Residual Hyperbolic Graph Convolution Networks
AAAI 2024(2024)
摘要
Hyperbolic graph convolutional networks (HGCNs) have demonstrated representational capabilities of modeling hierarchical-structured graphs. However, as in general GCNs, over-smoothing may occur as the number of model layers increases, limiting the representation capabilities of most current HGCN models. In this paper, we propose residual hyperbolic graph convolutional networks (R-HGCNs) to address the over-smoothing problem. We introduce a hyperbolic residual connection function to overcome the over-smoothing problem, and also theoretically prove the effectiveness of the hyperbolic residual function. Moreover, we use product manifolds and HyperDrop to facilitate the R-HGCNs. The distinctive features of the R-HGCNs are as follows: (1) The hyperbolic residual connection preserves the initial node information in each layer and adds a hyperbolic identity mapping to prevent node features from being indistinguishable. (2) Product manifolds in R-HGCNs have been set up with different origin points in different components to facilitate the extraction of feature information from a wider range of perspectives, which enhances the representing capability of R-HGCNs. (3) HyperDrop adds multiplicative Gaussian noise into hyperbolic representations, such that perturbations can be added to alleviate the over-fitting problem without deconstructing the hyperbolic geometry.
Experiment results demonstrate the effectiveness of R-HGCNs under various graph convolution layers and different structures of product manifolds.
更多查看译文
关键词
ML: Learning with Manifolds,ML: Deep Learning Algorithms,ML: Optimization
AI 理解论文
溯源树
样例
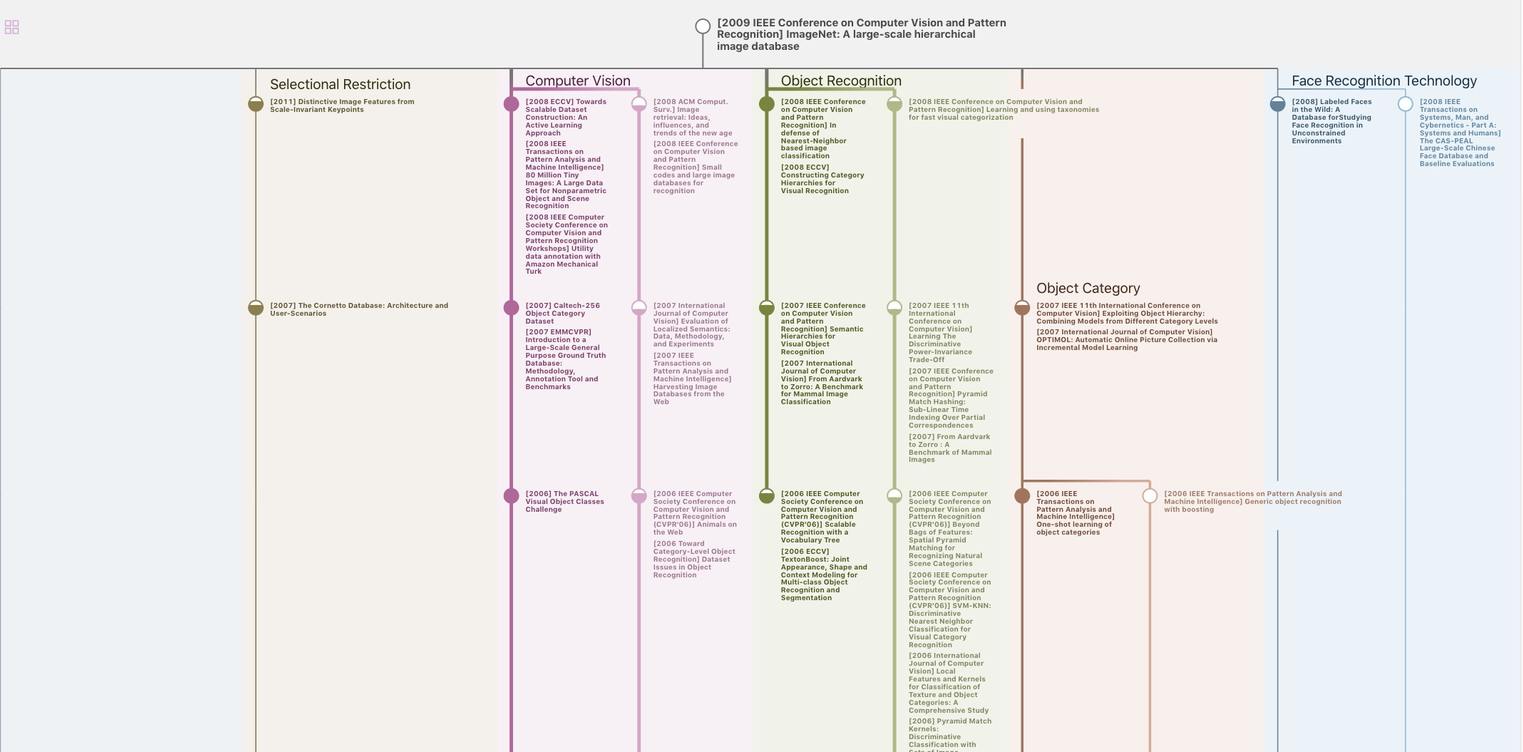
生成溯源树,研究论文发展脉络
Chat Paper
正在生成论文摘要