Abstract 5887: Identifying New Immunotherapy Targets Using Machine Learning and Ex Vivo Validation
Cancer Research(2024)
摘要
Abstract Overview: Immunotherapy has revolutionized cancer treatment. However, existing immune checkpoint inhibitors (CPI) yield low response rates in most cancers, highlighting the need for new therapeutic options. Traditional immune-oncology (IO) target discovery relies on preclinical models, which struggle to recapitulate human tumor complexity, limiting translation potential. Attention is thus directed at harnessing multimodal patient molecular data with modern machine learning (ML) techniques to identify new IO targets which may have higher clinical viability. Approach: We posed IO target discovery as a binary classification task, training ML systems on known candidate drug targets that have progressed to stage I or higher clinical trials. We constructed a rich knowledge graph database to support model development. Graph nodes (n=11,919) comprised genes with edges linking genes involved in n=99,275 protein-protein interactions (PPIs; PMID: 28936969). Genes were labelled with n=6,387 gene-disease associations alongside bulk exome and transcriptome (RNAseq) features from n=1,317 CPI-treated patients (PMID: 33508232). We considered the role of different immune subsets by incorporating cell type-specific PPIs from single cell RNAseq atlases of n=350 samples (PMID: 31786210). To capture how antigen processing affects immunotherapy response, we also examined the immunopeptidome of n=60 patients (PMID: 30556813). Causal data on immune responses to genetic perturbation stemmed from n=7 publicly available CRISPR tumor-T cell co-cultures and n=15,442 SNP-phenotype links. Lastly, we used interpretability analysis to understand drivers of model predictions and elucidate critical genes that influence multiple IO target pathways. Results: Firstly, we developed an ensemble ML approach which achieved test ROC-AUC>0.75. Next, we used a graph-based ML framework which yielded superior performance (test ROC-AUC>0.90). Orthogonal validation confirmed these models can discriminate known targets by trial phase (p<0.001), predict patient response in new CPI trials (p<0.05), and identify genes that rank highly in unseen genome-wide CRISPR screens. Targets from both methods have entered experimental validation in patient-derived explants and organoid-immune co-cultures. Already, we have identified 2 novel targets predicted to relate to macrophage activity. Early data suggest that perturbing them leads to macrophage repolarization. Further validation experiments are ongoing. Conclusions: We will reveal candidate targets, showing that our method is effective at uncovering new IO targets and can identify critical nodes in biological networks that might be attractive hits. In addition, deciphering data types that drive model predictions provides a broader immunobiological understanding of anti-tumor immune responses. Thus, our results endorse using ML and multimodal data for novel IO target discovery. Citation Format: Marcellus Augustine, Nuno Rocha Nene, Krupa Thakkar, Danwen Qian, Evelyn Fitzsimons, Benjamin S. Simpson, Chris Watkins, Charles Swanton, Kevin Litchfield. Identifying new immunotherapy targets using machine learning and ex vivo validation [abstract]. In: Proceedings of the American Association for Cancer Research Annual Meeting 2024; Part 1 (Regular Abstracts); 2024 Apr 5-10; San Diego, CA. Philadelphia (PA): AACR; Cancer Res 2024;84(6_Suppl):Abstract nr 5887.
更多查看译文
AI 理解论文
溯源树
样例
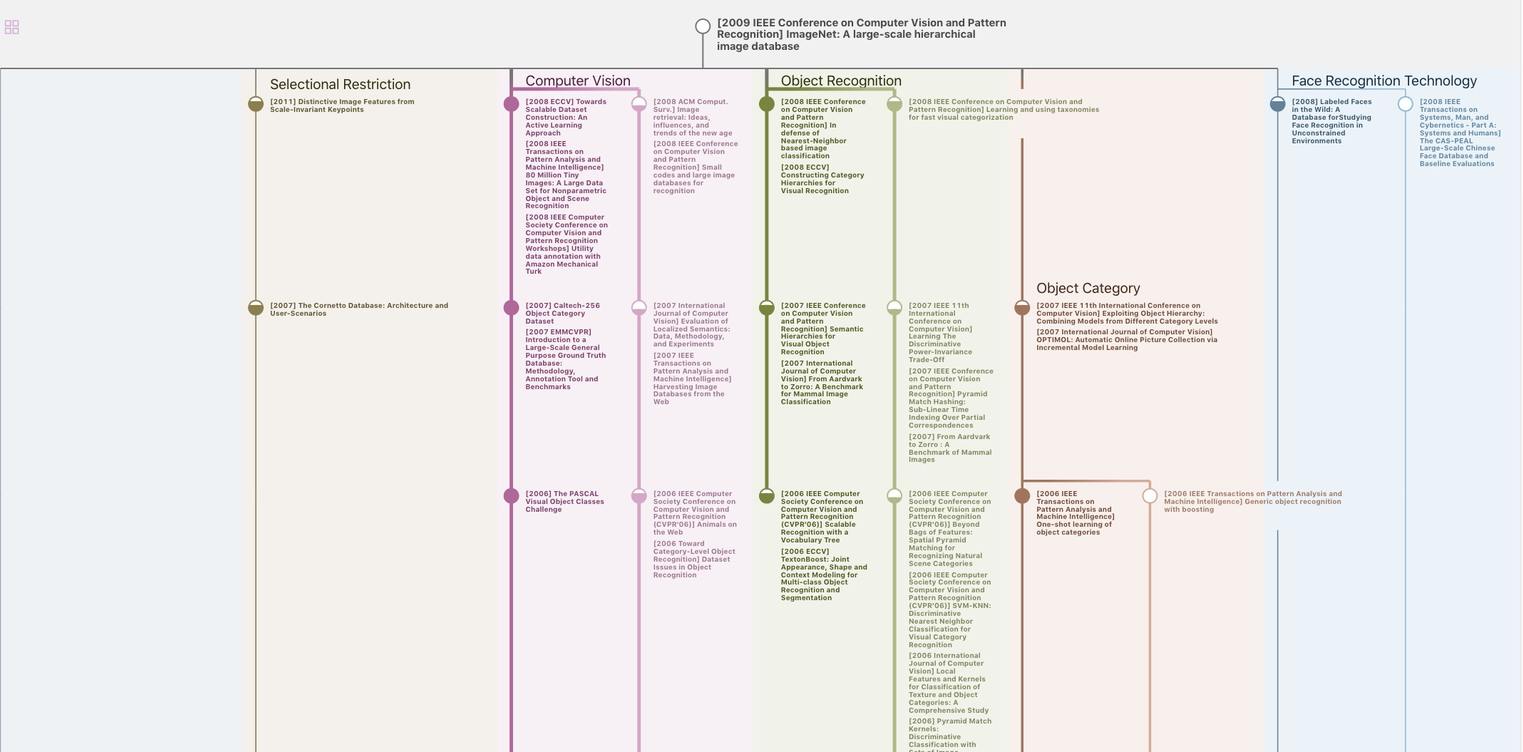
生成溯源树,研究论文发展脉络
Chat Paper
正在生成论文摘要