Abstract 3524: Leveraging ChatGPT for literature-based inference of drug-gene relationships in cancer
Cancer Research(2024)
摘要
Abstract Approximately 5,000 new articles are indexed by PubMed daily, many providing critical insights into novel gene-drug relationships. However, searching and distilling the wealth of biomedical literature to infer specific pharmacogenomic relationships between a gene and a drug poses a significant burden for researchers. Recent advances in large language models, such as ChatGPT, offer a promising solution to tackle this challenge. This study aims to prototype a literature-based inference for drug-gene relationships in the context of cancer using ChatGPT, marking an exploratory effort in this emerging domain. Our approach involves developing an automated pipeline that integrates the Application Programming Interfaces (APIs) of PubMed and ChatGPT, specifically GPT-3.5. Given a gene, a drug, and a disease, the pipeline searches PubMed for relevant articles and extracts their abstracts. Leveraging prompt engineering techniques, we formulated a prompt that facilitates accurate summarization of these abstracts. The output of our pipeline includes three key components: 1) a concise, one-sentence summary elucidating the relationship between the drug-gene pair; 2) a step-by-step explanation of the inference process; and 3) the confidence level associated with the generated summary. Our approach was able to confirm well-known gene-drug relationships in cancer, such as palbociclib and CDK4. We further examined a challenging case of CX-5461, an inhibitor of ribosomal RNA synthesis currently under clinical investigation for various cancers. Until recent evidence emerged in 2021, this drug was mischaracterized as an RNA-Pol I inhibitor, whereas it primarily targets topoisomerase II beta (TOP2B). Notably, our pipeline correctly identified TOP2B as the primary target of CX-5461, despite an extensive body of literature prior to 2021 supporting the RNA-Pol I relationship. To evaluate the sensitivity of our pipeline, we conducted a systematic assessment using a mixture of relevant and irrelevant abstracts. Utilizing bevacizumab, VEGF, and hepatocellular carcinoma as a demonstrating example, we showed that ChatGPT achieved nearly 95% accuracy even when only 30% of the abstracts were relevant to the case. In summary, this pilot research serves as a foundational step towards the utilization of large language models in the field of drug discovery and development. Our ongoing efforts involve the rigorous evaluation of our approach across a diverse spectrum of drug targets and cancer types, as well as the optimization of prompts through state-of-the-art prompt engineering techniques. Citation Format: Yuna Shin, Michael Ning, Li-Ju Wang, Yufei Huang, Yu-Chiao Chiu. Leveraging ChatGPT for literature-based inference of drug-gene relationships in cancer [abstract]. In: Proceedings of the American Association for Cancer Research Annual Meeting 2024; Part 1 (Regular Abstracts); 2024 Apr 5-10; San Diego, CA. Philadelphia (PA): AACR; Cancer Res 2024;84(6_Suppl):Abstract nr 3524.
更多查看译文
AI 理解论文
溯源树
样例
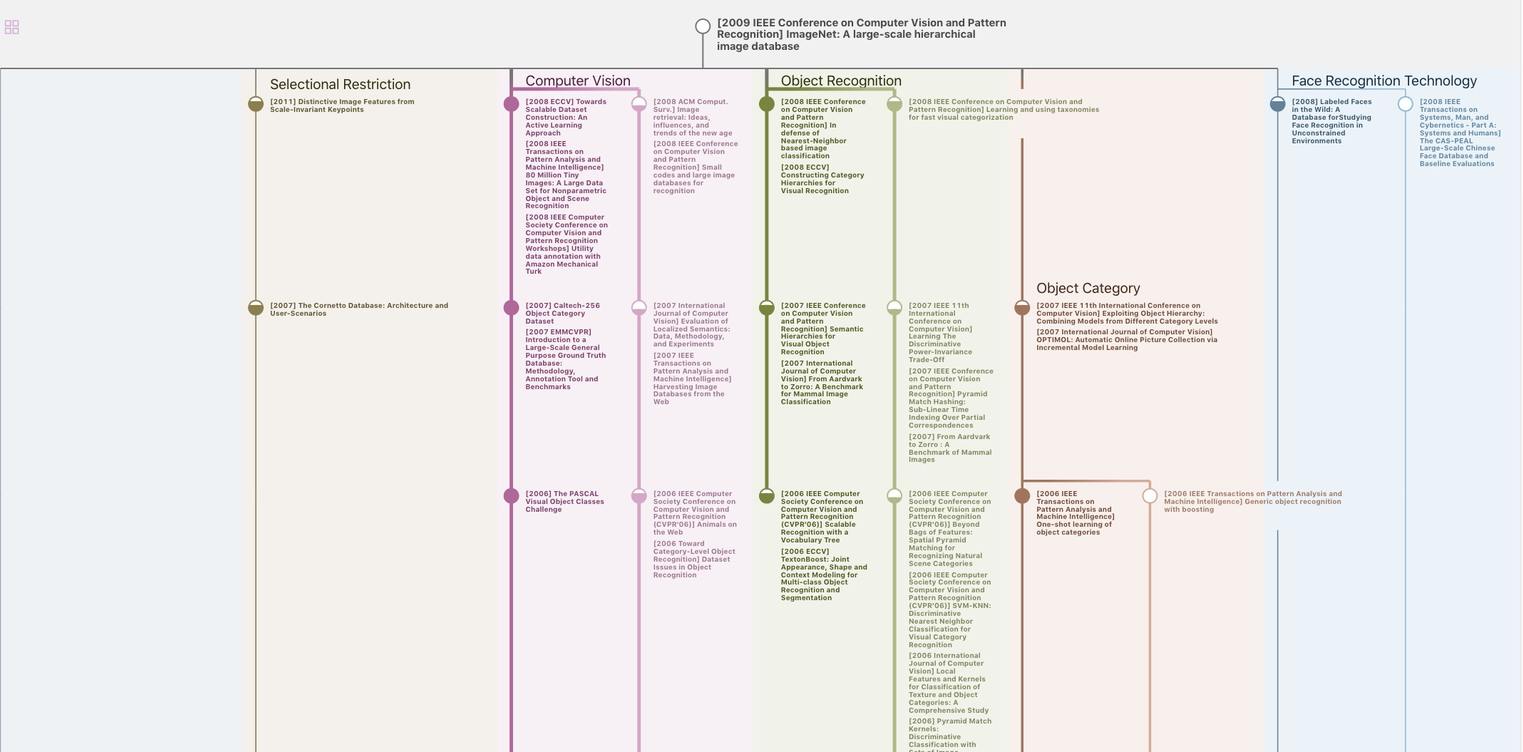
生成溯源树,研究论文发展脉络
Chat Paper
正在生成论文摘要