On Exploiting Network Topology for Hierarchical Coded Multi-task Learning
IEEE Transactions on Communications(2024)
摘要
Distributed multi-task learning (MTL) is a learning paradigm where distributed users simultaneously learn multiple tasks by leveraging the correlations among tasks. However, distributed MTL suffers from a more severe communication bottleneck than single-task learning as more than one models need to be transmitted in the communication phase. To address this issue, we investigate the hierarchical MTL system where distributed users wish to jointly learn different learning models orchestrated by a central server with the help of multiple relays. We propose a coded distributed computing scheme for hierarchical MTL systems that jointly exploits the network topology and relays’ computing capability to create coded multicast opportunities to improve communication efficiency. We theoretically prove that the proposed scheme can significantly reduce the communication loads both in the uplink and downlink transmissions between relays and the server. To further illustrate the optimality of the proposed scheme, we derive information-theoretic lower bounds on the minimum uplink and downlink communication loads and prove that the gaps between achievable upper bounds and lower bounds are within the minimum number of connected users among all relays. In particular, when the network topology can be delicately designed, the proposed scheme can achieve the information-theoretic optimal communication loads. Experiments on real-world datasets show that our proposed scheme can greatly reduce the overall training time compared to the conventional hierarchical MTL scheme.
更多查看译文
关键词
Multi-task learning,coding techniques,distributed learning,hierarchical systems,communication load
AI 理解论文
溯源树
样例
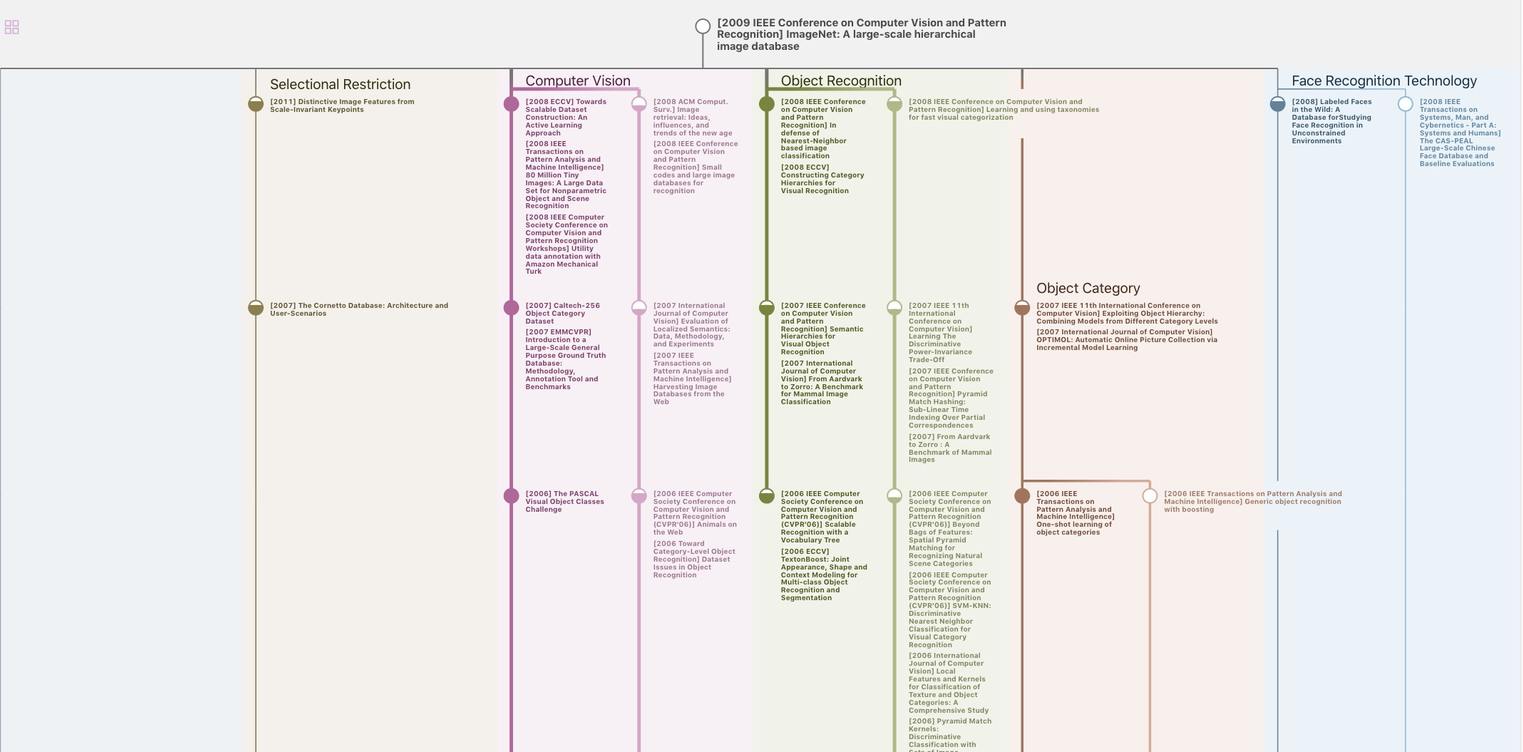
生成溯源树,研究论文发展脉络
Chat Paper
正在生成论文摘要