Transformer-Based Video-Structure Multi-Instance Learning for Whole Slide Image Classification
AAAI 2024(2024)
摘要
Pathological images play a vital role in clinical cancer diagnosis. Computer-aided diagnosis utilized on digital Whole Slide Images (WSIs) has been widely studied. The major challenge of using deep learning models for WSI analysis is the huge size of WSI images and existing methods struggle between end-to-end learning and proper modeling of contextual information. Most state-of-the-art methods utilize a two-stage strategy, in which they use a pre-trained model to extract features of small patches cut from a WSI and then input these features into a classification model. These methods can not perform end-to-end learning and consider contextual information at the same time. To solve this problem, we propose a framework that models a WSI as a pathologist's observing video and utilizes Transformer to process video clips with a divide-and-conquer strategy, which helps achieve both context-awareness and end-to-end learning. Extensive experiments on three public WSI datasets show that our proposed method outperforms existing SOTA methods in both WSI classification and positive region detection.
更多查看译文
关键词
ML: Multi-instance/Multi-view Learning,CV: Medical and Biological Imaging
AI 理解论文
溯源树
样例
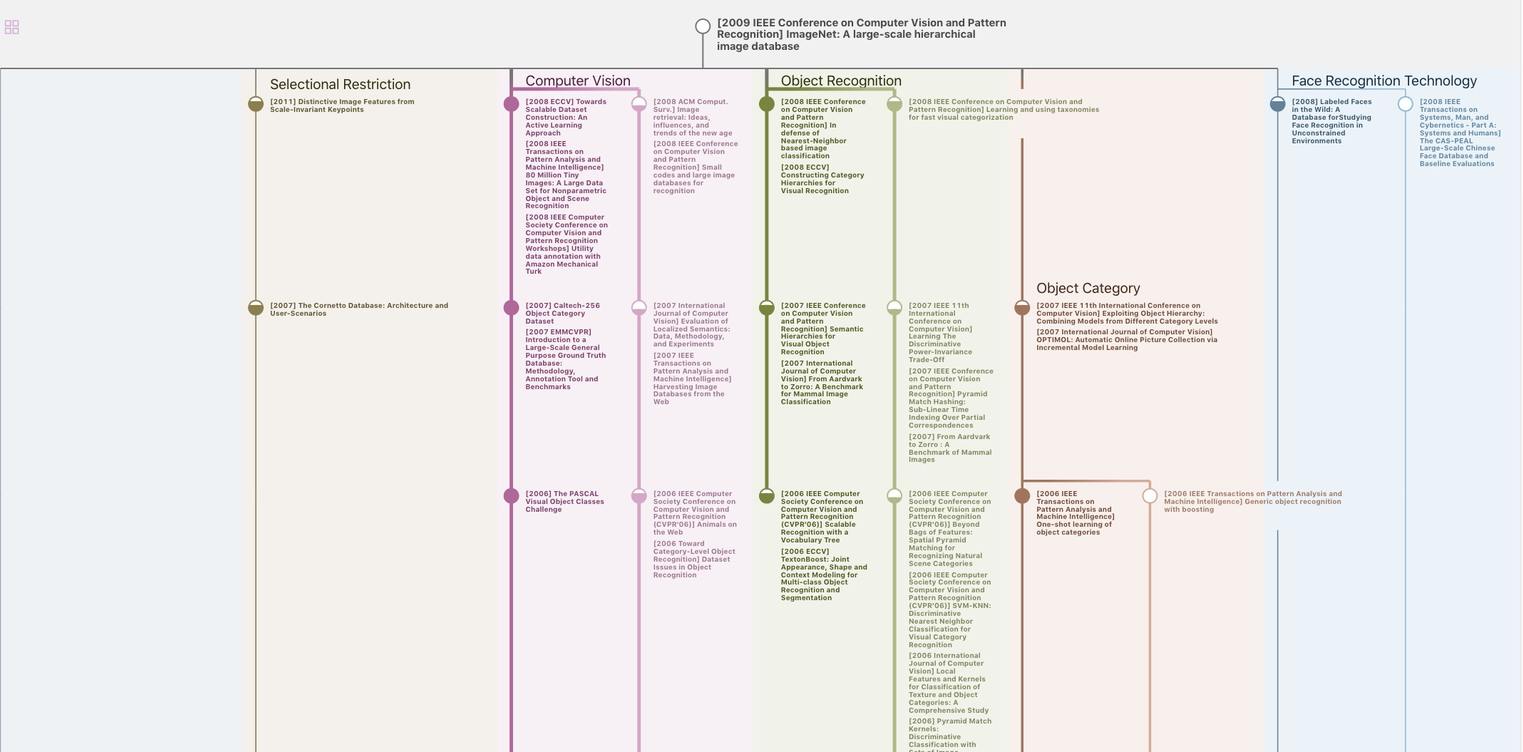
生成溯源树,研究论文发展脉络
Chat Paper
正在生成论文摘要