Predictive Maintenance Framework for Fault Detection in Remote Terminal Units
Forecasting(2024)
摘要
The scheduled maintenance of industrial equipment is usually performed with a low frequency, as it usually leads to unpredicted downtime in business operations. Nevertheless, this confers a risk of failure in individual modules of the equipment, which may diminish its performance or even lead to its breakdown, rendering it non-operational. Lately, predictive maintenance methods have been considered for industrial systems, such as power generation stations, as a proactive measure for preventing failures. Such methods use data gathered from industrial equipment and Machine Learning (ML) algorithms to identify data patterns that indicate anomalies and may lead to potential failures. However, industrial equipment exhibits specific behavior and interactions that originate from its configuration from the manufacturer and the system that is installed, which constitutes a great challenge for the effectiveness of ML model maintenance and failure predictions. In this article, we propose a novel method for tackling this challenge based on the development of a digital twin for industrial equipment known as a Remote Terminal Unit (RTU). RTUs are used in electrical systems to provide the remote monitoring and control of critical equipment, such as power generators. The method is applied in an RTU that is connected to a real power generator within a Public Power Corporation (PPC) facility, where operational anomalies are forecasted based on measurements of its processing power, operating temperature, voltage, and storage memory.
更多查看译文
关键词
predictive maintenance,remote terminal unit,time-series forecasting,anomaly detection
AI 理解论文
溯源树
样例
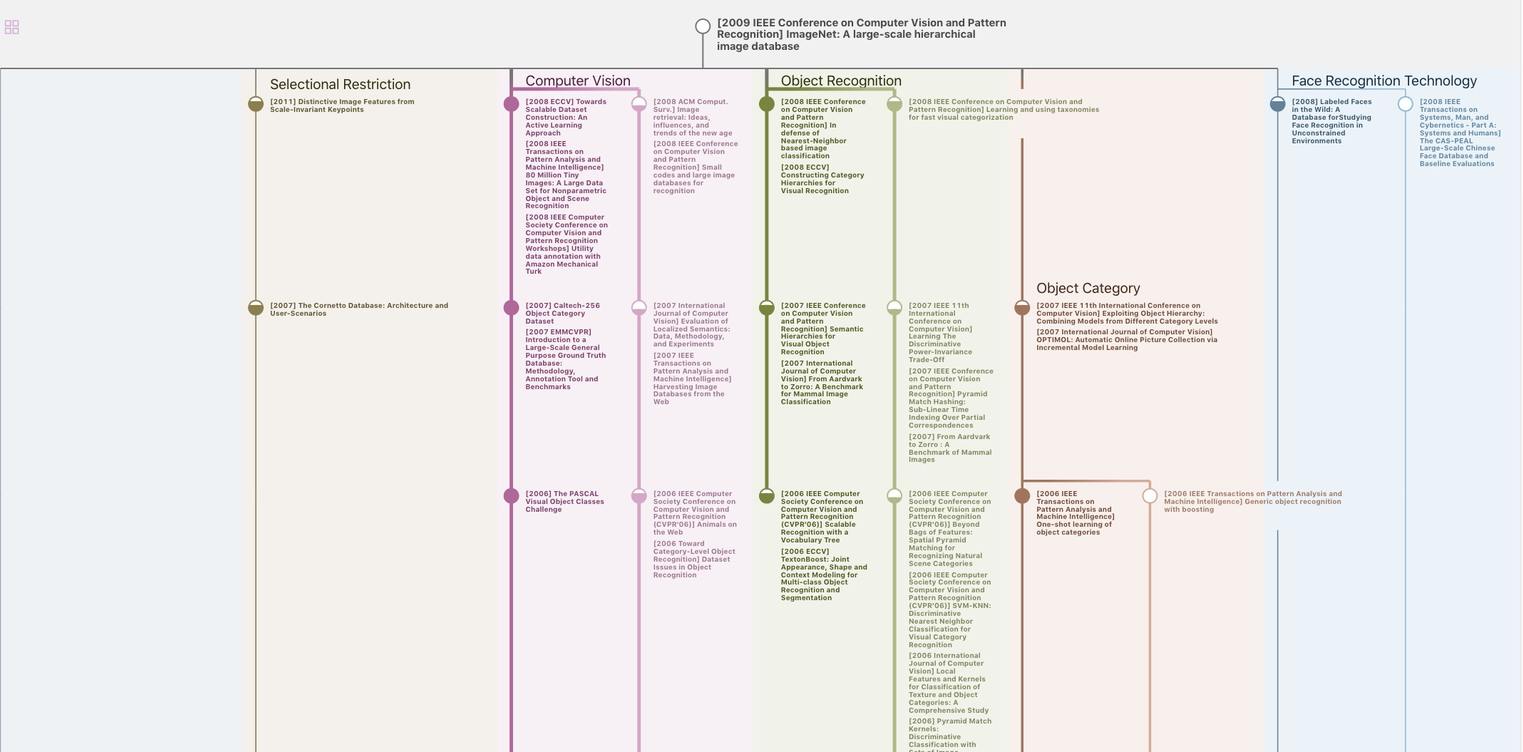
生成溯源树,研究论文发展脉络
Chat Paper
正在生成论文摘要