Responsible Bandit Learning via Privacy-Protected Mean-Volatility Utility
AAAI 2024(2024)
摘要
For ensuring the safety of users by protecting the privacy, the traditional privacy-preserving bandit algorithm aiming to maximize the mean reward has been widely studied in scenarios such as online ride-hailing, advertising recommendations, and personalized healthcare. However, classical bandit learning is irresponsible in such practical applications as they fail to account for risks in online decision-making and ignore external system information. This paper firstly proposes privacy protected mean-volatility utility as the objective of bandit learning and proves its responsibility, because it aims at achieving the maximum probability of utility by considering the risk. Theoretically, our proposed responsible bandit learning is expected to achieve the fastest convergence rate among current bandit algorithms and generates more statistical power than classical normality-based test. Finally, simulation studies provide supporting evidence for the theoretical results and demonstrate stronger performance when using stricter privacy budgets.
更多查看译文
关键词
General
AI 理解论文
溯源树
样例
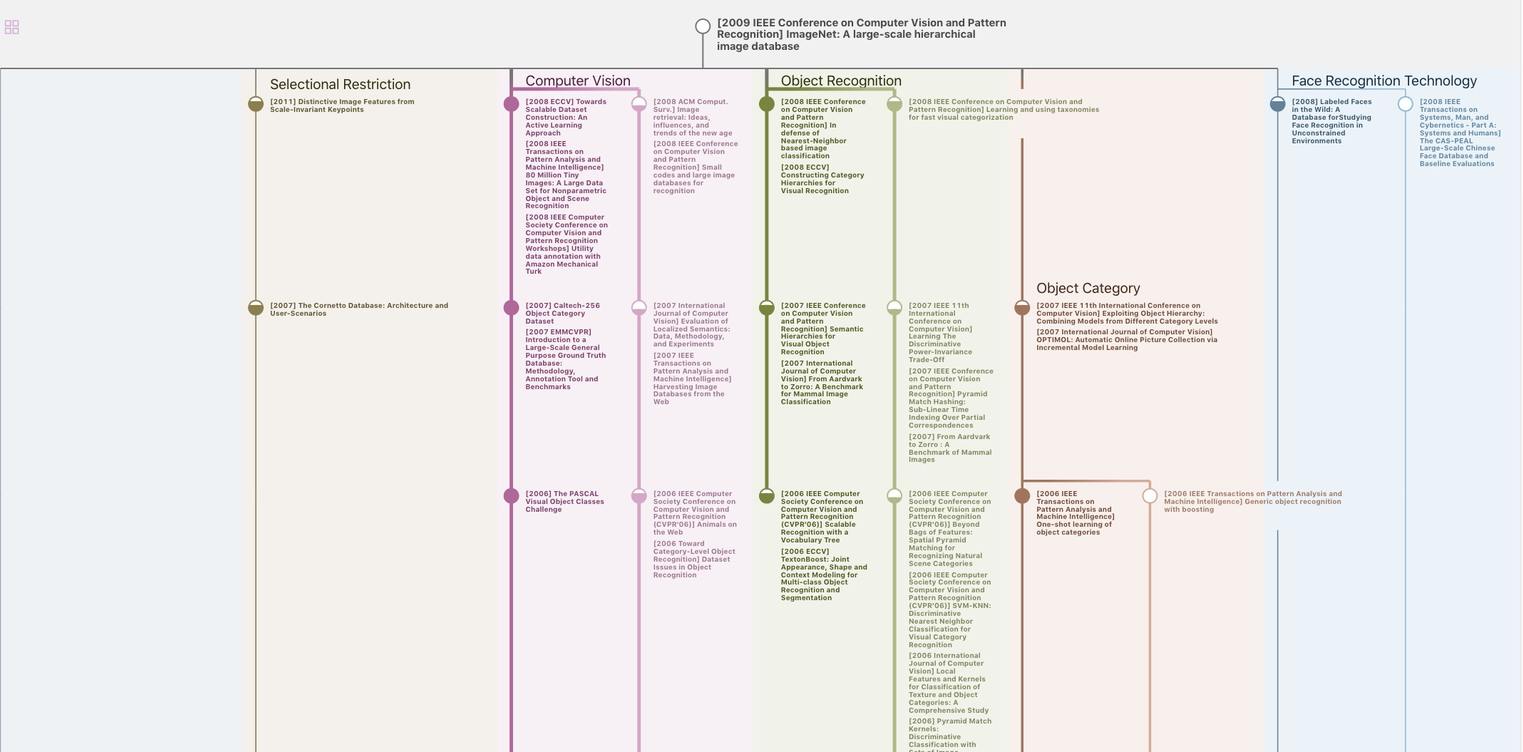
生成溯源树,研究论文发展脉络
Chat Paper
正在生成论文摘要