Detecting Ballistic Motions in Quadruped Robots: A Boosted Tree Motif Classifier for Understanding Reinforcement Learning
2023 SEVENTH IEEE INTERNATIONAL CONFERENCE ON ROBOTIC COMPUTING, IRC 2023(2023)
摘要
Quadrupedal robots require sophisticated algorithms to learn dynamic and ballistic motions, such as jumping. Traditional methods, which often employ Reinforcement Learning (RL), face inherent limitations in identifying the formation of desired actions during training. This study introduces the Boosted Tree Motif Classifier (BTMC), a novel approach designed to accurately detect complex motion patterns, thus facilitating learning dynamic actions in quadrupedal robots. Unlike classical motif search techniques and simple neural networks, which achieved a precision rate of merely 2% and 12%, respectively, in identifying a "jump" motif, BTMC demonstrated remarkable efficiency with a 96% precision rate. The overall accuracy of BTMC was comparable to other learning approaches developed but superior in capturing essential motifs crucial for dynamic motions. The results highlight BTMC's potential as an innovative solution in RL-based robotic systems, offering an advancement in robotic locomotion. Our findings open up new avenues for RL's theoretical and practical applications in robotics.
更多查看译文
关键词
Reinforcement Learning,Dynamic Jumping,Quadrupedal Robots,Model Explainability,Interpretable AI,Motif Analysis,Robot Locomotion
AI 理解论文
溯源树
样例
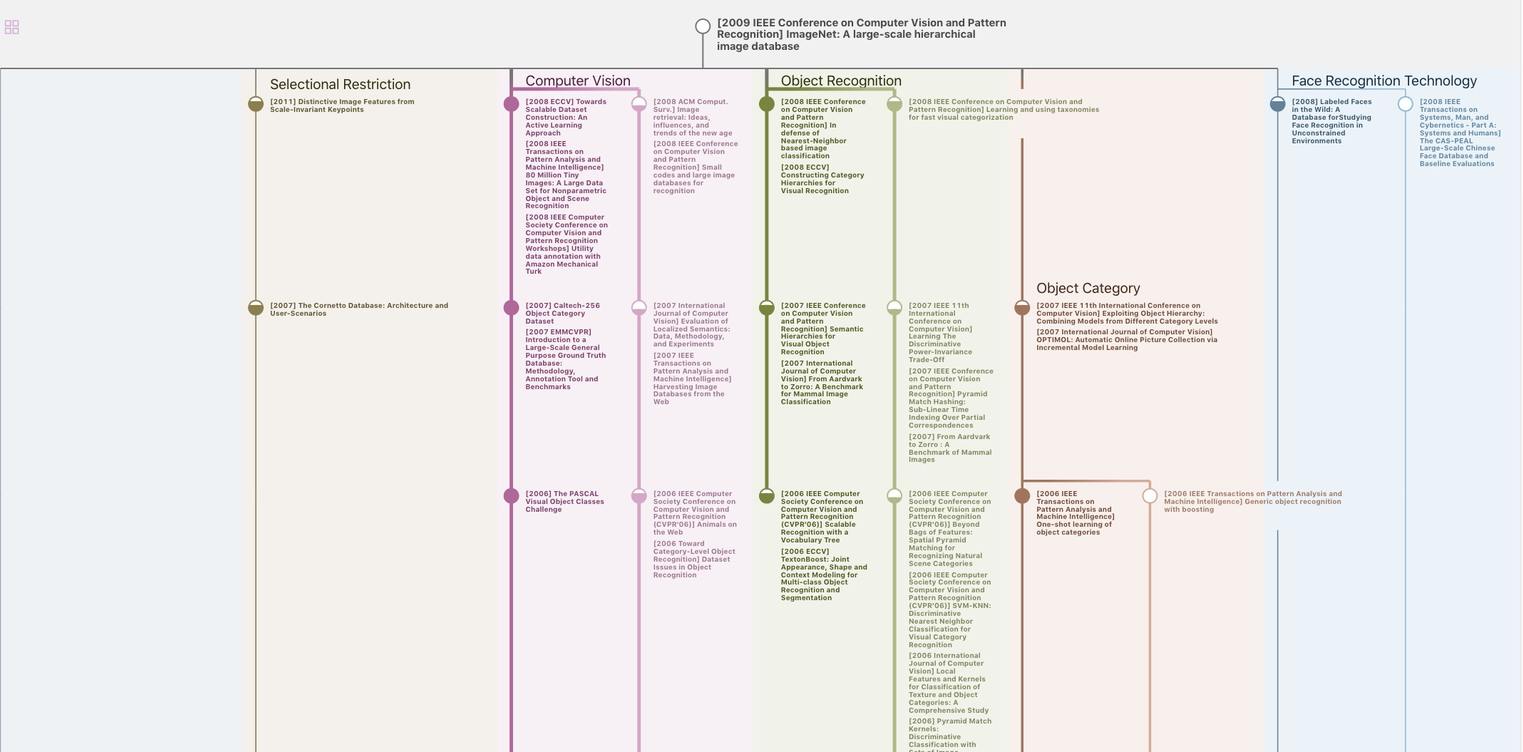
生成溯源树,研究论文发展脉络
Chat Paper
正在生成论文摘要