Towards Human-Like Machine Comprehension: Few-Shot Relational Learning in Visually-Rich Documents
arXiv (Cornell University)(2024)
Abstract
Key-value relations are prevalent in Visually-Rich Documents (VRDs), oftendepicted in distinct spatial regions accompanied by specific color and fontstyles. These non-textual cues serve as important indicators that greatlyenhance human comprehension and acquisition of such relation triplets. However,current document AI approaches often fail to consider this valuable priorinformation related to visual and spatial features, resulting in suboptimalperformance, particularly when dealing with limited examples. To address thislimitation, our research focuses on few-shot relational learning, specificallytargeting the extraction of key-value relation triplets in VRDs. Given theabsence of a suitable dataset for this task, we introduce two new few-shotbenchmarks built upon existing supervised benchmark datasets. Furthermore, wepropose a variational approach that incorporates relational 2D-spatial priorsand prototypical rectification techniques. This approach aims to generaterelation representations that are more aware of the spatial context and unseenrelation in a manner similar to human perception. Experimental resultsdemonstrate the effectiveness of our proposed method by showcasing its abilityto outperform existing methods. This study also opens up new possibilities forpractical applications.
MoreTranslated text
Key words
Information Retrieval,Word Representation,Machine Translation,Topic Modeling,Named Entity Recognition
PDF
View via Publisher
AI Read Science
Must-Reading Tree
Example
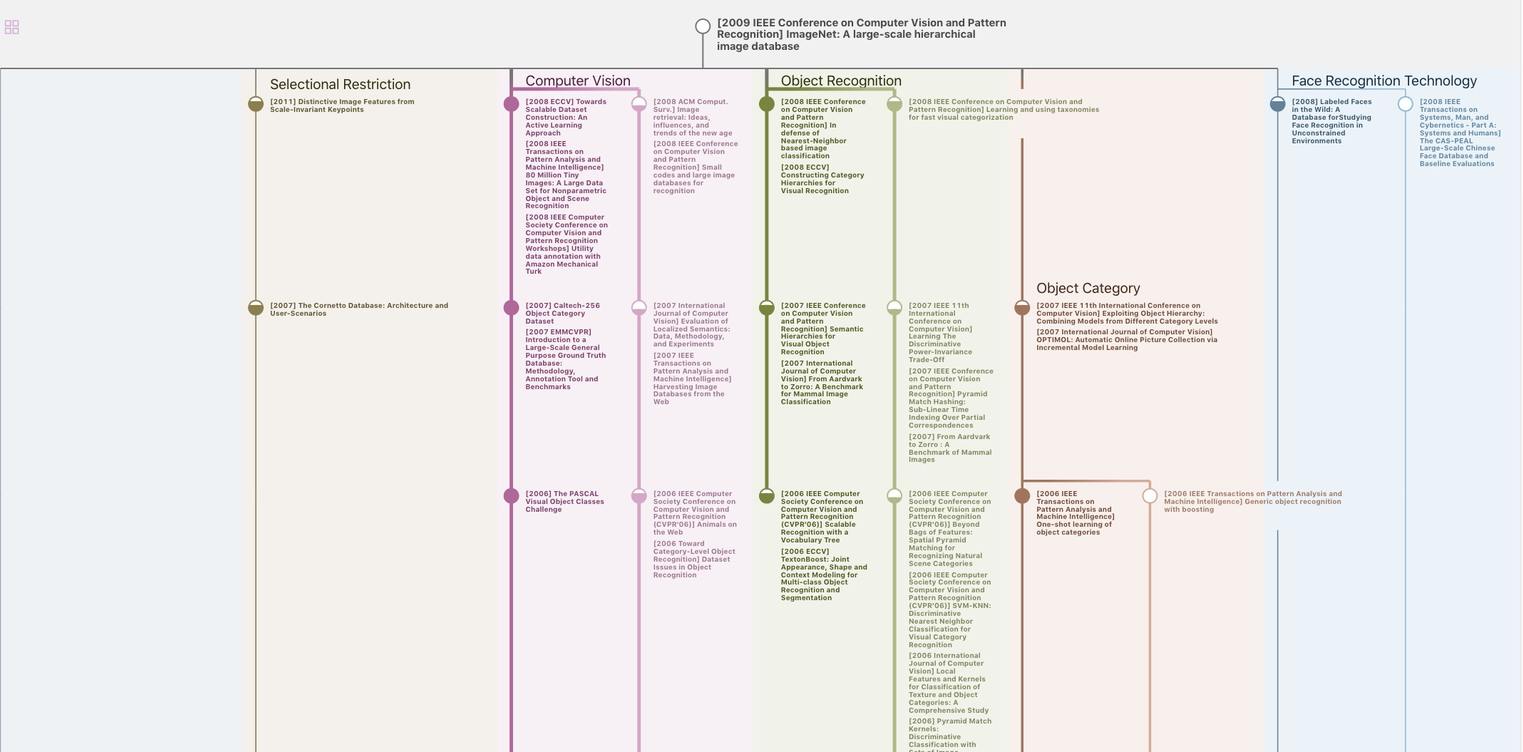
Generate MRT to find the research sequence of this paper
Data Disclaimer
The page data are from open Internet sources, cooperative publishers and automatic analysis results through AI technology. We do not make any commitments and guarantees for the validity, accuracy, correctness, reliability, completeness and timeliness of the page data. If you have any questions, please contact us by email: report@aminer.cn
Chat Paper
Summary is being generated by the instructions you defined