SOH Based Power Sharing Algorithm for Second-life Batteries Using Health Factors
2024 Third International Conference on Power, Control and Computing Technologies (ICPC2T)(2024)
Electrical Engineering Department
Abstract
The uncertain capacity differences in second-life batteries due to internal characteristics require SOH balancing for SLB modules. The most popular SOC balancing does not guarantee the SOH balance and battery efficiency. Many works of literature try to extract the SOH parameter through either the number of cycles or DOD change. Still, current rate and temperature also have significant influence over the SOH parameter. To solve this problem, in this article the SOH is derived from the most effective health degradation parameters based on DOD and charge rate. An online SOH estimation method is proposed, and according to the determined SOHs, a power-sharing control algorithm is developed to realize the SOH balancing. This article employs the conventional battery energy storage system (BESS) as the second-life battery module since most EVs utilize the bi-directional DC-DC converter boost-buck configuration. MATLAB simulation and floating-point DSP controller hardware-in-loop (CHIL) experimentation results are presented for discharging, DC bus voltage variation and change in power demand cases to verify the proposed algorithm. The algorithm performs decent power-sharing according to their SOH values based on DOD and current rates.
MoreTranslated text
Key words
Battery energy storage system (BESS),Depth of discharge (DOD),State of charge (SOC),State of health (SOH),Lithium-ion (Li-ion)
求助PDF
上传PDF
View via Publisher
AI Read Science
AI Summary
AI Summary is the key point extracted automatically understanding the full text of the paper, including the background, methods, results, conclusions, icons and other key content, so that you can get the outline of the paper at a glance.
Example
Background
Key content
Introduction
Methods
Results
Related work
Fund
Key content
- Pretraining has recently greatly promoted the development of natural language processing (NLP)
- We show that M6 outperforms the baselines in multimodal downstream tasks, and the large M6 with 10 parameters can reach a better performance
- We propose a method called M6 that is able to process information of multiple modalities and perform both single-modal and cross-modal understanding and generation
- The model is scaled to large model with 10 billion parameters with sophisticated deployment, and the 10 -parameter M6-large is the largest pretrained model in Chinese
- Experimental results show that our proposed M6 outperforms the baseline in a number of downstream tasks concerning both single modality and multiple modalities We will continue the pretraining of extremely large models by increasing data to explore the limit of its performance
Upload PDF to Generate Summary
Must-Reading Tree
Example
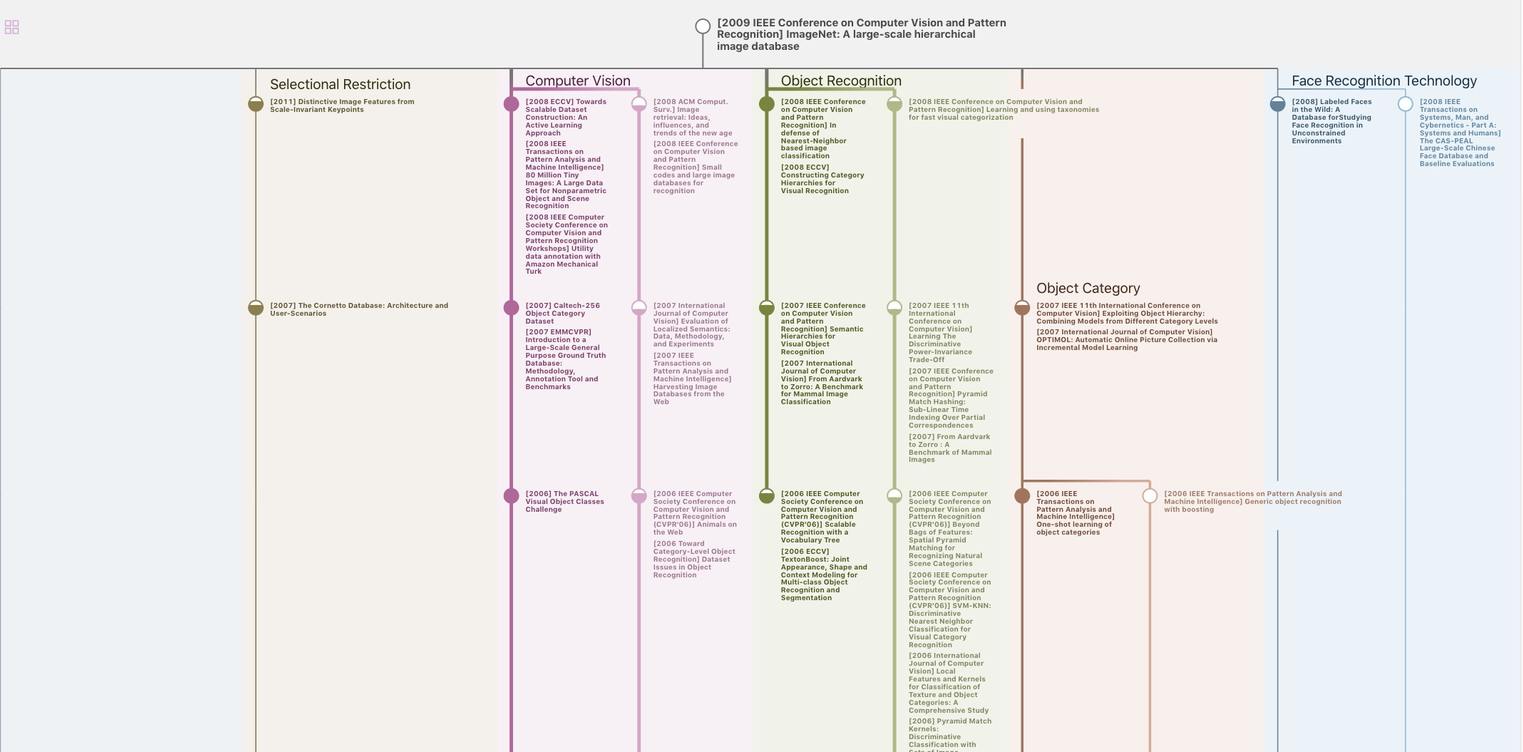
Generate MRT to find the research sequence of this paper
Related Papers
Data Disclaimer
The page data are from open Internet sources, cooperative publishers and automatic analysis results through AI technology. We do not make any commitments and guarantees for the validity, accuracy, correctness, reliability, completeness and timeliness of the page data. If you have any questions, please contact us by email: report@aminer.cn
Chat Paper
GPU is busy, summary generation fails
Rerequest