Deletion-Robust Submodular Maximization under a Knapsack Constraint
IEEE International Conference on High Performance Computing and Communications (HPCC)(2023)CCF C
Abstract
In recent years, as one of the important problems in machine learning optimization theory, submodular maximization has received extensive research attention. Due to the property of diminishing returns of submodular function, many natural problems can be cast to the submodular maximization problem and that can be addressed relatively efficiently. In this paper, we investigate the deletion robust sub modular maximization problem under the knapsack constraint. The robustness means the algorithm can guarantee the quality of its output even after an adversary deleted some elements from the ground set $V$ . During the period when $V$ is accessed, the algorithm does not know the elements the adversary deleted. We consider the scenario where the adversary deletes elements randomly, which is consistent with unexpected events in nature. For offline and streaming scenarios, we present two algorithms that achieve an approximation ratio of $(\frac{1}{4} - \epsilon)$ in expectation and a summary size of $O (k+ \frac{d\log^{2}B}{\epsilon^{3}})$ and $O(\frac{k\log B}{\epsilon}+\frac{d\log^{3} B}{\epsilon^{4}})$ respectively, where $k$ represents the maximum element number of any feasible solution with budget $B$ and $d$ is the maximum number of deleted elements. We conduct experiments on real-world applications to evaluate our proposed algorithms and the experimental results confirm the superiority of our proposed algorithms.
MoreTranslated text
Key words
Submodular maximization,approximation algorithms,robust algorithms,optimization algorithms,machine learning
求助PDF
上传PDF
View via Publisher
AI Read Science
AI Summary
AI Summary is the key point extracted automatically understanding the full text of the paper, including the background, methods, results, conclusions, icons and other key content, so that you can get the outline of the paper at a glance.
Example
Background
Key content
Introduction
Methods
Results
Related work
Fund
Key content
- Pretraining has recently greatly promoted the development of natural language processing (NLP)
- We show that M6 outperforms the baselines in multimodal downstream tasks, and the large M6 with 10 parameters can reach a better performance
- We propose a method called M6 that is able to process information of multiple modalities and perform both single-modal and cross-modal understanding and generation
- The model is scaled to large model with 10 billion parameters with sophisticated deployment, and the 10 -parameter M6-large is the largest pretrained model in Chinese
- Experimental results show that our proposed M6 outperforms the baseline in a number of downstream tasks concerning both single modality and multiple modalities We will continue the pretraining of extremely large models by increasing data to explore the limit of its performance
Upload PDF to Generate Summary
Must-Reading Tree
Example
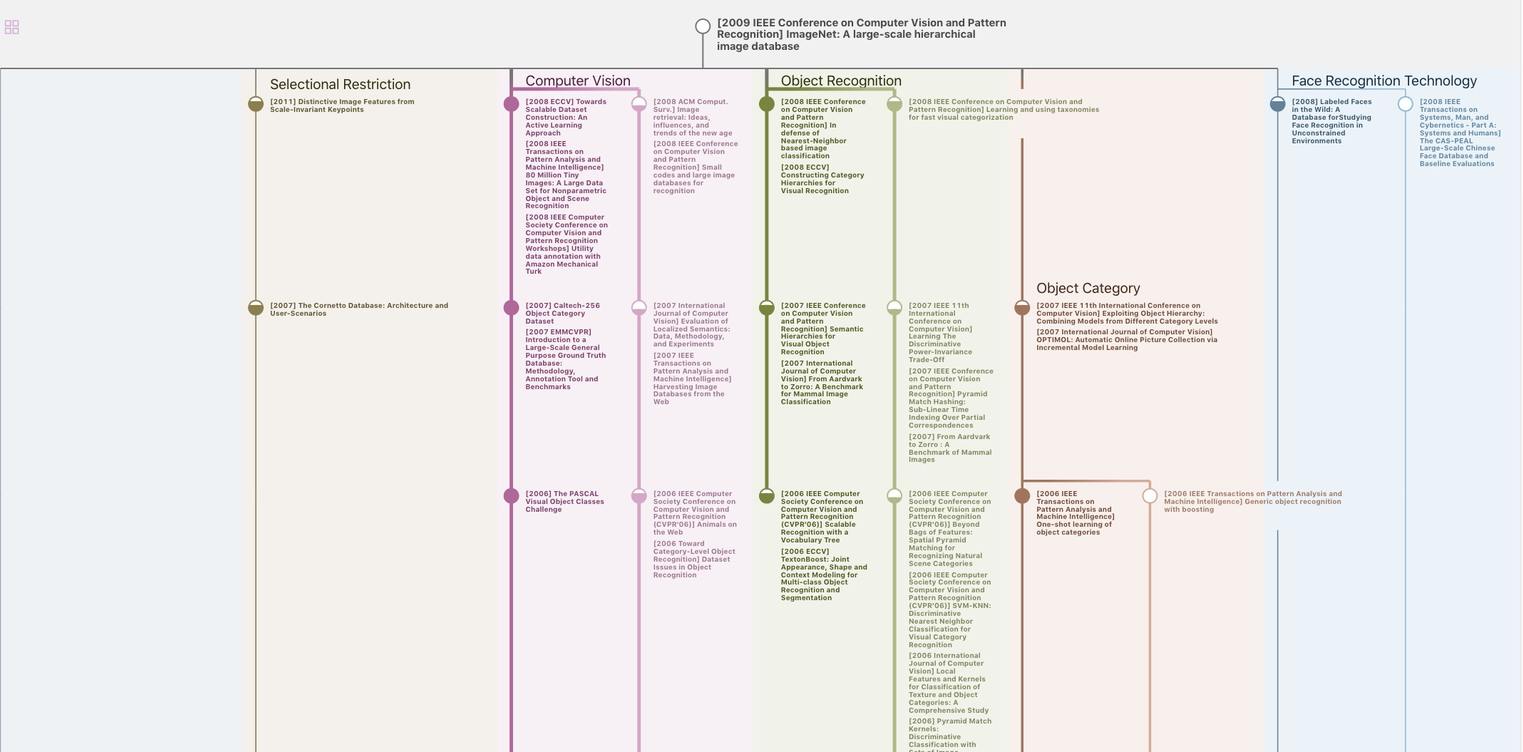
Generate MRT to find the research sequence of this paper
Data Disclaimer
The page data are from open Internet sources, cooperative publishers and automatic analysis results through AI technology. We do not make any commitments and guarantees for the validity, accuracy, correctness, reliability, completeness and timeliness of the page data. If you have any questions, please contact us by email: report@aminer.cn
Chat Paper
GPU is busy, summary generation fails
Rerequest