Beyond Words: A Heterogeneous Graph Representation of Text via Graph Neural Networks For Classification
2024 20th CSI International Symposium on Artificial Intelligence and Signal Processing (AISP)(2024)
摘要
In this research, a method employing Graph Neural Networks (GNNs) for text classification is introduced. Our approach transforms unprocessed raw text into structured heterogeneous graphs, a format known for its remarkable propensity to understand complex data relations. The conversion process enables the GNN, a model distinguished for its effectiveness in multiple domains, to comprehensively interpret and deliver a textured representation of the original text. By creating a graph per document and capturing both overt and covert contextual details within the texts, the text classification task is converted to a graph classification task. This approach can convert texts into graphs regardless of their length which eliminates the need to set a maximum length or add padding to texts that are shorter than the maximum length when inputting them into neural networks. To show the versatility and robustness of the method, experimental evaluations are conducted on two datasets – the Yelp Polarity for sentiment analysis binary classification and AG news for multi-class text classification. These experiments also serve to illustrate the efficacy of using dependencies and tags to trace and map relationships within word sequences such that the model’s context perception will be enhanced. In comparison with established baselines, the advanced GNN-based procedure manifests superior capacity in text representation. Hence, this study paves the way toward a new avenue in text classification specially sentiment analysis meanwhile underscores the eminent role of graph neural networks in reinforcing context understanding and text representation.
1
1
Code available at: Beyond-Words
更多查看译文
关键词
Graph Neural Network,Heterogeneous Graph,Sentiment Analysis,Text Classification
AI 理解论文
溯源树
样例
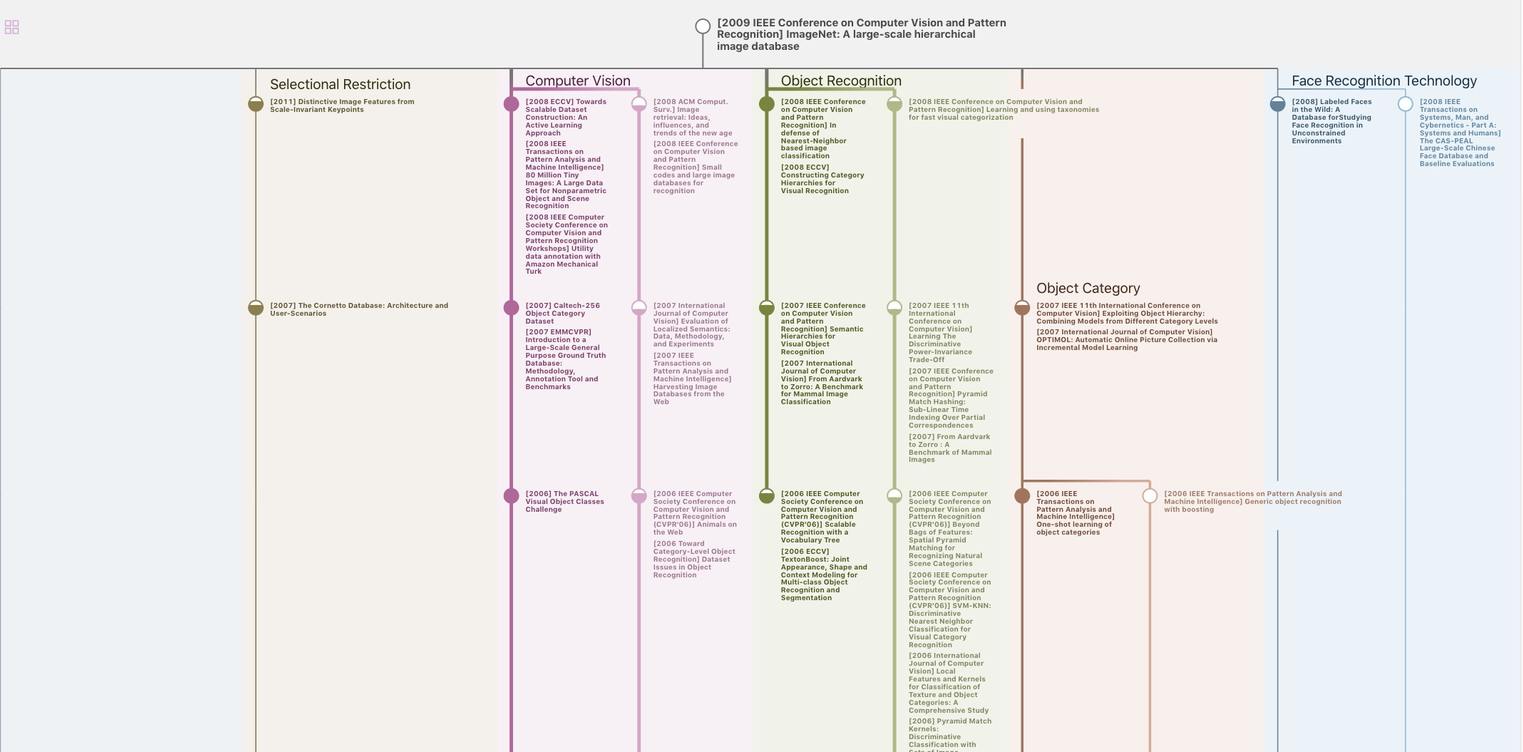
生成溯源树,研究论文发展脉络
Chat Paper
正在生成论文摘要