Block Image Compressive Sensing with Local and Global Information Interaction
AAAI 2024(2024)
摘要
Block image compressive sensing methods, which divide a single image into small blocks for efficient sampling and reconstruction, have achieved significant success.
However, these methods process each block locally and thus disregard the global communication among different blocks in the reconstruction step.
Existing methods have attempted to address this issue with local filters or by directly reconstructing the entire image, but they have only achieved insufficient communication among adjacent pixels or bypassed the problem.
To directly confront the communication problem among blocks and effectively resolve it, we propose a novel approach called Block Reconstruction with Blocks' Communication Network (BRBCN).
BRBCN focuses on both local and global information, while further taking their interactions into account.
Specifically, BRBCN comprises dual CNN and Transformer architectures, in which CNN is used to reconstruct each block for powerful local processing and Transformer is used to calculate the global communication among all the blocks.
Moreover, we propose a global-to-local module (G2L) and a local-to-global module (L2G) to effectively integrate the representations of CNN and Transformer, with which our BRBCN network realizes the bidirectional interaction between local and global information.
Extensive experiments show our BRBCN method outperforms existing state-of-the-art methods by a large margin.
The code is available at https://github.com/kongxiuxiu/BRBCN
更多查看译文
关键词
CV: Low Level & Physics-based Vision,CV: Applications
AI 理解论文
溯源树
样例
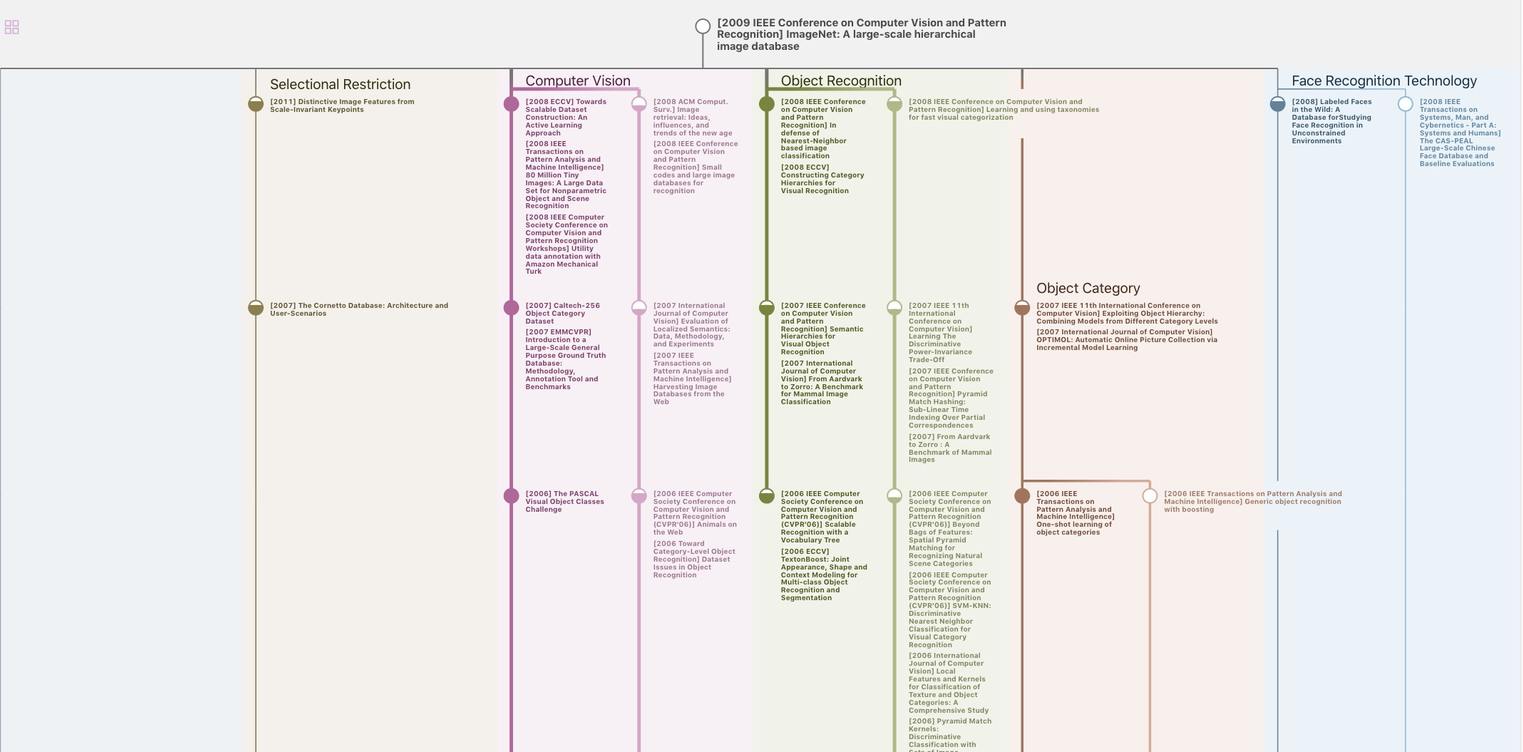
生成溯源树,研究论文发展脉络
Chat Paper
正在生成论文摘要