Improving the Optimization in Model Predictive Controllers: Scheduling Large Groups of Electric Vehicles
arxiv(2024)
摘要
In parking lots with large groups of electric vehicles (EVs), charging has to
happen in a coordinated manner, among others, due to the high load per vehicle
and the limited capacity of the electricity grid. To achieve such coordination,
model predictive control can be applied, thereby repeatedly solving an
optimization problem. Due to its repetitive nature and its dependency on the
time granularity, optimization has to be (computationally) efficient. The work
presented here focuses on that optimization subroutine, its computational
efficiency and how to speed up the optimization for large groups of EVs. In
particular, we adapt FOCS, an algorithm that can solve the underlying
optimization problem, to better suit the repetitive set-up of model predictive
control by adding a pre-mature stop feature. Based on real-world data, we
empirically show that the added feature speeds up the median computation time
for 1-minute granularity by up to 44
that uses maximum flow methods as a subroutine, the impact of choosing various
maximum flow methods on the runtime is investigated. Finally, we compare FOCS
to a commercially available solver, concluding that FOCS outperforms the
state-of-the-art when making a full-day schedule for large groups of EVs.
更多查看译文
AI 理解论文
溯源树
样例
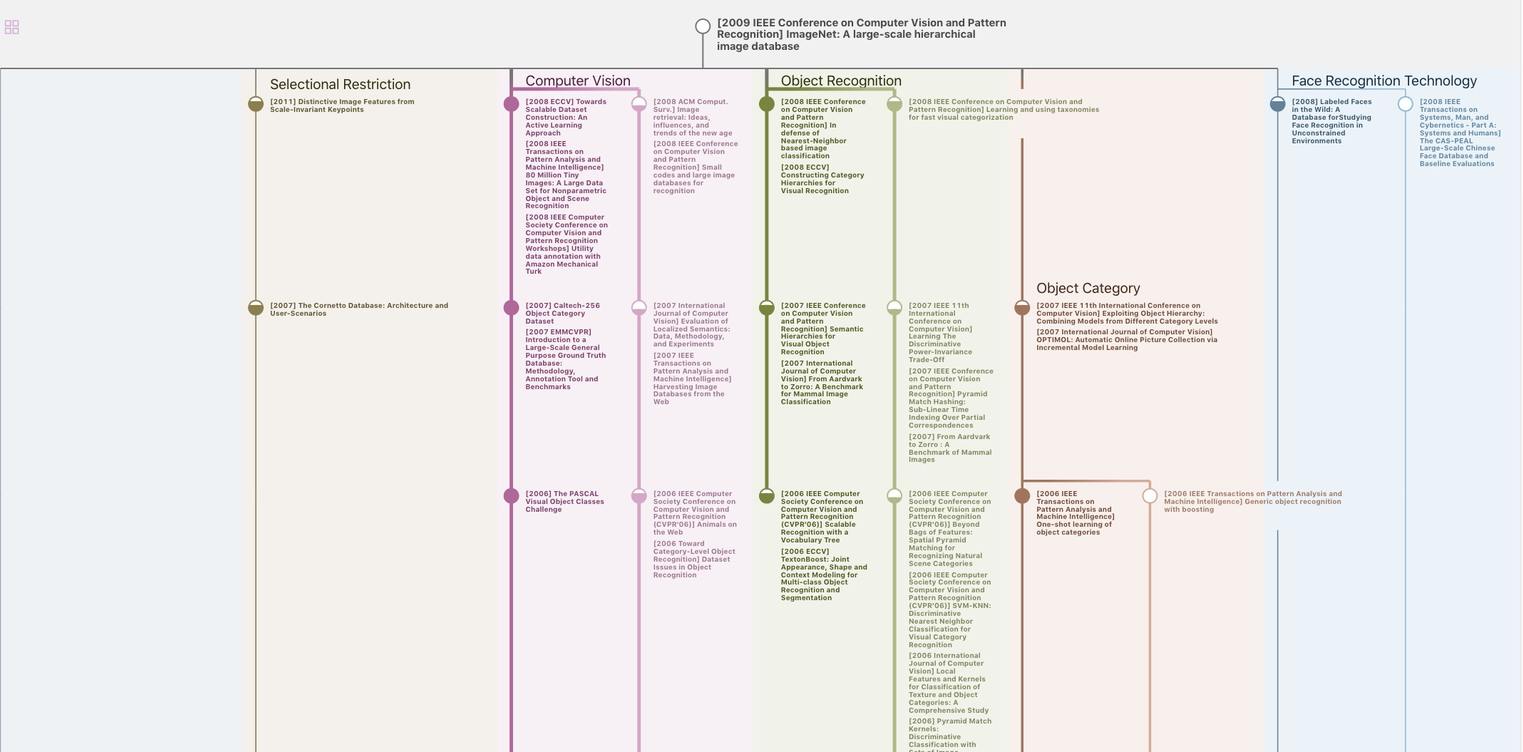
生成溯源树,研究论文发展脉络
Chat Paper
正在生成论文摘要