A Diffusion-Based Pre-training Framework for Crystal Property Prediction
AAAI 2024(2024)
摘要
Many significant problems involving crystal property prediction from 3D structures have limited labeled data due to expensive and time-consuming physical simulations or lab experiments. To overcome this challenge, we propose a pretrain-finetune framework for the crystal property prediction task named CrysDiff based on diffusion models. In the pre-training phase, CrysDiff learns the latent marginal distribution of crystal structures via the reconstruction task. Subsequently, CrysDiff can be fine-tuned under the guidance of the new sparse labeled data, fitting the conditional distribution of the target property given the crystal structures. To better model the crystal geometry, CrysDiff notably captures the full symmetric properties of the crystals, including the invariance of reflection, rotation, and periodic translation. Extensive experiments demonstrate that CrysDiff can significantly improve the performance of the downstream crystal property prediction task on multiple target properties, outperforming all the SOTA pre-training models for crystals with good margins on the popular JARVIS-DFT dataset.
更多查看译文
关键词
DMKM: Applications
AI 理解论文
溯源树
样例
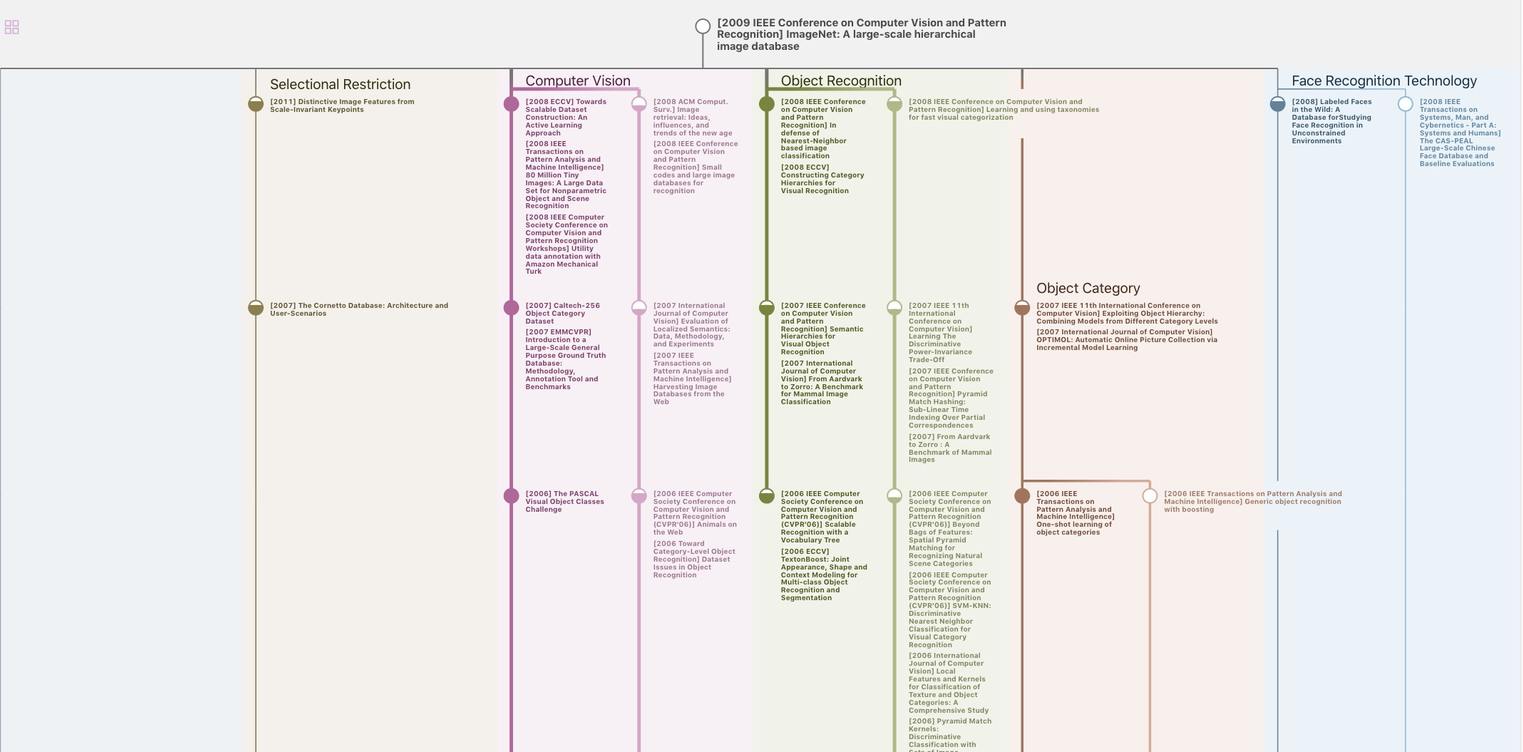
生成溯源树,研究论文发展脉络
Chat Paper
正在生成论文摘要