The Effectiveness of Local Updates for Decentralized Learning under Data Heterogeneity
CoRR(2024)
摘要
We revisit two fundamental decentralized optimization methods, Decentralized
Gradient Tracking (DGT) and Decentralized Gradient Descent (DGD), with multiple
local updates. We consider two settings and demonstrate that incorporating K >
1 local update steps can reduce communication complexity. Specifically, for
μ-strongly convex and L-smooth loss functions, we proved that local DGT
achieves communication complexity 𝒪̃(L/μ K +
δ/μ (1 - ρ) + ρ/(1 - ρ)^2·L+
δ/μ), where ρ measures the network connectivity and δ
measures the second-order heterogeneity of the local loss. Our result reveals
the tradeoff between communication and computation and shows increasing K can
effectively reduce communication costs when the data heterogeneity is low and
the network is well-connected. We then consider the over-parameterization
regime where the local losses share the same minimums, we proved that employing
local updates in DGD, even without gradient correction, can yield a similar
effect as DGT in reducing communication complexity. Numerical experiments
validate our theoretical results.
更多查看译文
AI 理解论文
溯源树
样例
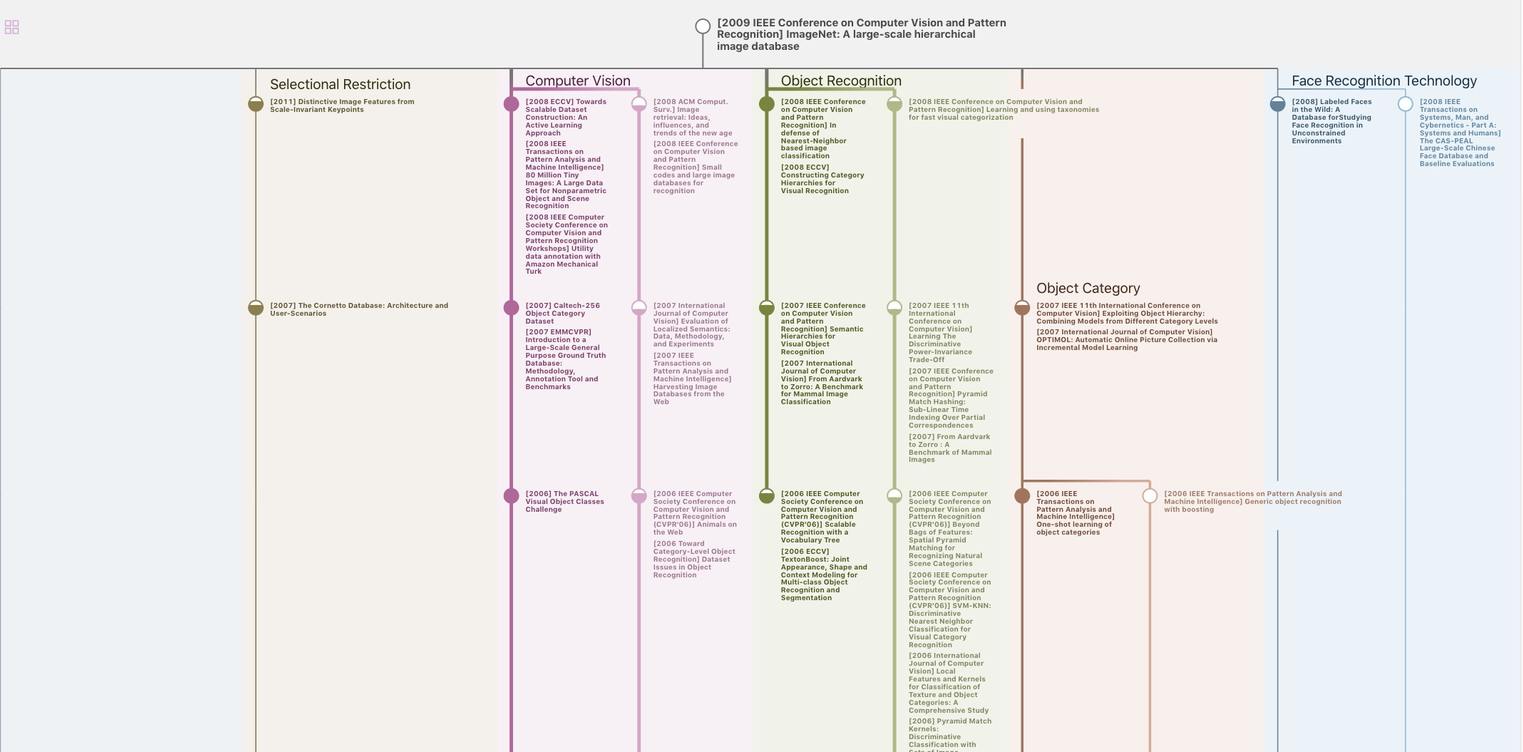
生成溯源树,研究论文发展脉络
Chat Paper
正在生成论文摘要