Compact HD Map Construction via Douglas-Peucker Point Transformer
AAAI 2024(2024)
摘要
High-definition (HD) map construction requires a comprehensive understanding of traffic environments, encompassing centimeter-level localization and rich semantic information. Previous works face challenges in redundant point representation or high-complexity curve modeling. In this paper, we present a flexible yet effective map element detector that synthesizes hierarchical information with a compact Douglas-Peucker (DP) point representation in a transformer architecture for robust and reliable predictions. Specifically, our proposed representation approximates class-agnostic map elements with DP points, which are sparsely located in crucial positions of structures and can get rid of redundancy and complexity. Besides, we design a position constraint with uncertainty to avoid potential ambiguities. Moreover, pairwise-point shape matching constraints are proposed to balance local structural information of different scales. Experiments on the public nuScenes dataset demonstrate that our method overwhelms current SOTAs. Extensive ablation studies validate each component of our methods. Codes will be released at https://github.com/sweety121/DPFormer.
更多查看译文
关键词
CV: Vision for Robotics & Autonomous Driving,CV: Object Detection & Categorization
AI 理解论文
溯源树
样例
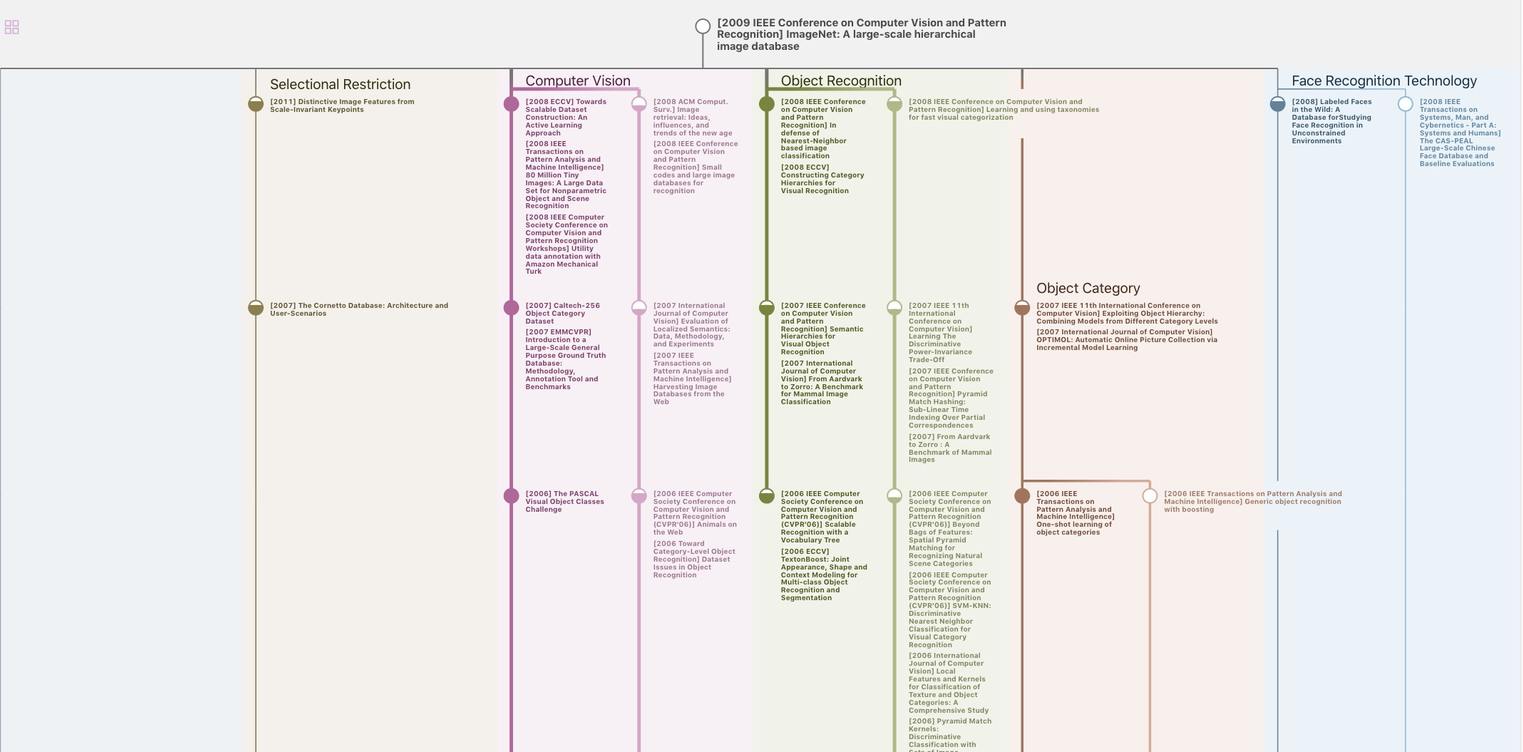
生成溯源树,研究论文发展脉络
Chat Paper
正在生成论文摘要