FL-NAS: Towards Fairness of NAS for Resource Constrained Devices via Large Language Models : (Invited Paper)
2024 29th Asia and South Pacific Design Automation Conference (ASP-DAC)(2024)
摘要
Neural Architecture Search (NAS) has become the de fecto tools in the industry in automating the design of deep neural networks for various applications, especially those driven by mobile and edge devices with limited computing resources. The emerging large language models (LLMs), due to their prowess, have also been incorporated into NAS recently and show some promising results. This paper conducts further exploration in this direction by considering three important design metrics simultaneously, i.e., model accuracy, fairness, and hardware deployment efficiency. We propose a novel LLM-based NAS framework, FL-NAS, in this paper, and show experimentally that FL-NAS can indeed find high-performing DNNs, beating state-of-the-art DNN models by orders-of-magnitude across almost all design considerations.
更多查看译文
关键词
neural architecture search,hardware efficiency,large language model,fairness
AI 理解论文
溯源树
样例
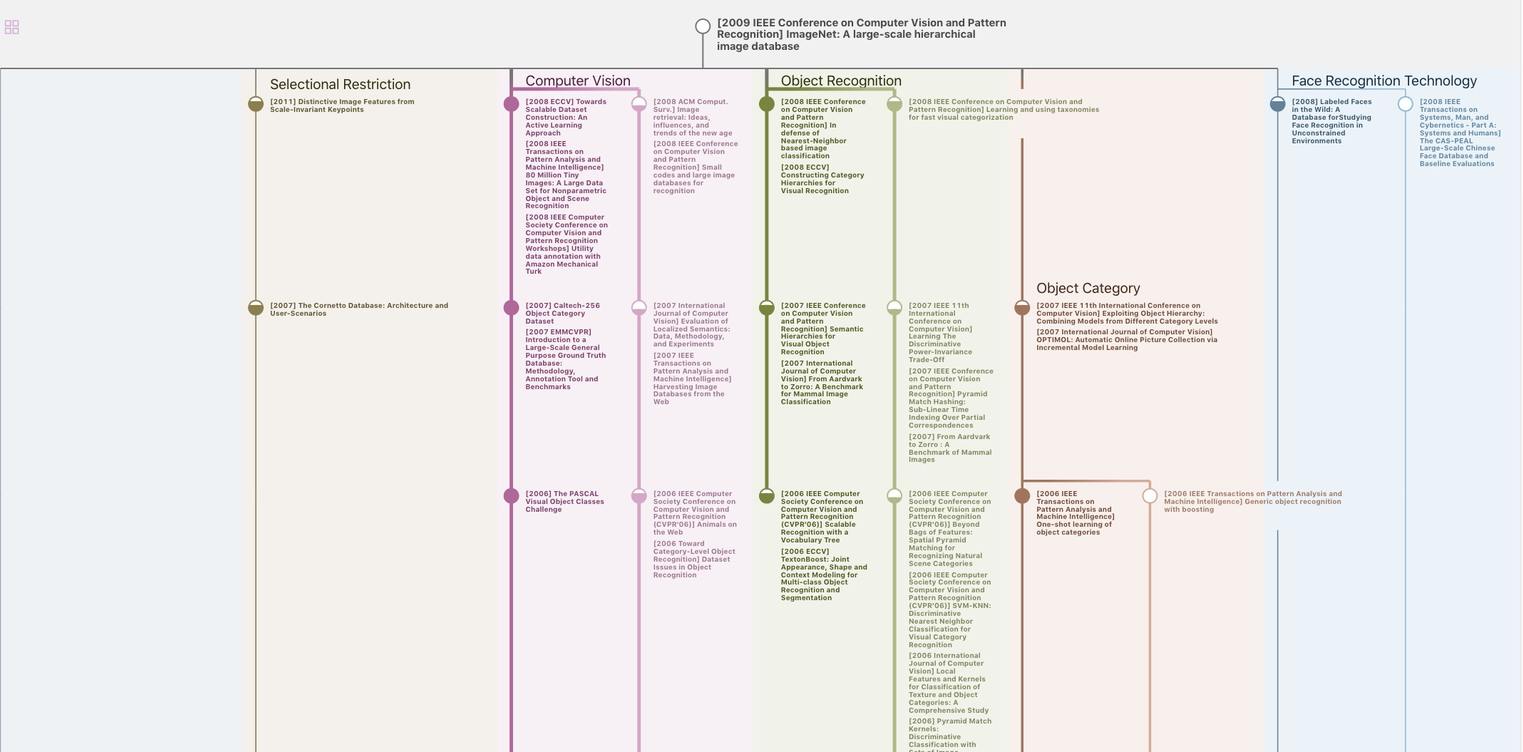
生成溯源树,研究论文发展脉络
Chat Paper
正在生成论文摘要