Unveiling Details in the Dark: Simultaneous Brightening and Zooming for Low-Light Image Enhancement
AAAI 2024(2024)
摘要
Existing super-resolution methods exhibit limitations when applied to nighttime scenes, primarily due to their lack of adaptation to low-pair dynamic range and noise-heavy dark-light images. In response, this research introduces an innovative customized framework to simultaneously Brighten and Zoom in low-resolution images captured in low-light conditions, dubbed BrZoNet. The core method begins by feeding low-light, low-resolution images, and their corresponding ground truths into the Retinex-induced siamese decoupling network. This process yields distinct reflectance maps and illuminance maps, guided by supervision from the ground truth’s decomposition maps. Subsequently, these reflectance and illuminance maps transition into an intricate super-resolution sub-network. This sub-network employs a meticulously designed cross-layer content-aware interactor - Illumination-aware Interaction Unit(IaIU), elegantly endowed with a gating mechanism. The IaIU facilitates meaningful feature interaction between illuminance and reflectance features while effectively reducing unwanted noise. An intricate super-resolution cage is also constructed to comprehensively integrate information, ultimately resulting in the generation of high-resolution images featuring intricate details. Thorough and diverse experiments validate the superiority of the proposed BrZoNet, surpassing contemporary cutting-edge technologies by proficiently augmenting brightness and intricately recovering complex details, showcasing advancements of 7.1% in PSNR, 2.4% in SSIM, and an impressive 36.8% in LPIPS metrics.
更多查看译文
关键词
CV: Low Level & Physics-based Vision
AI 理解论文
溯源树
样例
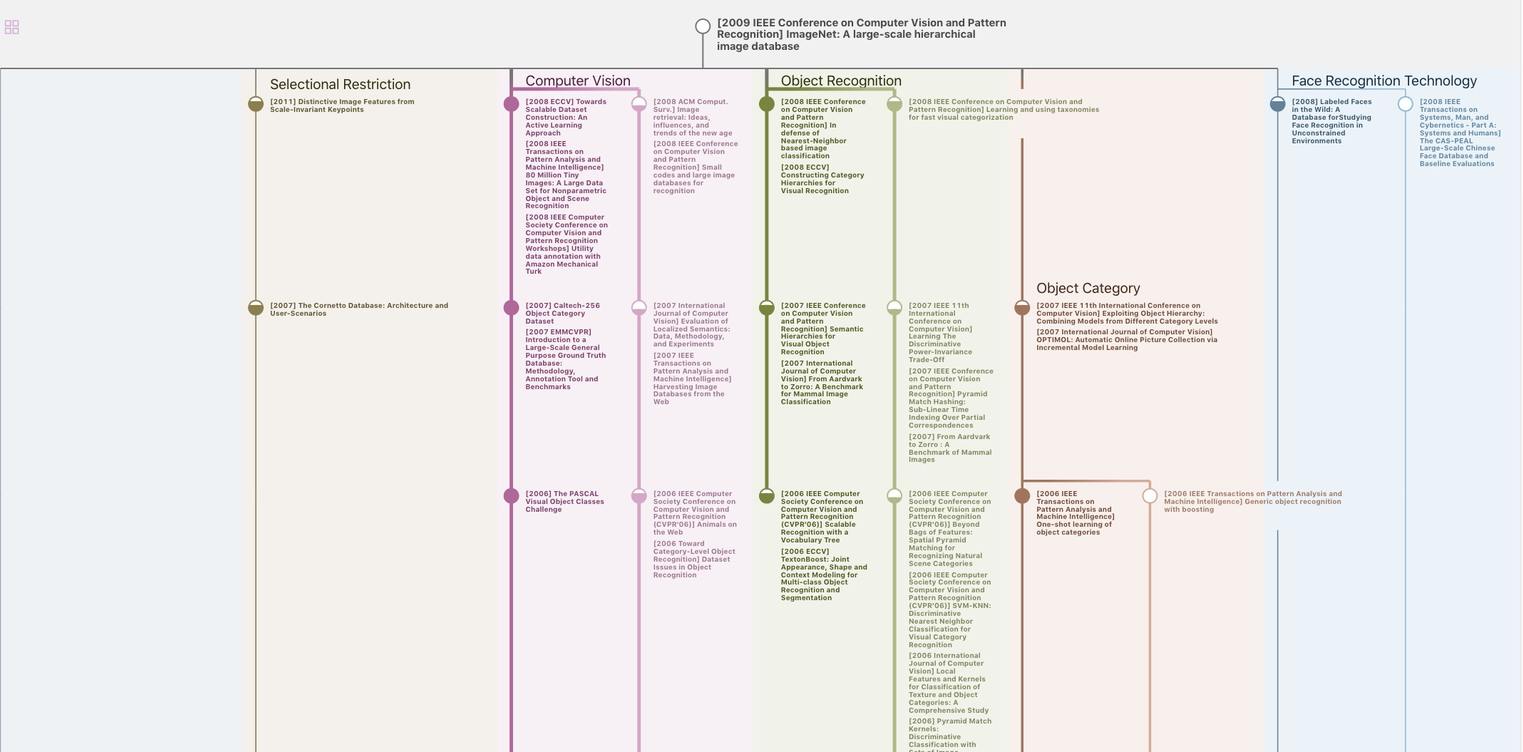
生成溯源树,研究论文发展脉络
Chat Paper
正在生成论文摘要