Advancing Personal Well-Being Analysis through the Integration of Instance-Based Learning with Interpretable AI Techniques
crossref(2024)
摘要
Abstract Understanding factors that affect Personal Well-Being Index (PWI) is crucial for developing strategies that enhance quality of life at both individual and community levels. This research introduces a novel approach to PWI analysis by integrating Instance-Based Learning (IBL) with interpretable Artificial Intelligence (AI) methods. Utilizing IBL, the study creates tailored matrices for individual instances, enabling a dynamic and specific analysis of well-being factors. It leverages advanced AI techniques, notably the graph-based clustering and Locally Weighted Scatterplot Smoothing (LOWESS), to provide transparent and comprehensible insights. Key innovations include the use of sophisticated feature selection techniques like Random Forest (RF), and Permutation Importance (PI) to identify critical PWI indicators. The research also employs LOWESS for state-of-the-art prediction modeling, providing adaptable forecasts for future trends in PWI indicators. The application of graph-based clustering offers a comprehensive analysis of the current state of PWI, generating detailed scenarios and enhancing the understanding of well-being. This integrative methodology positions the study at the forefront of PWI research, offering new pathways for enhancing well-being in diverse settings.
更多查看译文
AI 理解论文
溯源树
样例
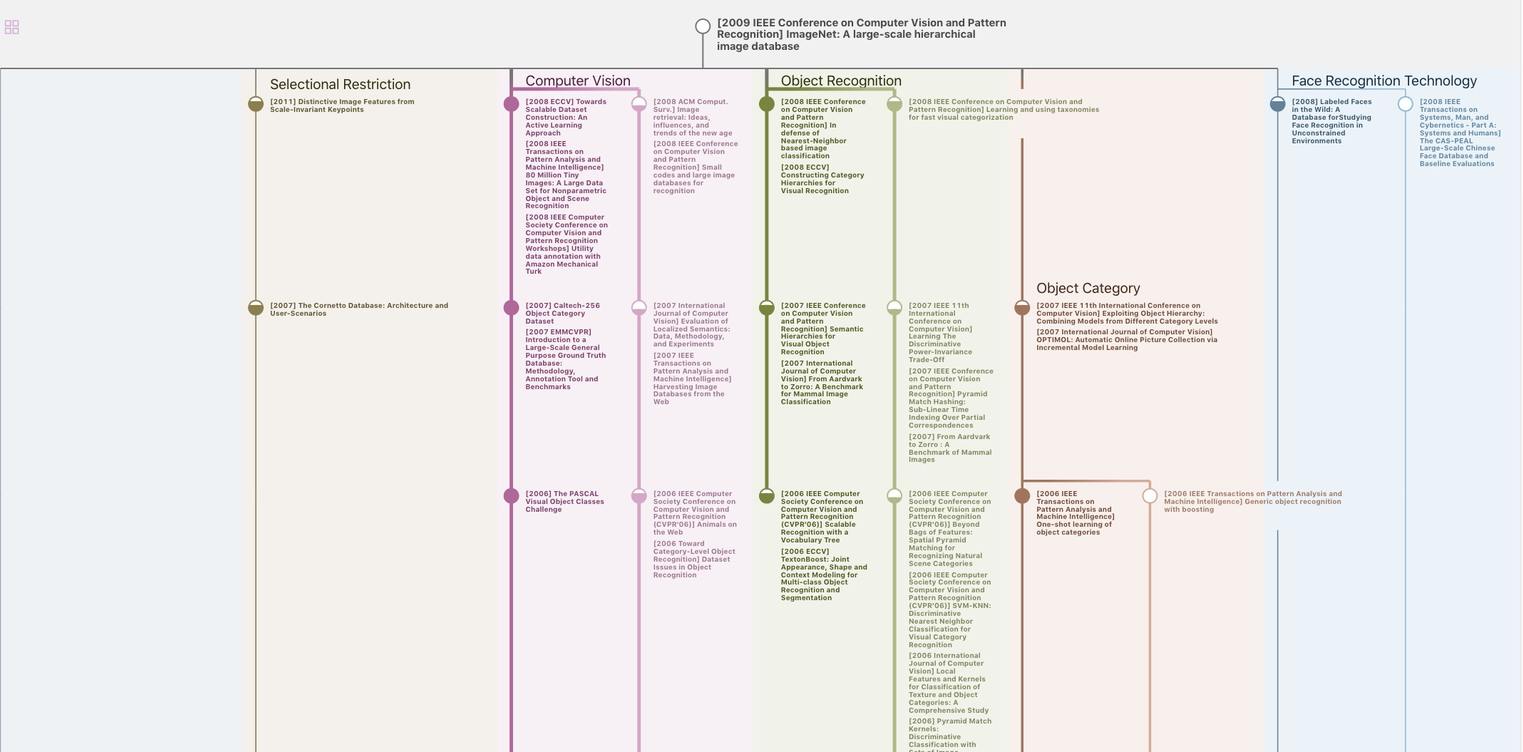
生成溯源树,研究论文发展脉络
Chat Paper
正在生成论文摘要