Joint Low-Light Enhancement and Deblurring with Structural Priors Guidance
Expert systems with applications(2024)
摘要
Images captured under low-light conditions usually co-exist with low light and blur degradation. Most existing cascade and joint enhancement methods may provide undesirable results, suffering from severe artifacts, deteriorating blur, and unclear details. In this paper, we propose a novel network with structural priors, including high-frequency and edge, to enable effective image representation learning for joint low-light enhancement and deblurring. Specifically, we employ a Transformer backbone to explore the global clues of the image. To compensate for the inadequate local detail optimization, we propose a multi-patch perception pyramid block that models the correlation between different size patches and ambiguity, and identifies non-uniform deblurring spatial features, facilitating the reconstruction of potential high-frequency and edge information. Furthermore, a prior-guided reconstruction block based on the parallel attention mechanism is present to adaptively correct global image with statistical features, which helps guide the model to refine sharp texture and structure. Extensive experiments performed on simulated and real-world datasets demonstrate the efficacy of our proposed method in restoring low-light blurry and low-light images with increased visual perception compared to state-of-the-art methods.
更多查看译文
关键词
Low-light enhancement,Deblurring,Structural prior,Deep learning
AI 理解论文
溯源树
样例
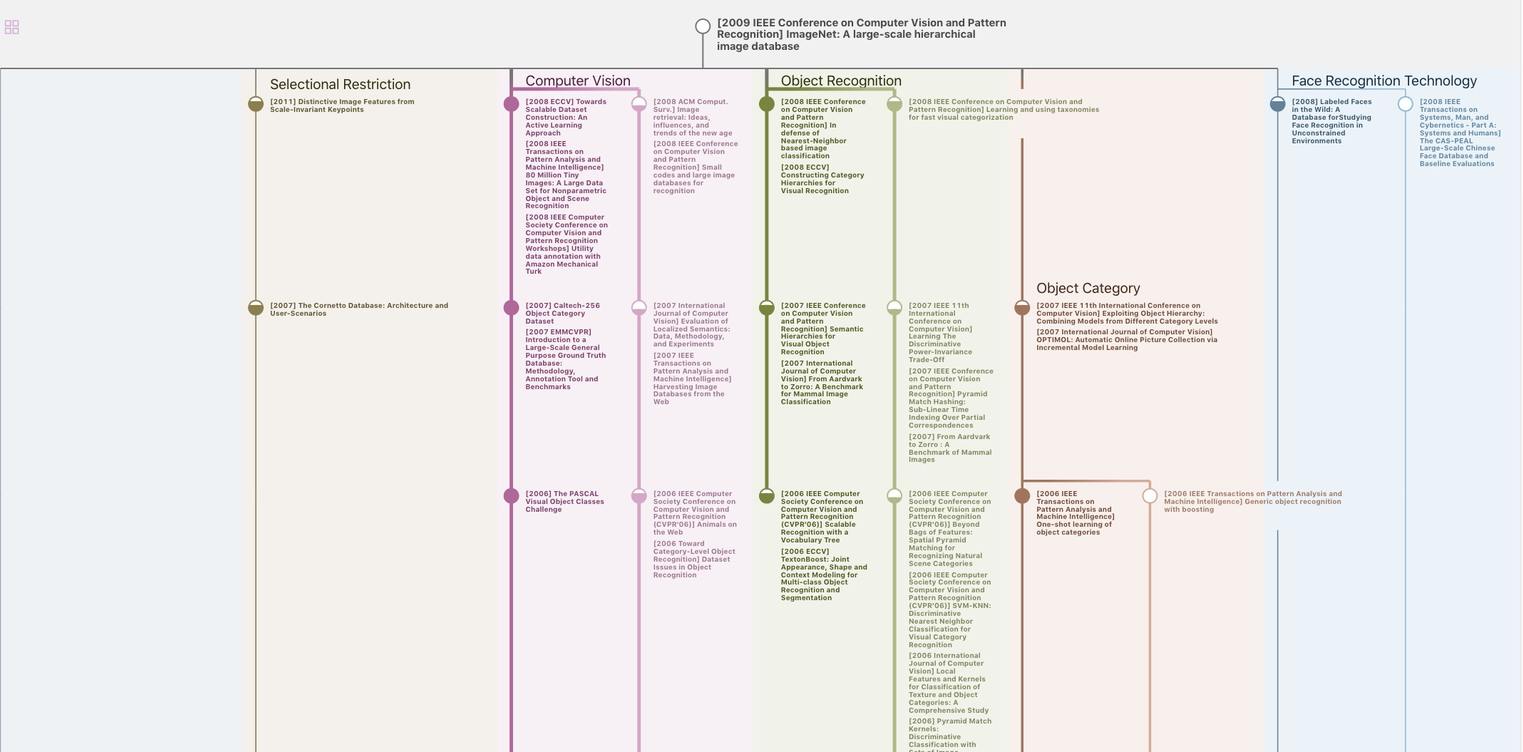
生成溯源树,研究论文发展脉络
Chat Paper
正在生成论文摘要