OENet: An overexposure correction network fused with residual block and transformer
Expert Systems with Applications(2024)
摘要
With the wide application of vision in the fields of autonomous driving and medical imaging, the demand for overexposure image correction algorithms is becoming increasingly urgent. However, existing overexposure image correction algorithms can lead to problems such as blurring, color bias, and over-enhancement of the generated images. Optimizing overexposure image quality has a significant impact on improving system performance, accuracy, and safety. In this paper, we propose an overexposure image correction network. First, we built the Detail Enhancement Module (DEM). It adopts global average pooling on each channel of the input feature map. After pooling, an activation function is used for nonlinear mapping to generate a channel attention weight vector. And it is multiplied with the original input feature map to achieve the purpose of enhancing the details of the overexposed image. Second, we construct a context-aware backbone (CAB) to extract features such as color and texture. The linear attention gating mechanism replaces the multi-head attention module in Transformer, and reduces the computational complexity in high-resolution images while maintaining performance by learning linear transformation and attention gating. Finally, we design an attention-guided feature fusion (AGFF) to fuse shallow and deep features. It computes weight vectors for shallow features through an attention module. The calculated result is converted to the same dimension as the input feature by bilinear interpolation, so as to enrich the semantic information and detailed information of the generated image. In addition to designing the network structure, we design a hybrid loss function to improve the quality of the generated image from the spatial and structural aspects, and the exposure function can correct the exposure degree of the generated image. Experiments are conducted on two public datasets and the dataset in this paper. Specifically, the PSNR and SSIM of images generated on the dataset MSEC increased by 1.3813% and 5.56%. The PSNR and SSIM of images generated on the dataset SICE increased by 1.545% and 4.64%. The proposed method can effectively generate clear and high-fidelity images.
更多查看译文
关键词
Overexposure,Detail enhancement,Backbone,Transformer,Linear attention,Feature fusion,Loss function
AI 理解论文
溯源树
样例
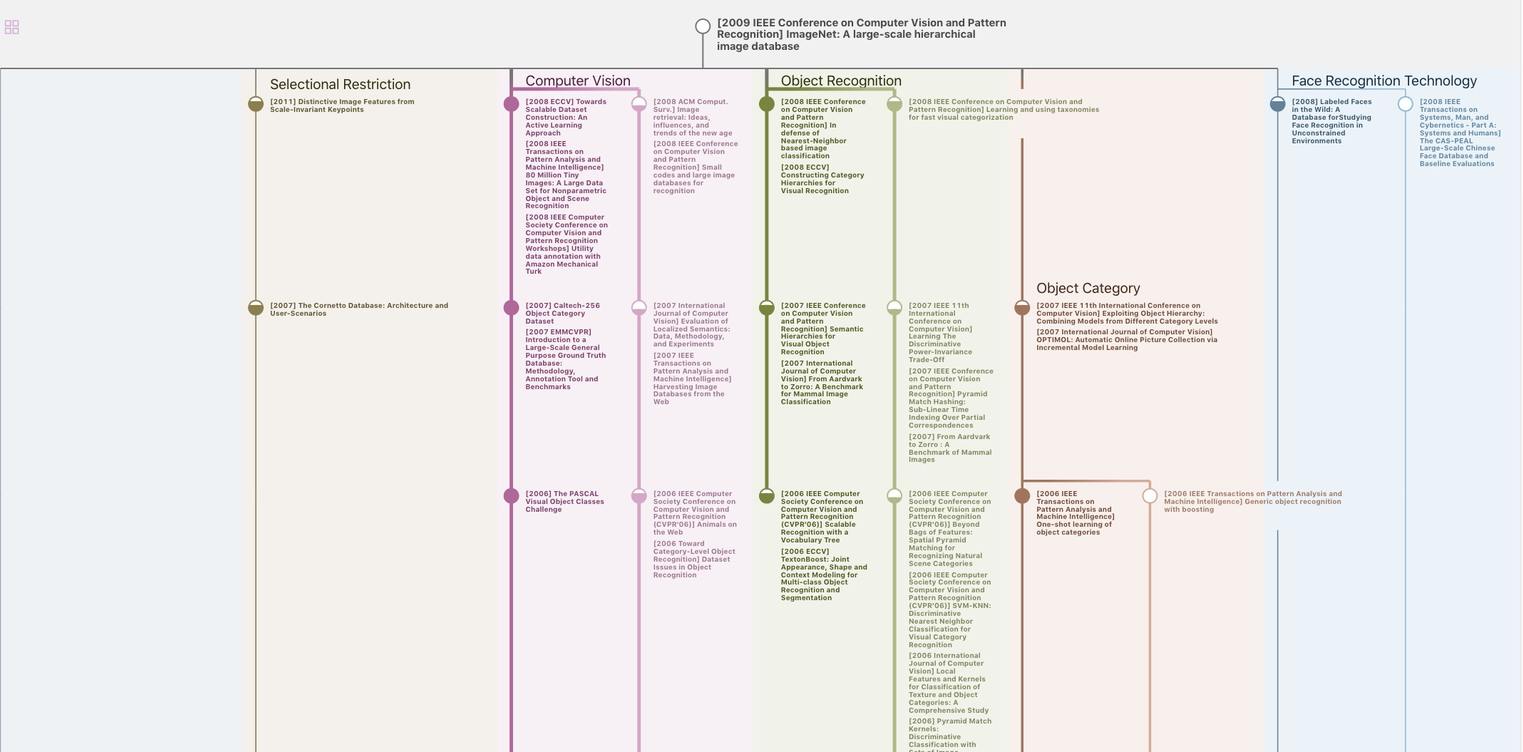
生成溯源树,研究论文发展脉络
Chat Paper
正在生成论文摘要