A Task-Oriented Grasping Framework Guided by Visual Semantics for Mobile Manipulators.
IEEE Trans. Instrum. Meas.(2024)
摘要
The densely cluttered operational environment and the absence of object information hinder mobile manipulators from achieving specific grasping tasks. To address this issue, this paper proposes a task-oriented grasping framework guided by visual semantics for mobile manipulators. With multiple attention mechanisms, we first present a modified DeepLabV3+ model by replacing the backbone networks with Mobilenetv2 and incorporating a novel attention feature fusion module to build a preprocessing module, thus producing semantic images efficiently and accurately. A semantic-guided viewpoint adjustment strategy is designed in which the semantic images are used to calculate the optimal viewpoint that enables the eye-in-hand installed camera to self-adjust until it encompasses all the objects within the task-related area. Based on the improved DeepLabV3+ model and the generative residual convolutional neural network, a task-oriented grasp detection structure is developed to generate a more precise grasp representation for the specific object in densely cluttered scenarios. The effectiveness of the proposed framework is validated through the dataset comparison tests and multiple sets of practical grasping experiments. The results demonstrate that our proposed method achieves competitive results versus the state-of-art methods, which attains an accuracy of 98.3% on the Cornell grasping dataset and achieves a grasping success rate of 91% in densely cluttered scenes.
更多查看译文
关键词
Task-oriented robotic grasping,visual semantics,absence of object information,deep learning,mobile manipulator
AI 理解论文
溯源树
样例
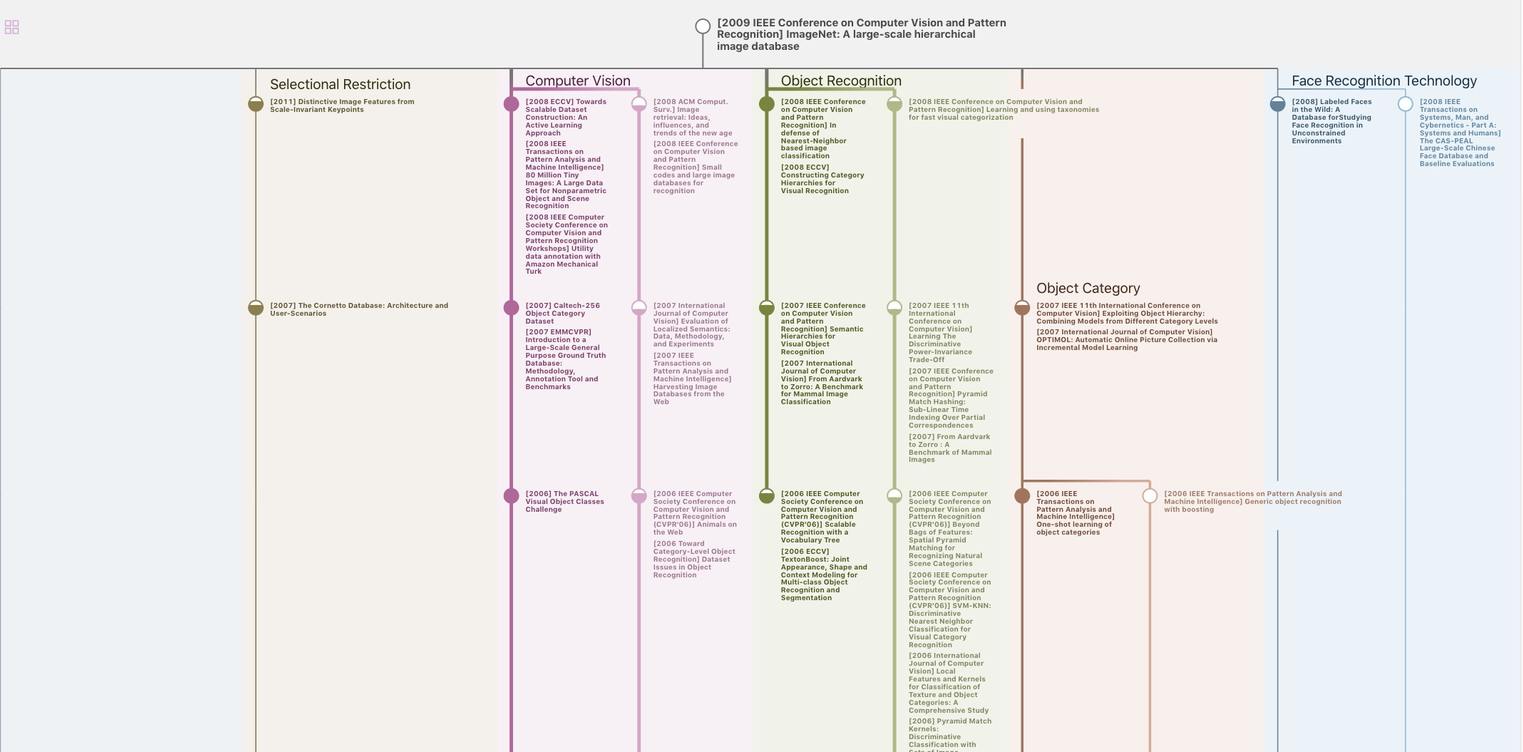
生成溯源树,研究论文发展脉络
Chat Paper
正在生成论文摘要