Network Learning with Directional Sign Patterns
arxiv(2024)
摘要
Complex systems can be effectively modeled via graphs that encode networked
interactions, where relations between entities or nodes are often quantified by
signed edge weights, e.g., promotion/inhibition in gene regulatory networks, or
encoding political of friendship differences in social networks. However, it is
often the case that only an aggregate consequence of such edge weights that
characterize relations may be directly observable, as in protein expression of
in gene regulatory networks. Thus, learning edge weights poses a significant
challenge that is further exacerbated for intricate and large-scale networks.
In this article, we address a model problem to determine the strength of
sign-indefinite relations that explain marginal distributions that constitute
our data. To this end, we develop a paradigm akin to that of the Schrödinger
bridge problem and an efficient Sinkhorn type algorithm (more properly,
Schrödinger-Fortet-Sinkhorn algorithm) that allows fast convergence to
parameters that minimize a relative entropy/likelihood criterion between the
sought signed adjacency matrix and a prior. The formalism that we present
represents a novel generalization of the earlier Schrödinger formalism in
that marginal computations may incorporate weights that model directionality in
underlying relations, and further, that it can be extended to high-order
networks – the Schrödinger-Fortet-Sinkhorn algorithm that we derive is
applicable all the same and allows geometric convergence to a sought
sign-indefinite adjacency matrix or tensor, for high-order networks. We
demonstrate our framework with synthetic and real-world examples.
更多查看译文
AI 理解论文
溯源树
样例
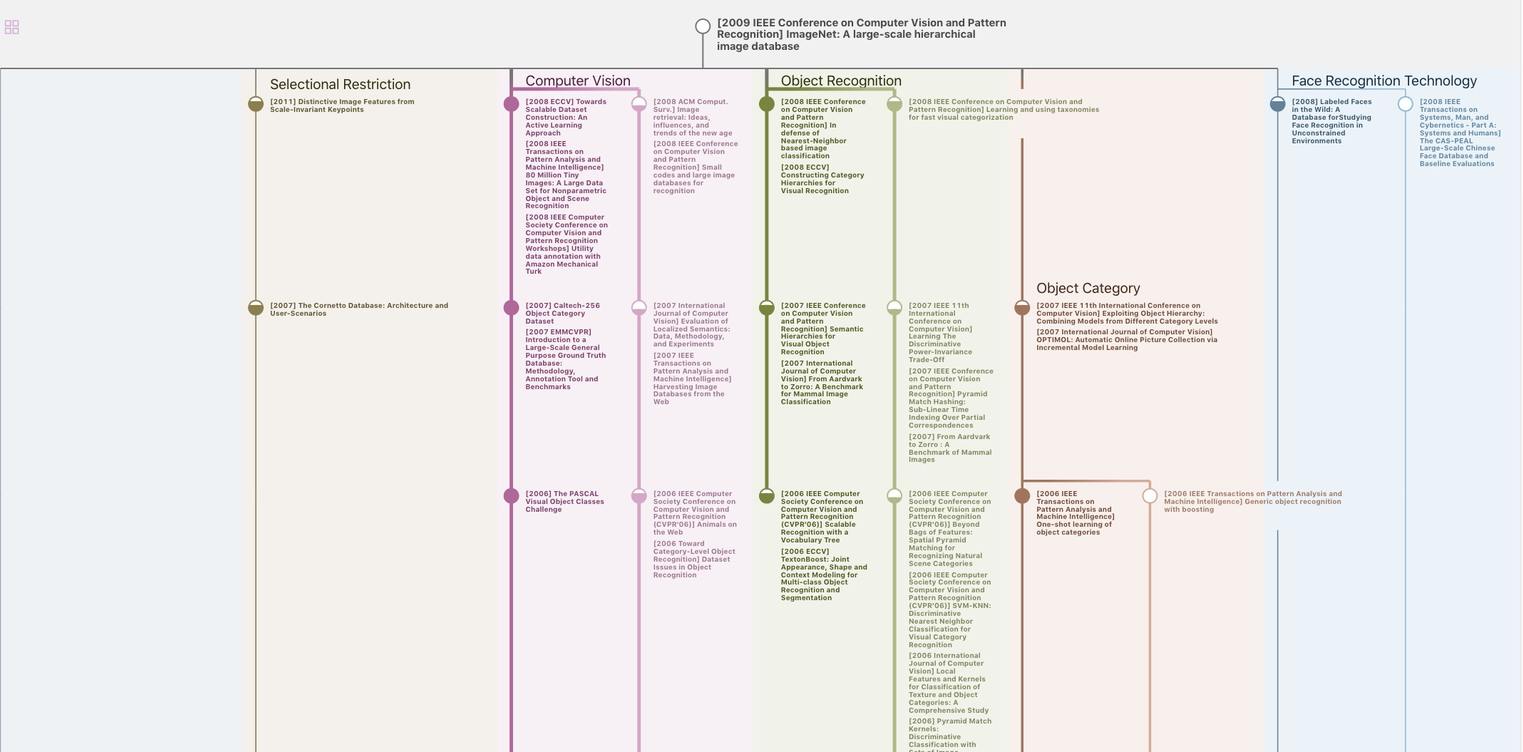
生成溯源树,研究论文发展脉络
Chat Paper
正在生成论文摘要