Data-Driven Approximation of Stationary Nonlinear Filters with Optimal Transport Maps
arxiv(2024)
摘要
The nonlinear filtering problem is concerned with finding the conditional
probability distribution (posterior) of the state of a stochastic dynamical
system, given a history of partial and noisy observations. This paper presents
a data-driven nonlinear filtering algorithm for the case when the state and
observation processes are stationary. The posterior is approximated as the
push-forward of an optimal transport (OT) map from a given distribution, that
is easy to sample from, to the posterior conditioned on a truncated observation
window. The OT map is obtained as the solution to a stochastic optimization
problem that is solved offline using recorded trajectory data from the state
and observations. An error analysis of the algorithm is presented under the
stationarity and filter stability assumptions, which decomposes the error into
two parts related to the truncation window during training and the error due to
the optimization procedure. The performance of the proposed method, referred to
as optimal transport data-driven filter (OT-DDF), is evaluated for several
numerical examples, highlighting its significant computational efficiency
during the online stage while maintaining the flexibility and accuracy of OT
methods in nonlinear filtering.
更多查看译文
AI 理解论文
溯源树
样例
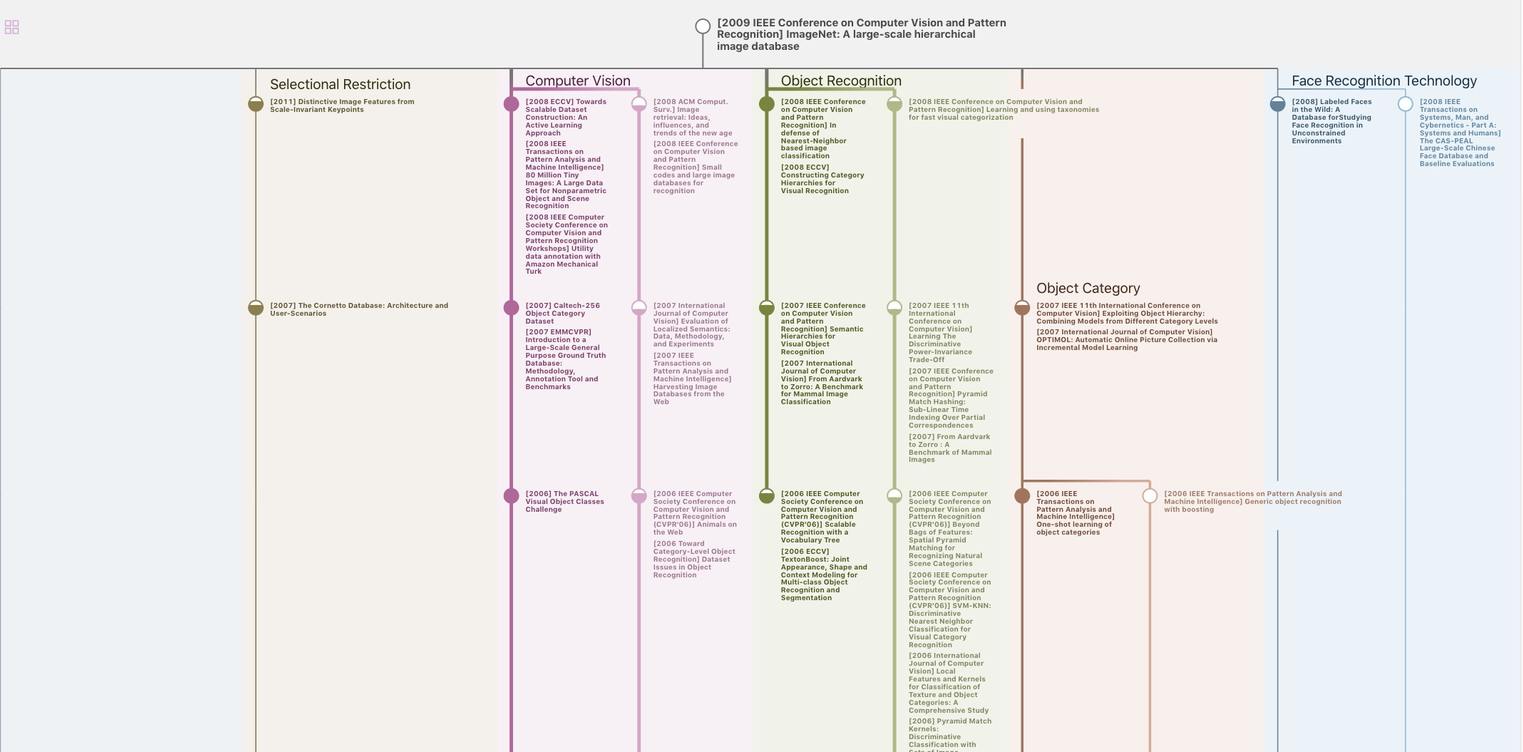
生成溯源树,研究论文发展脉络
Chat Paper
正在生成论文摘要