EG-NAS: Neural Architecture Search with Fast Evolutionary Exploration
AAAI 2024(2024)
摘要
Differentiable Architecture Search (DARTS) has achieved a rapid search for excellent architectures by optimizing architecture parameters through gradient descent. However, this efficiency comes with a significant challenge: the risk of premature convergence to local optima, resulting in subpar performance that falls short of expectations. To address this issue, we propose a novel and effective method called Evolutionary Gradient-Based Neural Architecture Search (EG-NAS). Our approach combines the strengths of both gradient descent and evolutionary strategy, allowing for the exploration of various optimization directions during the architecture search process. To begin with, we continue to employ gradient descent for updating network parameters to ensure efficiency. Subsequently, to mitigate the risk of premature convergence, we introduce an evolutionary strategy with global search capabilities to optimize the architecture parameters. By leveraging the best of both worlds, our method strikes a balance between efficient exploration and exploitation of the search space. Moreover, we have redefined the fitness function to not only consider accuracy but also account for individual similarity. This inclusion enhances the diversity and accuracy of the optimized directions identified by the evolutionary strategy. Extensive experiments on various datasets and search spaces demonstrate that EG-NAS achieves highly competitive performance at significantly low search costs compared to state-of-the-art methods. The code is available at https://github.com/caicaicheng/EG-NAS.
更多查看译文
关键词
ML: Evolutionary Learning,CV: Learning & Optimization for CV
AI 理解论文
溯源树
样例
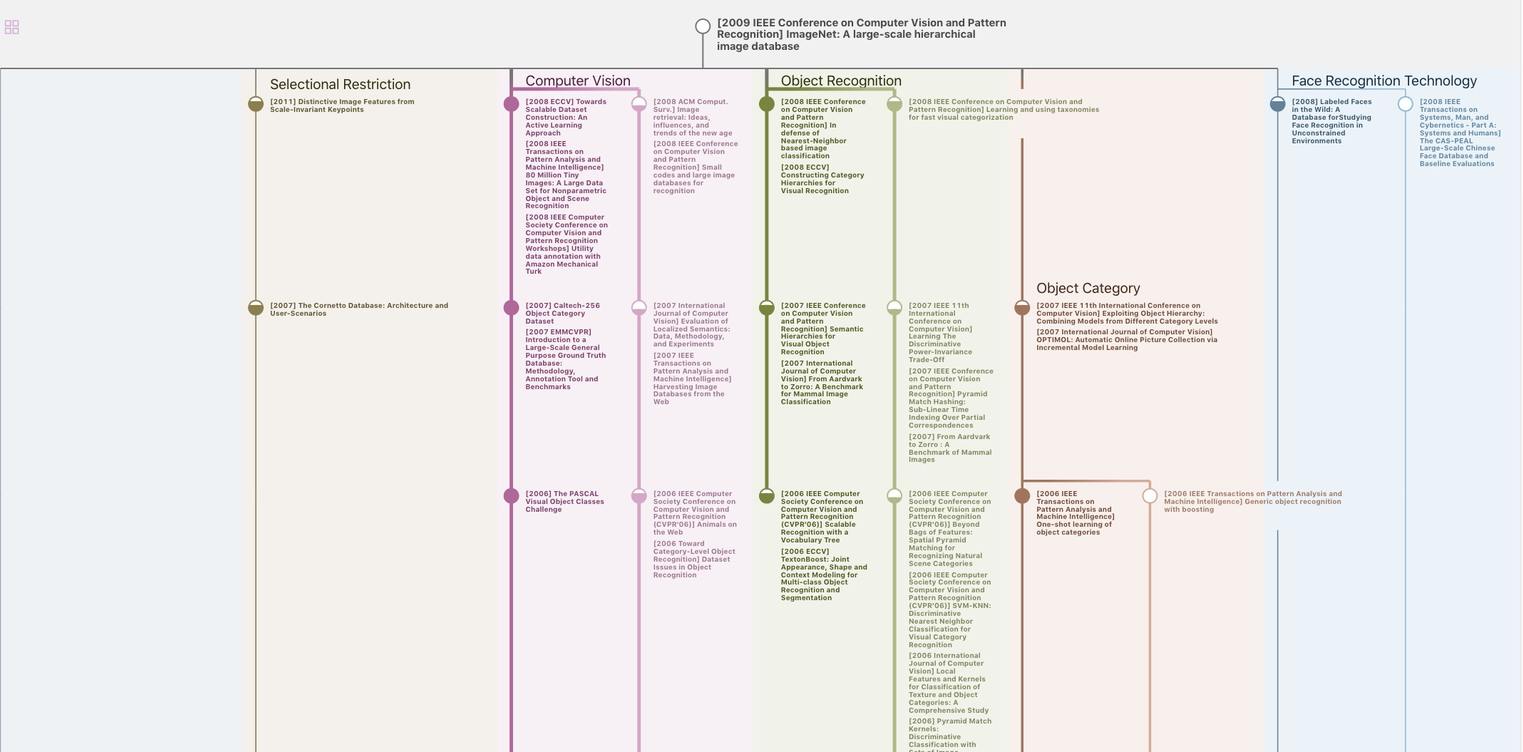
生成溯源树,研究论文发展脉络
Chat Paper
正在生成论文摘要