GCFormer: Global Context-Aware Transformer for Remote Sensing Image Change Detection
IEEE TRANSACTIONS ON GEOSCIENCE AND REMOTE SENSING(2024)
摘要
In recent years, Transformer-based change detection (CD) in remote sensing images has achieved significant advances, making it an emerging hot research topic. However, the current CD methods suffer from some problems, such as incomplete detection of change regions and missed detection of small change regions. In this article, a global context-aware Transformer is proposed for CD tasks, named GCFormer, to address above issues by efficiently enhancing the global context information. It is fulfilled from two aspects based on the hybrid convolutional neural network (CNN) + Transformer framework. First, a multireceptive-field Conv-Attention (MRFCA) mechanism is designed, which combines dilated convolutions with multiple rates (DCMRs) and Conv-Attention, fully leveraging the advantages of convolution operation and self-attention mechanism. It is embedded at the highest layer of CNN to extract multireceptive-field global context information. Second, a context-aware relative position encoding (CRPE) mode is proposed to replace the absolute position encoding (APE) mode of Transformer. As a result, it can capture long-range dependency more efficiently and further enhance the global context information extraction and representation ability of the network. Experimental results on three public benchmark datasets of LEVIR-CD, WHU-CD, and DSIFN-CD show that, the proposed GCFormer achieves superior detection performance with lower model complexity than the state-of-the-art (SOTA) Transformer-based CD methods. The source code is available at https://github.com/yuwanting828/yuwanting828.github.io.
更多查看译文
关键词
Transformers,Feature extraction,Convolutional neural networks,Task analysis,Computer architecture,Remote sensing,Semantics,Change detection (CD),global context-aware Transformer,long-range dependency,remote sensing images
AI 理解论文
溯源树
样例
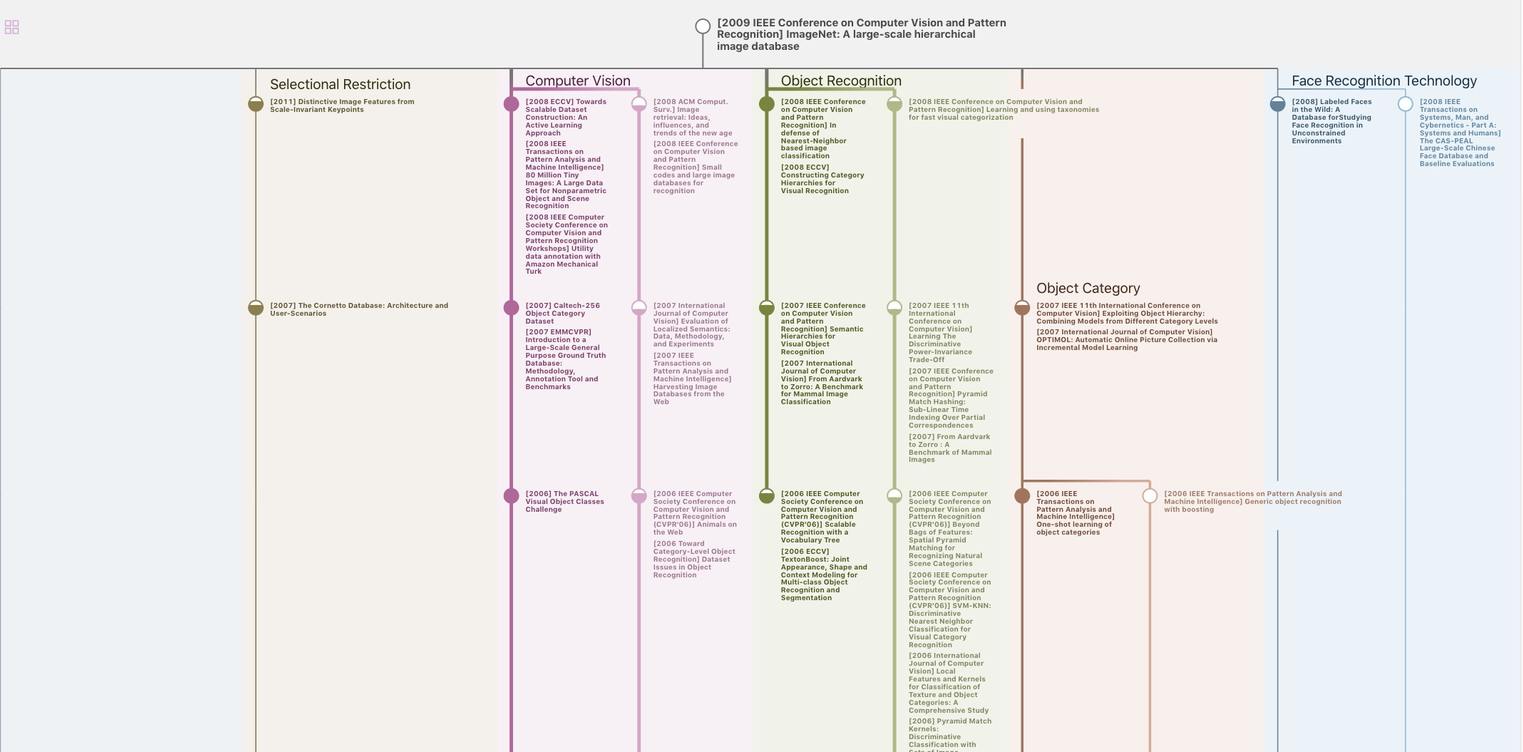
生成溯源树,研究论文发展脉络
Chat Paper
正在生成论文摘要