Abstract 2595: Accelerated and precise tumor segmentation in NSCLC: A comparative analysis of automated ClickSeg and manual annotation for radiomics
Cancer Research(2024)
摘要
Abstract Background: Radiomics models utilizing artificial intelligence are being explored as a potential biomarker in the field of oncology. Radiomics analysis requires segmentations of radiographic imaging. However, manual segmentation is a labor-intensive process that is time consuming, and acts as a major rate-limiting step. Thus the development of automated segmentation tools presents an opportunity for innovation in regards to efficiency and precision. Our study aims to explore the feasibility of autosegmentation in comparison with manual segmentation. Methods: A cohort of 105 stage III-IV non-small cell lung cancer patients receiving immunotherapy was examined, with a total of 168 lesions. We conducted a comprehensive comparative analysis of manual versus automated segmentation in time efficiency and segmentation quality, utilizing CT scans from the patients. Manual segmentation was performed by three physicians annotating in consensus using LIFEx software v7.3.0 (IMIV/CEA, Orsay, France). For a subset of randomly selected patients, annotation time was recorded as a reference point for the automated segmentation. In addition, automated segmentation was performed on all 168 lesions using ClickSeg from PictureHealth, a click-based interactive segmentation algorithm optimized for NSCLC lesions. Segmentation quality was evaluated using the Dice similarity coefficient to measure the concordance between ClickSeg outputs and consensus expert annotations. Results: Average manual annotation time of the tumor region was 15min 34sec, and ranged from 1min 2sec to 53min 12sec.. Average prediction time for the automated segmentation model was 1.94 seconds, and ranged from 0.71 seconds to 4.39 seconds. The median Dice coefficient, reflecting segmentation accuracy, was 0.72, highlighting the robust performance of the automated method. Conclusion: Our study demonstrates the efficient and accurate nature of automated segmentation supporting its potential integration into routine radiomic analysis procedures, streamlining the assessment of treatment response and ultimately improving patient care. Citation Format: Seyoung Lee, Kai Zhang, Jeeyeon Lee, Peter Haseok Kim, Amogh Hiremath, Salie Lee, Monica Yadav, Maria J. Chuchuca, Taegyu Um, Myungwoo Nam, Liam Il-Young Chung, Hye Sung Kim, Jisang Yu, Trie Arni Djunadi, Leeseul Kim, Youjin Oh, Sungmi Yoon, Zunairah Shah, Yuchan Kim, Ilene Hong, Grace Kang, Jessica Jang, Amy Cho, Soowon Lee, Cecilia Nam, Timothy Hong, Yuri S. Velichko, Anant Madabhushi, Nathaniel Braman, Young Kwang Chae. Accelerated and precise tumor segmentation in NSCLC: A comparative analysis of automated ClickSeg and manual annotation for radiomics [abstract]. In: Proceedings of the American Association for Cancer Research Annual Meeting 2024; Part 1 (Regular Abstracts); 2024 Apr 5-10; San Diego, CA. Philadelphia (PA): AACR; Cancer Res 2024;84(6_Suppl):Abstract nr 2595.
更多查看译文
AI 理解论文
溯源树
样例
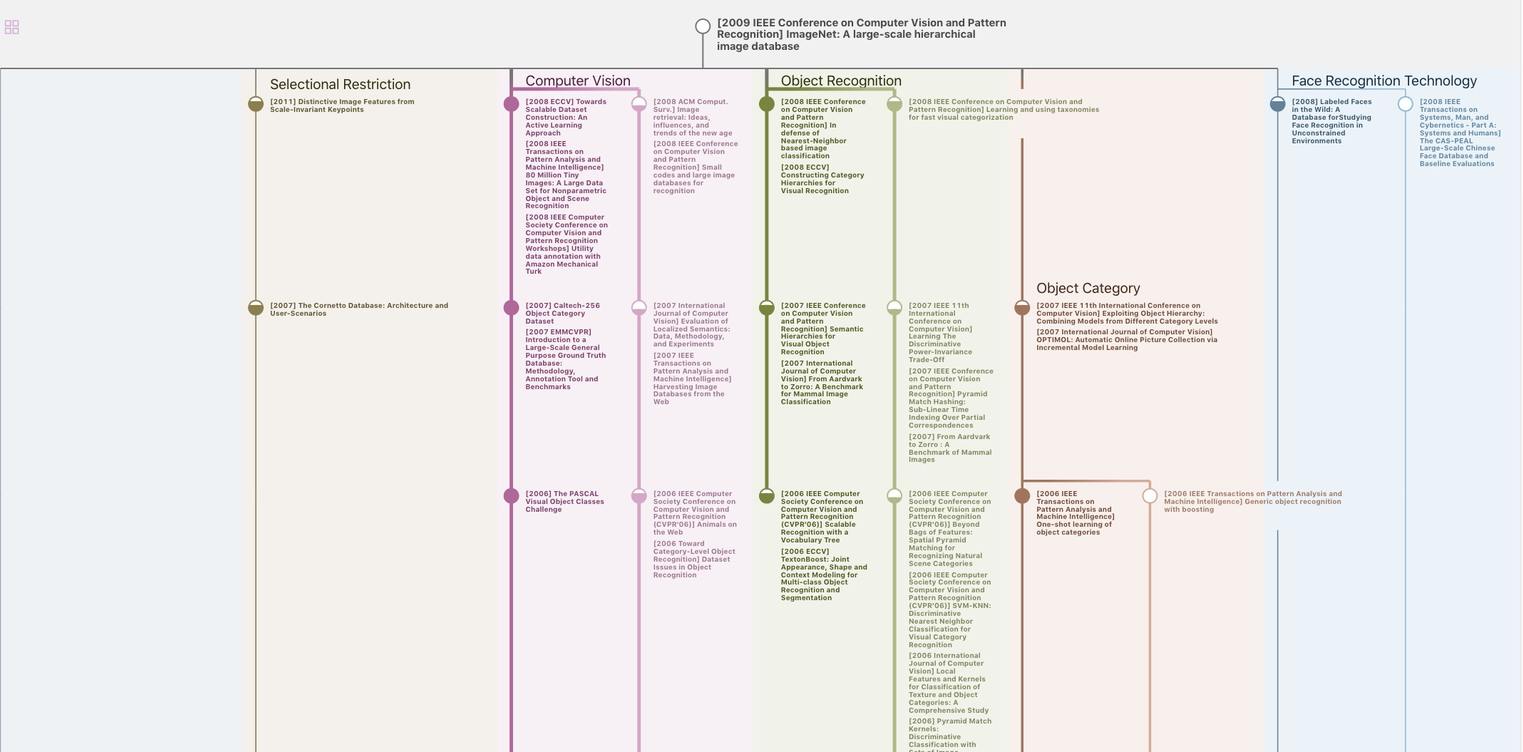
生成溯源树,研究论文发展脉络
Chat Paper
正在生成论文摘要