From prediction to prevention: Machine learning revolutionizes hepatocellular carcinoma recurrence monitoring
WORLD JOURNAL OF GASTROENTEROLOGY(2024)
摘要
In this editorial, we comment on the article by Zhang et al entitled Development of a machine learning-based model for predicting the risk of early postoperative recurrence of hepatocellular carcinoma. Hepatocellular carcinoma (HCC), which is characterized by high incidence and mortality rates, remains a major global health challenge primarily due to the critical issue of postoperative recurrence. Early recurrence, defined as recurrence that occurs within 2 years posttreatment, is linked to the hidden spread of the primary tumor and significantly impacts patient survival. Traditional predictive factors, including both patient- and treatment-related factors, have limited predictive ability with respect to HCC recurrence. The integration of machine learning algorithms is fueled by the exponential growth of computational power and has revolutionized HCC research. The study by Zhang et al demonstrated the use of a groundbreaking preoperative prediction model for early postoperative HCC recurrence. Chall-enges persist, including sample size constraints, issues with handling data, and the need for further validation and interpretability. This study emphasizes the need for collaborative efforts, multicenter studies and comparative analyses to validate and refine the model. Overcoming these challenges and exploring innovative approaches, such as multi-omics integration, will enhance personalized oncology care. This study marks a significant stride toward precise, effi-cient, and personalized oncology practices, thus offering hope for improved patient outcomes in the field of HCC treatment.
更多查看译文
关键词
Hepatocellular carcinoma,Early recurrence,Machine learning,XGBoost model,Predictive precision medicine,Clinical utility,Personalized interventions
AI 理解论文
溯源树
样例
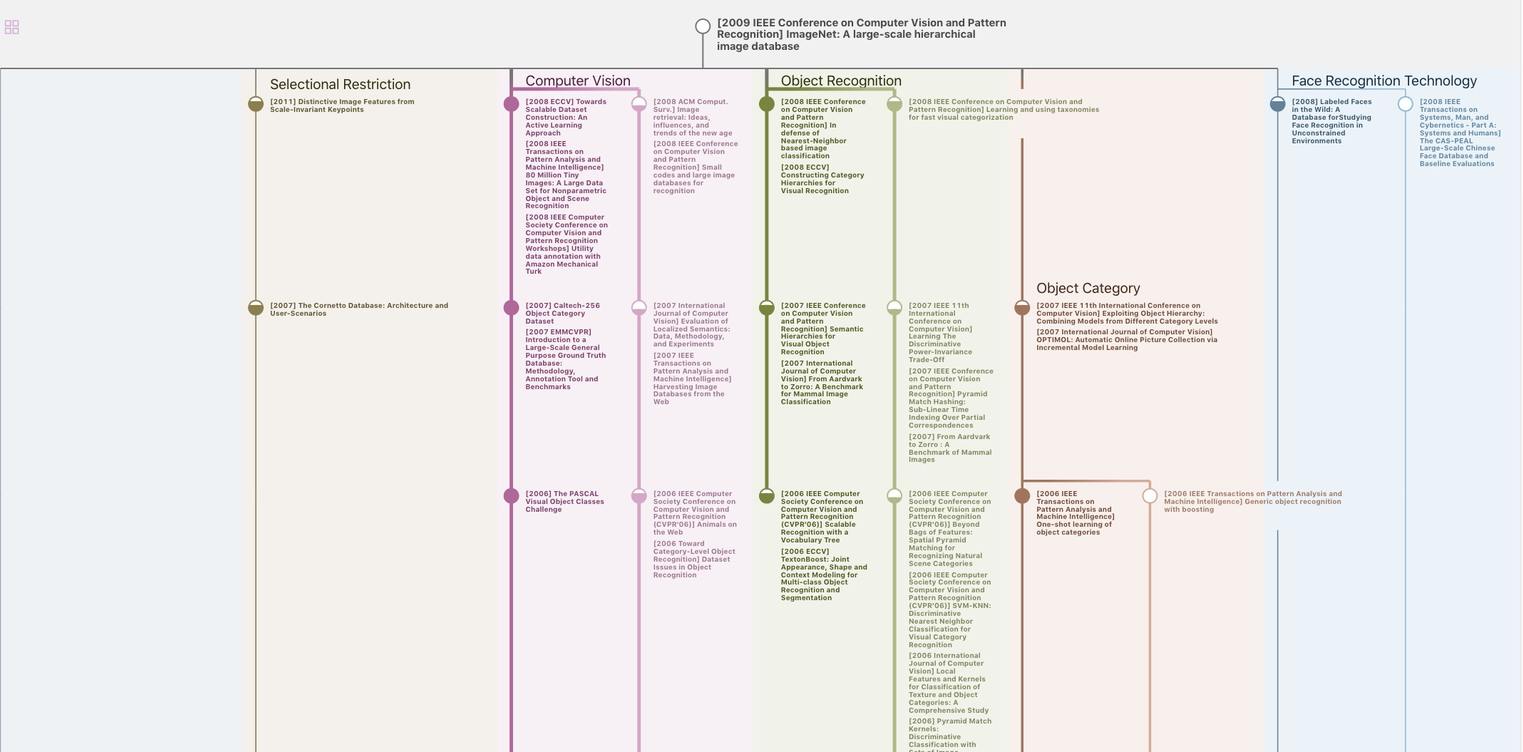
生成溯源树,研究论文发展脉络
Chat Paper
正在生成论文摘要