Illuminating Systematic Trends in Nuclear Data with Generative Machine Learning Models
arxiv(2024)
摘要
We introduce a novel method for studying systematic trends in nuclear
reaction data using generative adversarial networks. Libraries of nuclear cross
section evaluations exhibit intricate systematic trends across the nuclear
landscape, and predictive models capable of reproducing and analyzing these
trends are valuable for many applications. We have developed a predictive model
using deep generative adversarial networks to learn trends from the inelastic
neutron scattering channel of TENDL for even-even nuclei. The system predicts
cross sections based on adding/subtracting particles to/from the target
nucleus. It can thus help identify cross sections that break from expected
trends and predict beyond the limit of current experiments. Our model can
produce good predictions for cross section curves for many nuclides, and it is
most robust near the line of stability. We also create an ensemble of
predictions to leverage different correlations and estimate model uncertainty.
This research marks an important first step in computer generation of nuclear
cross-section libraries.
更多查看译文
AI 理解论文
溯源树
样例
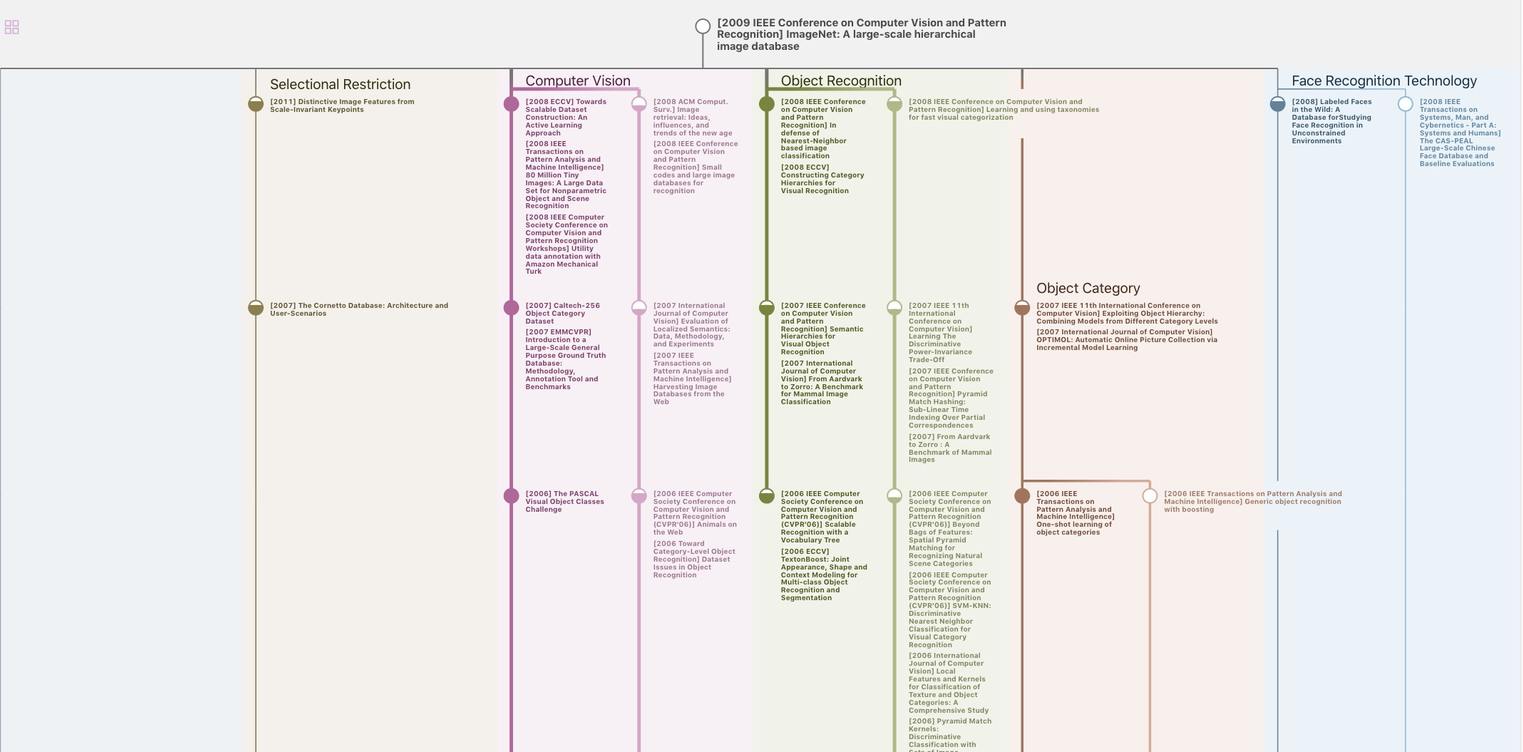
生成溯源树,研究论文发展脉络
Chat Paper
正在生成论文摘要