Hyneter:Hybrid Network Transformer for Multiple Computer Vision Tasks
IEEE Transactions on Industrial Informatics(2024)
摘要
In this article, we point out that the essential differences between convolutional neural network (CNN)-based and transformer-based detectors, which cause worse performance of small object in transformer-based methods, are the gap between local information and global dependencies in feature extraction and propagation. To address these differences, we propose a new vision transformer, called Hybrid Network Transformer (Hyneter), after preexperiments that indicate the gap causes CNN-based and transformer-based methods to increase size-different objects results unevenly. Different from the divide-and-conquer strategy in previous methods, Hyneters consist of hybrid network backbone (HNB) and dual switching (DS) module, which integrate local information and global dependencies, and transfer them simultaneously. Based on the balance strategy, HNB extends the range of local information by embedding convolution layers into transformer blocks in parallel, and DS adjusts excessive reliance on global dependencies outside the patch. Ablation studies illustrate that Hyneters achieve the state-of-the-art performance by a large margin of
$+2.1\sim 13.2 \text{AP}$
on COCO, and
$+3.1\sim 6.5 \text{mIoU}$
on VisDrone with lighter model size and lower computational cost in object detection. Furthermore, Hyneters achieve the state-of-the-art results on multiple computer vision tasks, such as object detection (
$60.1 \text{AP}$
on COCO and
$46.1 \text{AP}$
on VisDrone), semantic segmentation (
$54.3 \text{AP}$
on ADE20K), and instance segmentation (
$48.5 \text{AP}^{\text{mask}}$
on COCO), and surpass previous best methods. The code will be publicly available later.
更多查看译文
关键词
Convolutional neural network (CNN),hybrid network,object detection,transformer
AI 理解论文
溯源树
样例
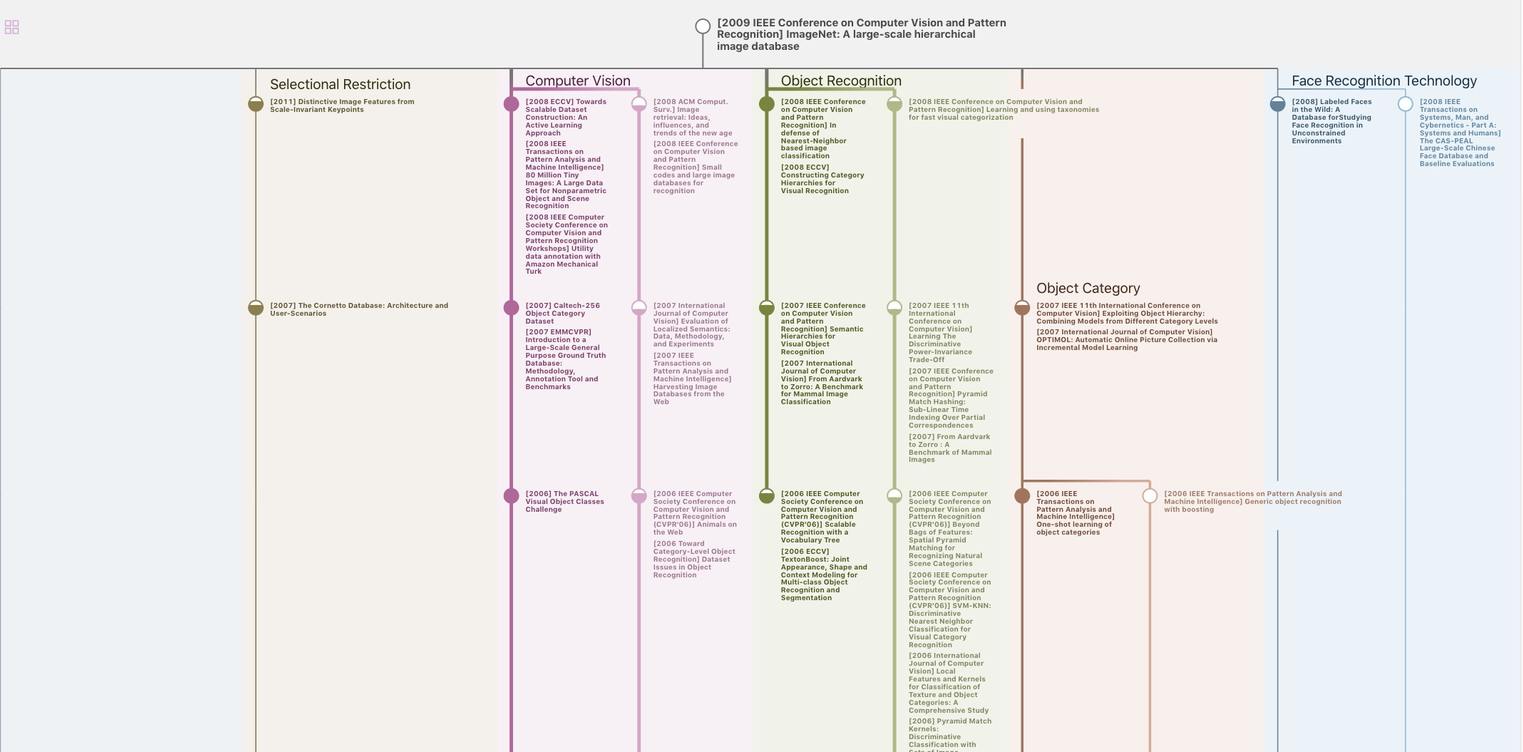
生成溯源树,研究论文发展脉络
Chat Paper
正在生成论文摘要