Limited-Supervised Multi-Label Learning with Dependency Noise
AAAI 2024(2024)
摘要
Limited-supervised multi-label learning (LML) leverages weak or noisy supervision for multi-label classification model training over data with label noise, which contain missing labels and/or redundant labels. Existing studies usually solve LML problems by assuming that label noise is independent of the input features and class labels, while ignoring the fact that noisy labels may depend on the input features (instance-dependent) and the classes (label-dependent) in many real-world applications. In this paper, we propose limited-supervised Multi-label Learning with Dependency Noise (MLDN) to simultaneously identify the instance-dependent and label-dependent label noise by factorizing the noise matrix as the outputs of a mapping from the feature and label representations. Meanwhile, we regularize the problem with the manifold constraint on noise matrix to preserve local relationships and uncover the manifold structure. Theoretically, we bound noise recover error for the resulting problem. We solve the problem by using a first-order scheme based on proximal operator, and the convergence rate of it is at least sub-linear. Extensive experiments conducted on various datasets demonstrate the superiority of our proposed method.
更多查看译文
关键词
ML: Multi-class/Multi-label Learning & Extreme Classification,ML: Multi-instance/Multi-view Learning,ML: Optimization
AI 理解论文
溯源树
样例
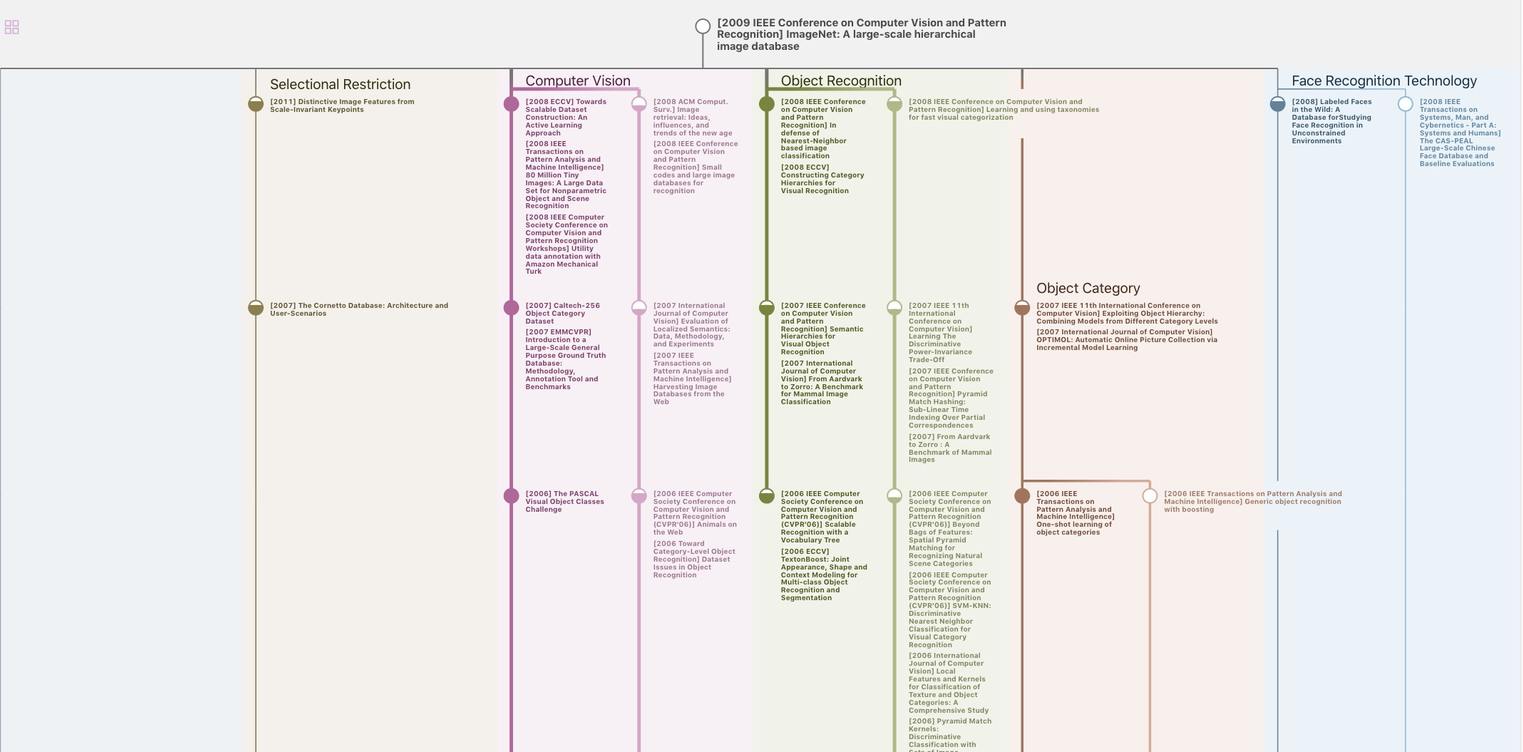
生成溯源树,研究论文发展脉络
Chat Paper
正在生成论文摘要