Abstract 886: Integrated deep learning model for predicting DNA methylation and tumor types from histopathology in central nervous system tumors
Cancer Research(2024)
摘要
Abstract Precision in diagnosis of diverse central nervous system (CNS) tumor types is crucial for optimal patient treatment. DNA methylation profiles, which capture the methylation status of thousands of individual CpG sites, are data-driven means to enhance diagnostic accuracy, but this technique is expensive, time-consuming, and not yet routinely available. To address this, we developed DEPLOY, a deep-learning model that predicts 10 major categories of CNS tumors from histopathology. DEPLOY integrates three distinct components: the first classifies CNS tumors directly from histopathology slide images (‘direct model’); the second initially generates predictions for DNA methylation beta values, which are subsequently used for tumor classification (‘indirect model’); and the third classifies tumor types directly from routinely available patient demographics. First, we find that DEPLOY accurately predicted beta values from histopathology images, suggesting that application of inferred methylation data is a promising approach for deep learning histopathology-based classifiers. Using a 10-class model trained on an internal dataset, we apply this model on two independent external test datasets of 1,522 and 348 cases, achieving top-1 accuracies of 96% and 94%, respectively on samples which are predicted with high confidence. Taken together, DEPLOY could assist pathologists in diagnosing CNS tumors, in an equitable very low-cost manner, within a clinically relevant short time frame. Citation Format: Eldad David Shulman, Danh-Tai Hoang, Rust Turakulov, Zied Abdullaev, Omkar Singh, Emma M. Campagnolo, Eric A. Stone, MacLean P. Nasrallah, Eytan Ruppin, Kenneth Aldape. Integrated deep learning model for predicting DNA methylation and tumor types from histopathology in central nervous system tumors [abstract]. In: Proceedings of the American Association for Cancer Research Annual Meeting 2024; Part 1 (Regular Abstracts); 2024 Apr 5-10; San Diego, CA. Philadelphia (PA): AACR; Cancer Res 2024;84(6_Suppl):Abstract nr 886.
更多查看译文
AI 理解论文
溯源树
样例
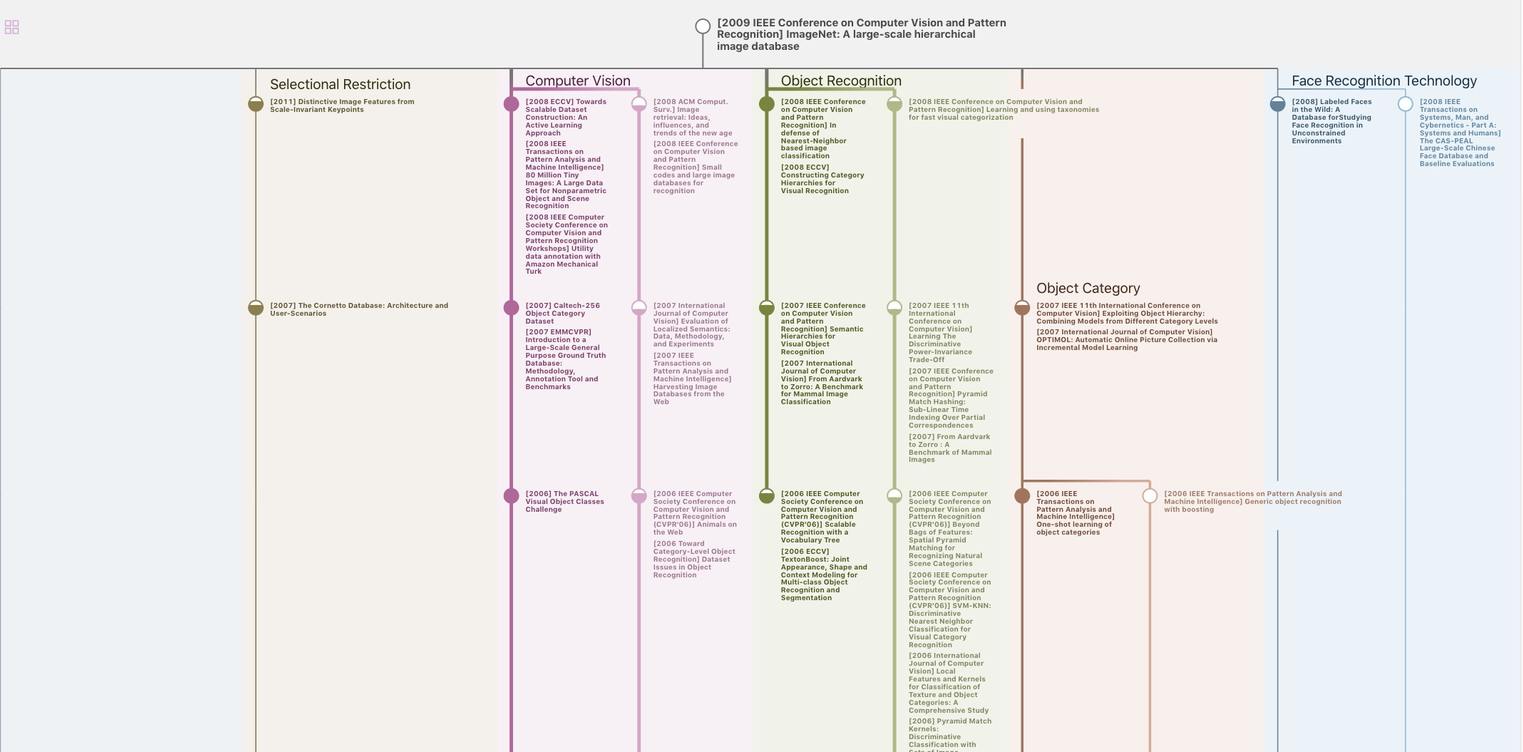
生成溯源树,研究论文发展脉络
Chat Paper
正在生成论文摘要