Galaxy merger challenge: A comparison study between machine learning-based detection methods
arxiv(2024)
摘要
Various galaxy merger detection methods have been applied to diverse
datasets. However, it is difficult to understand how they compare. We aim to
benchmark the relative performance of machine learning (ML) merger detection
methods. We explore six leading ML methods using three main datasets. The first
one (the training data) consists of mock observations from the IllustrisTNG
simulations and allows us to quantify the performance metrics of the detection
methods. The second one consists of mock observations from the Horizon-AGN
simulations, introduced to evaluate the performance of classifiers trained on
different, but comparable data. The third one consists of real observations
from the Hyper Suprime-Cam Subaru Strategic Program (HSC-SSP) survey. For the
binary classification task (mergers vs. non-mergers), all methods perform
reasonably well in the domain of the training data. At 0.1更多
查看译文
AI 理解论文
溯源树
样例
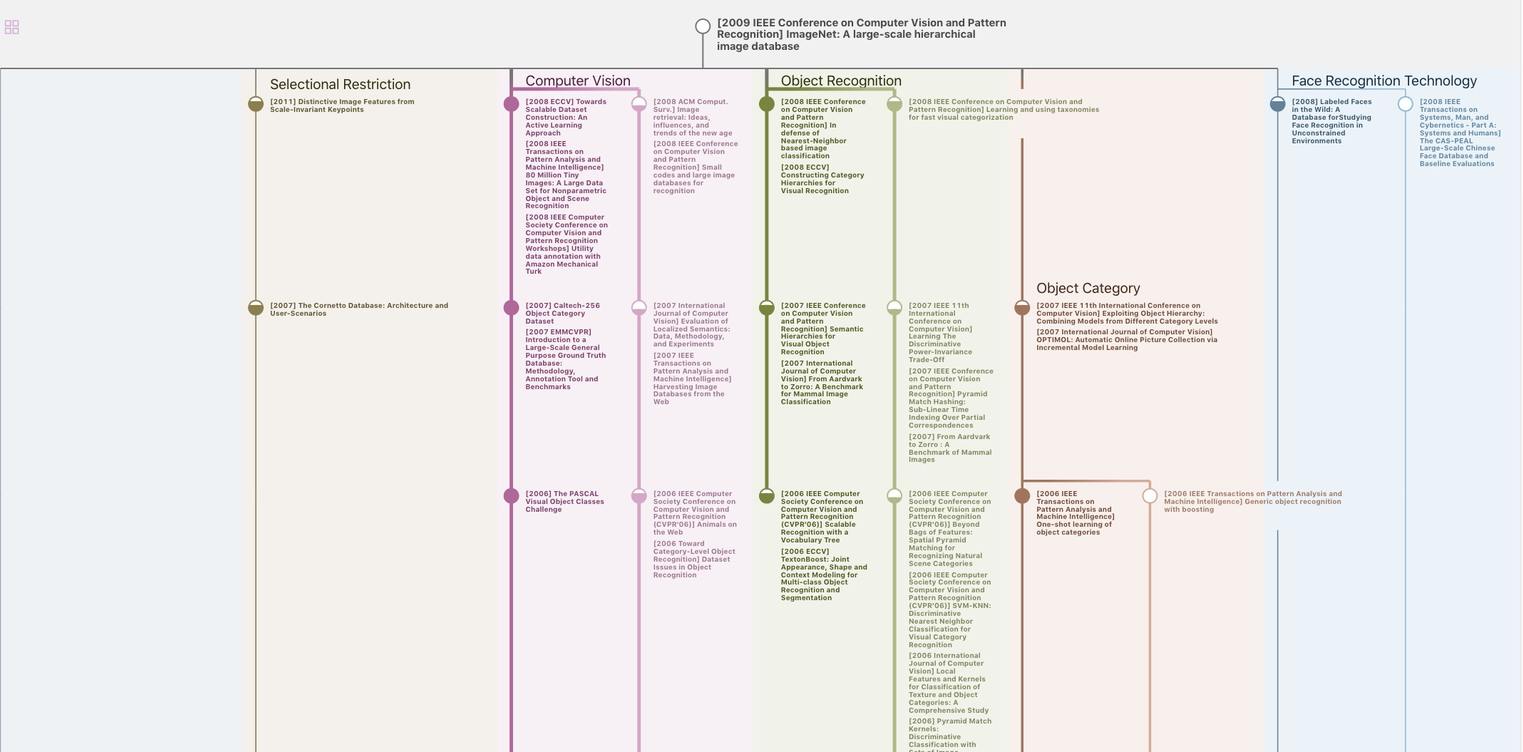
生成溯源树,研究论文发展脉络
Chat Paper
正在生成论文摘要