From Retrieval to Generation: A Simple and Unified Generative Model for End-to-End Task-Oriented Dialogue
AAAI 2024(2024)
摘要
Retrieving appropriate records from the external knowledge base to generate informative responses is the core capability of end-to-end task-oriented dialogue systems (EToDs). Most of the existing methods additionally train the retrieval model or use the memory network to retrieve the knowledge base, which decouples the knowledge retrieval task from the response generation task, making it difficult to jointly optimize and failing to capture the internal relationship between the two tasks. In this paper, we propose a simple and unified generative model for task-oriented dialogue systems, which recasts the EToDs task as a single sequence generation task and uses maximum likelihood training to train the two tasks in a unified manner. To prevent the generation of non-existent records, we design the prefix trie to constrain the model generation, which ensures consistency between the generated records and the existing records in the knowledge base. Experimental results on three public benchmark datasets demonstrate that our method achieves robust performance on generating system responses and outperforms the baseline systems. To facilitate future research in this area, the code is available at https://github.com/dzy1011/Uni-ToD.
更多查看译文
关键词
NLP: Conversational AI/Dialog Systems,NLP: Question Answering
AI 理解论文
溯源树
样例
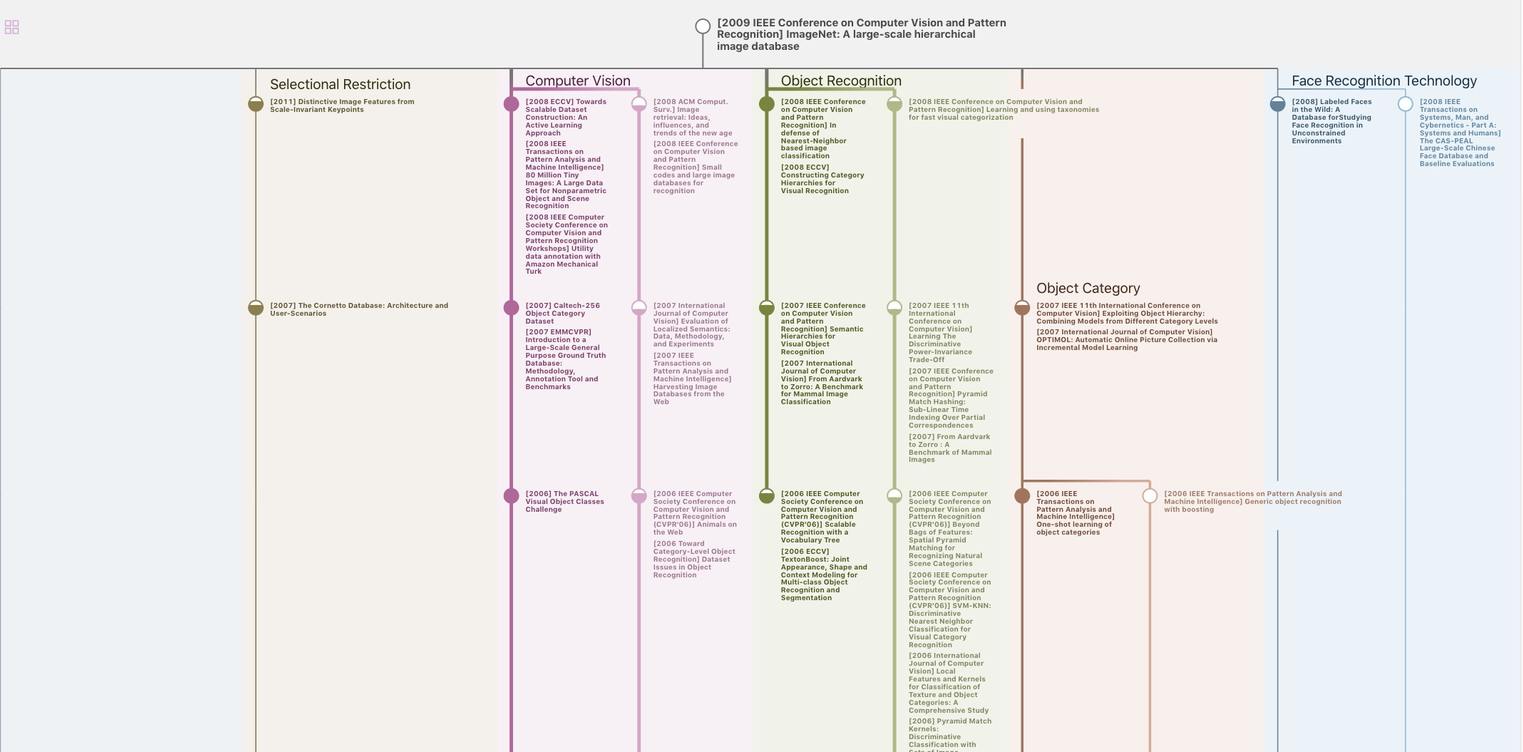
生成溯源树,研究论文发展脉络
Chat Paper
正在生成论文摘要