Cross-user activity recognition via temporal relation optimal transport
CoRR(2024)
摘要
Current research on human activity recognition (HAR) mainly assumes that
training and testing data are drawn from the same distribution to achieve a
generalised model, which means all the data are considered to be independent
and identically distributed (i.i.d.). In many real-world
applications, this assumption does not hold, and collected training and target
testing datasets have non-uniform distribution, such as in the case of
cross-user HAR. Domain adaptation is a promising approach for cross-user HAR
tasks. Existing domain adaptation works based on the assumption that samples in
each domain are i.i.d. and do not consider the knowledge of
temporal relation hidden in time series data for aligning data distribution.
This strong assumption of i.i.d. may not be suitable for time
series-related domain adaptation methods because the samples formed by time
series segmentation and feature extraction techniques are only coarse
approximations to i.i.d. assumption in each domain. In this
paper, we propose the temporal relation optimal transport (TROT) method to
utilise temporal relation and relax the i.i.d. assumption for
the samples in each domain for accurate and efficient knowledge transfer. We
obtain the temporal relation representation and implement temporal relation
alignment of activities via the Hidden Markov model (HMM) and optimal transport
(OT) techniques. Besides, a new regularisation term that preserves temporal
relation order information for an improved optimal transport mapping is
proposed to enhance the domain adaptation performance. Comprehensive
experiments are conducted on three public activity recognition datasets (i.e.
OPPT, PAMAP2 and DSADS), demonstrating that TROT outperforms other
state-of-the-art methods.
更多查看译文
AI 理解论文
溯源树
样例
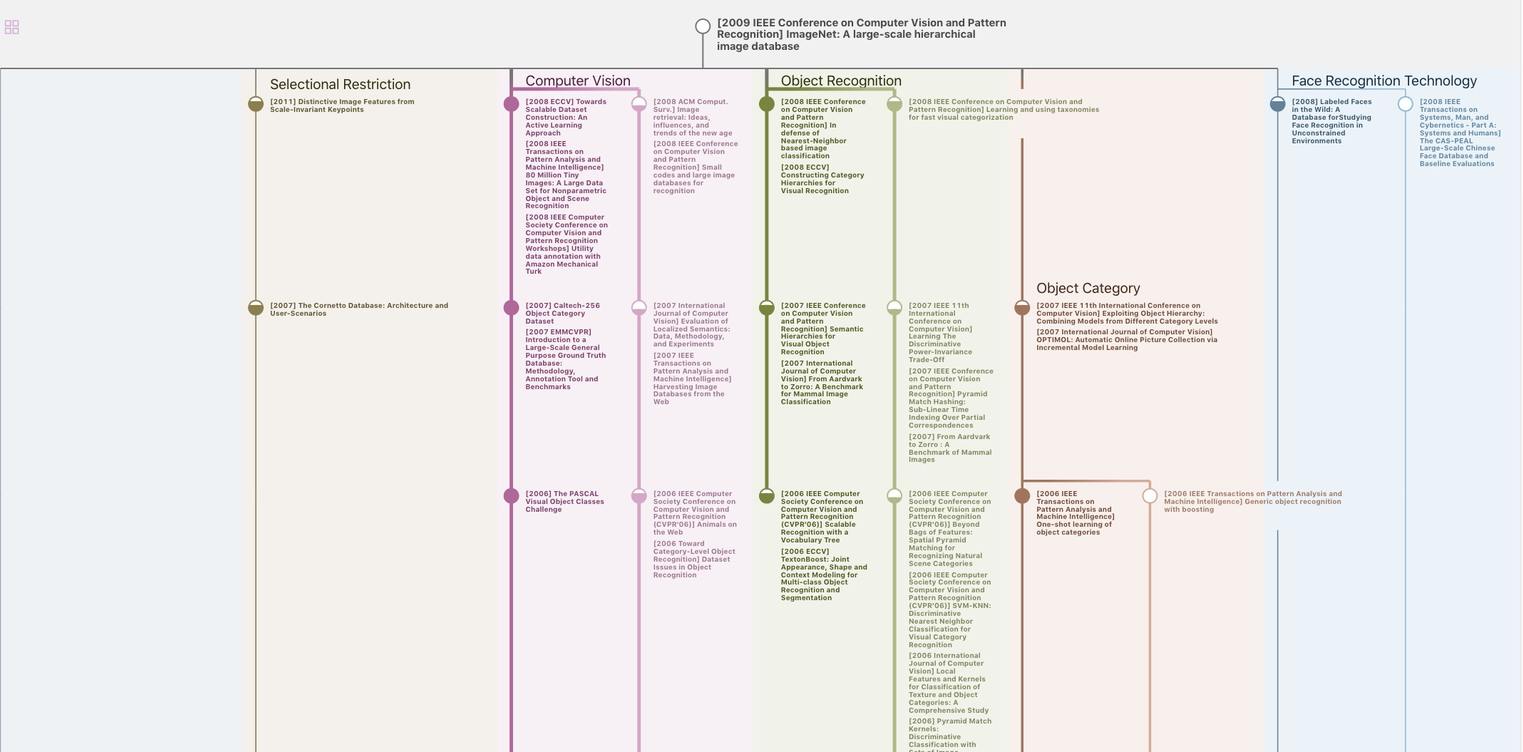
生成溯源树,研究论文发展脉络
Chat Paper
正在生成论文摘要