Advanced deep learning framework for underwater object detection with multibeam forward-looking sonar
STRUCTURAL HEALTH MONITORING-AN INTERNATIONAL JOURNAL(2024)
摘要
Underwater object detection (UOD) is an essential activity in maintaining and monitoring underwater infrastructure, playing an important role in their efficient and low-risk asset management. In underwater environments, sonar, recognized for overcoming the limitations of optical imaging in low-light and turbid conditions, has increasingly gained popularity for UOD. However, due to the low resolution and limited foreground-background contrast in sonar images, existing sonar-based object detection algorithms still face challenges regarding precision and transferability. To solve these challenges, this article proposes an advanced deep learning framework for UOD that uses the data from multibeam forward-looking sonar. The framework is adapted from the network architecture of YOLOv7, one of the state-of-the-art vision-based object detection algorithms, by incorporating unique optimizations in three key aspects: data preprocessing, feature fusion, and loss functions. These improvements are extensively tested on a dedicated public dataset, showing superior object classification performance compared to the selected existing sonar-based methods. Through experiments conducted on an underwater remotely operated vehicle, the proposed framework validates significant enhancements in target classification, localization, and transfer learning capabilities. Since the engineering structures have similar geometric shapes to the objects tested in this study, the proposed framework presents potential applicability to underwater structural inspection and monitoring, and autonomous asset management.
更多查看译文
关键词
Underwater infrastructure inspection,underwater object detection,multibeam forward-looking sonar imaging,deep learning,transfer learning,remotely operated vehicle,structural health monitoring
AI 理解论文
溯源树
样例
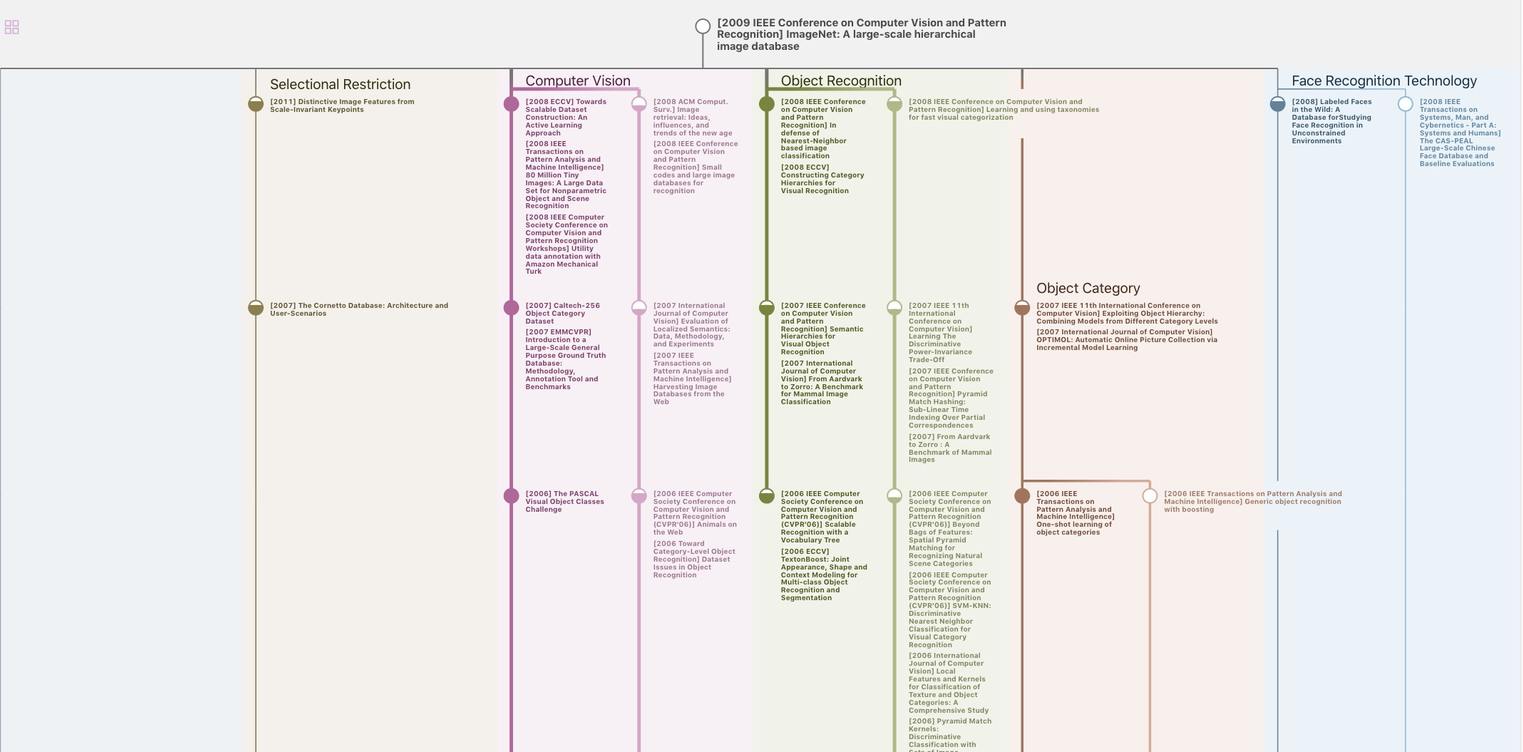
生成溯源树,研究论文发展脉络
Chat Paper
正在生成论文摘要