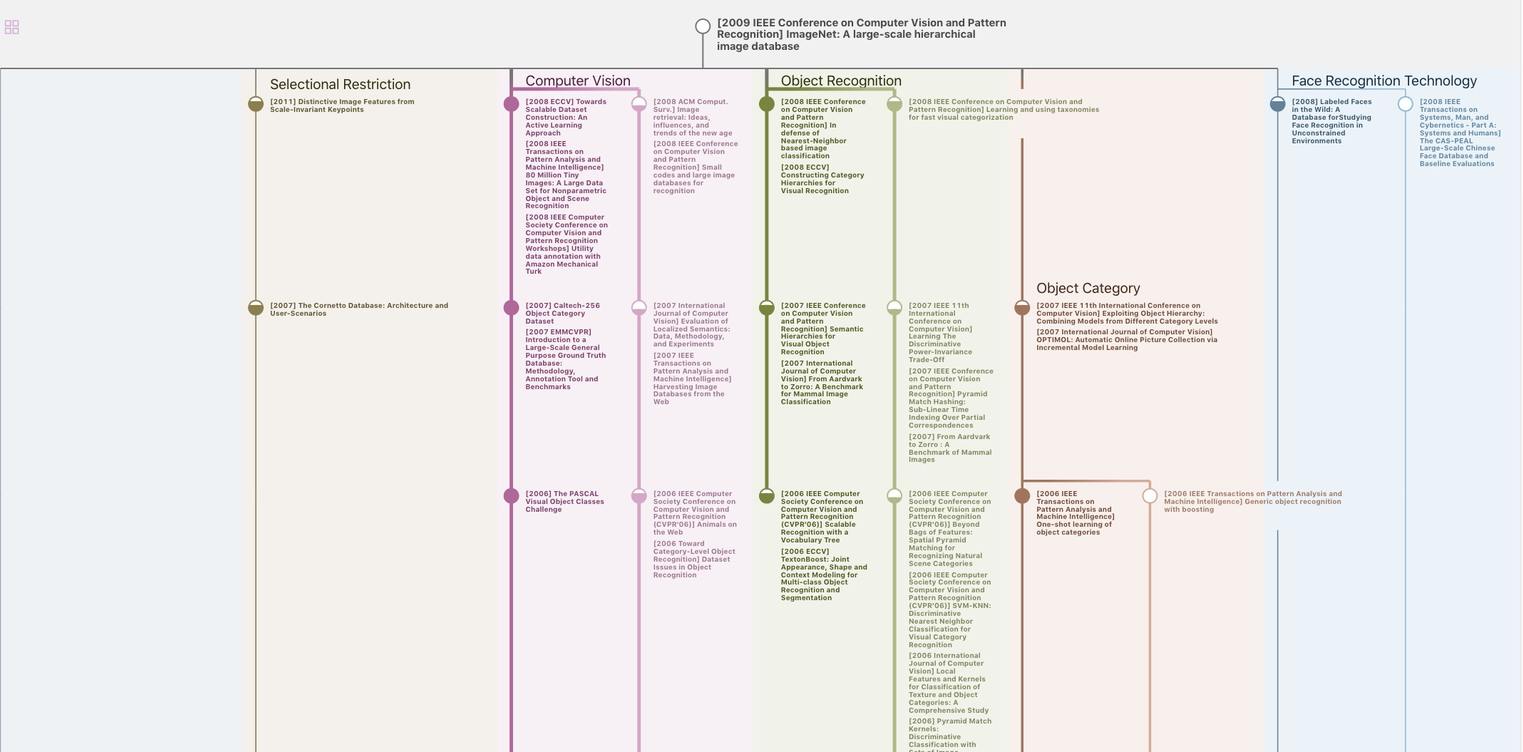
生成溯源树,研究论文发展脉络
Chat Paper
正在生成论文摘要