An Efficient Subgraph-Inferring Framework for Large-Scale Heterogeneous Graphs
AAAI 2024(2024)
摘要
Heterogeneous Graph Neural Networks (HGNNs) play a vital role in advancing the field of graph representation learning by addressing the complexities arising from diverse data types and interconnected relationships in real-world scenarios. However, traditional HGNNs face challenges when applied to large-scale graphs due to the necessity of training or inferring on the entire graph. As the size of the heterogeneous graphs increases, the time and memory overhead required by these models escalates rapidly, even reaching unacceptable levels. To address this issue, in this paper, we present a novel framework named (SubInfer), which conducts training and inferring on subgraphs instead of the entire graphs, hence efficiently handling large-scale heterogeneous graphs. The proposed framework comprises three main steps: 1) partitioning the heterogeneous graph from multiple perspectives to preserve various semantic information, 2) completing the subgraphs to improve the convergence speed of subgraph training and the performance of subgraph inference, and 3) training and inferring the HGNN model on distributed clusters to further reduce the time overhead. The framework is applicable to the vast majority of HGNN models. Experiments on five benchmark datasets demonstrate that SubInfer effectively optimizes the training and inference phase, delivering comparable performance to traditional HGNN models while significantly reducing time and memory overhead.
更多查看译文
关键词
DMKM: Graph Mining, Social Network Analysis & Community,ML: Graph-based Machine Learning
AI 理解论文
溯源树
样例
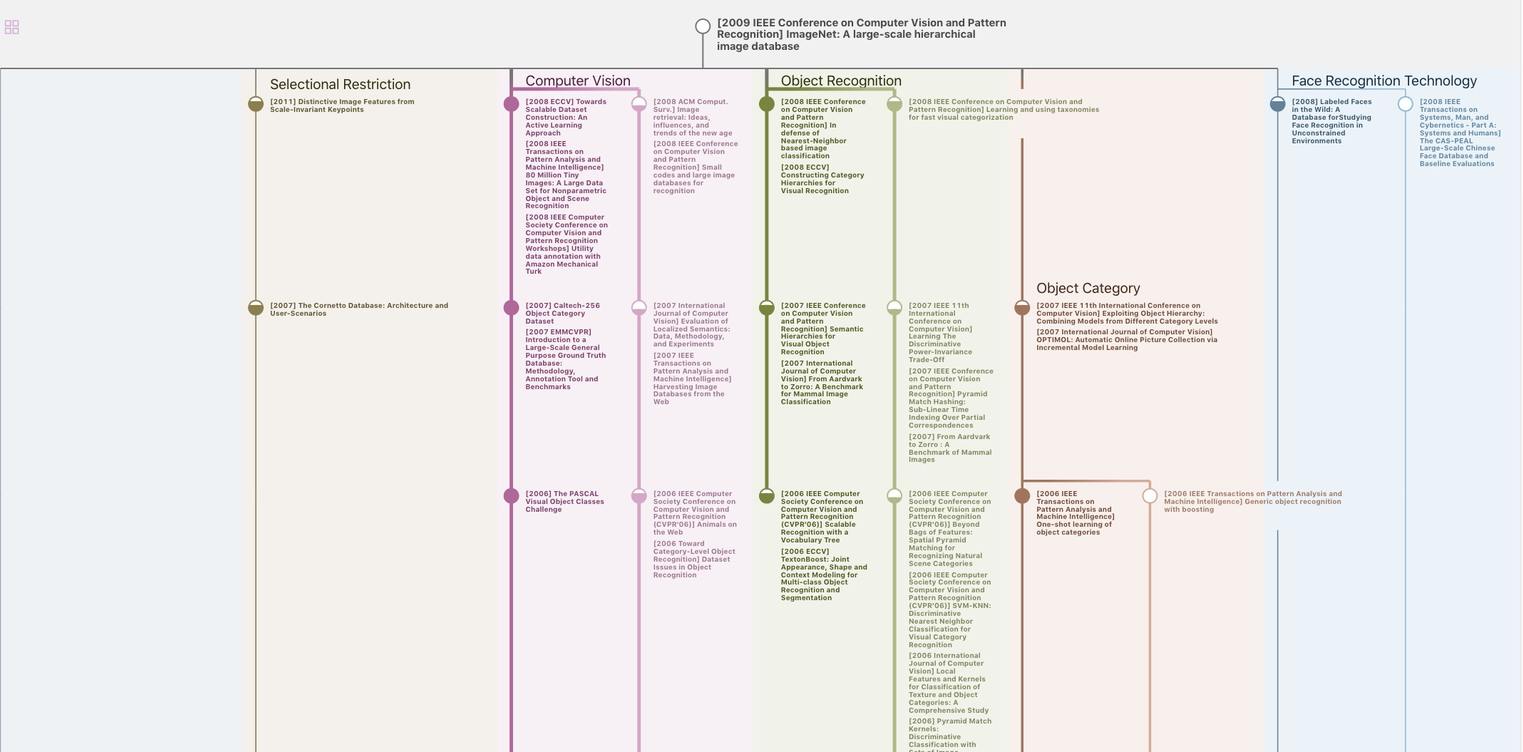
生成溯源树,研究论文发展脉络
Chat Paper
正在生成论文摘要